There is an emerging body of research showing excessive sitting time to be associated with various negative health consequences, including cardiometabolic and cardiovascular disease, some cancers, and premature mortality (1–3). The past decades have shown a reduction in physically demanding occupations and an increase in sedentary occupations, in particular in Western countries (4, 5). Even though the association of sitting with negative health consequences appears to be less consistent for occupational sitting than for leisure-time or total sitting (6–8), excessive sitting seems to be an emerging occupational health hazard with research increasingly being devoted to understanding the health effects of prolonged sitting at work. Office workers, who generally spend most of their workday sitting (9), are a particularly important interest group in this line of research.
Studies on the health consequences of occupational sitting have largely relied on self-reported measurements of sitting time based on questionnaires. However, such self-reports may result in imprecise and biased estimates of sitting (10–14). Self-reported total sitting time, work and leisure combined, has been shown to be underestimated by 15−37% (15, 16), while underestimation ranged from 1.5−43% among workers self-reporting their occupational sitting time (17, 18). Bias in self-reported sitting time may vary depending on the worker’s body mass index (BMI) (17, 19, 20), gender (17, 19, 21), age (19, 22), musculoskeletal pain (17, 21), psychosocial work demands (17), and education level (22). Bias in self-reported sitting time will result in flawed relations with health outcomes, while non-differential misclassification (eg, as a result of random variation in self-reported sitting time) can result in attenuation of associations with health outcomes (23). Such flawed and attenuated associations can affect guidelines and recommendations of sitting exposure across different occupational groups.
As an alternative to self-reports, objective measurements such as accelerometer- or inclinometer-based methods offer accurate information on sitting time (24). However, these methods demand more resources than questionnaires, which make them hard to apply in large-scale epidemiological studies. Therefore, despite the bias and possible imprecision of questionnaire-based data on sitting time and the availability of alternative measurement methods, self-reported measures of sitting are still commonly used in large cohorts. In order to address bias in self-reported sitting in existing data and future studies, it is of interest to determine the extent to which self-reported information on sitting can be improved.
Bias in self-reported sitting may be addressed by developing a calibration model associating self-reports with “true” sitting measured using more valid methods (25). Some previous studies have developed such calibration models for self-reported sitting time at work (26, 27), using predictors that were also obtained by self-reports. Other studies have developed models to improve self-reported sedentary behavior using objective measurements as an estimate of the ‘truth’ (13, 14). Thus far, only few studies have, however, addressed self-reported sitting at work (17, 19, 21). All these occupational studies were based on blue-collar workers, while models developed for office workers are lacking. Since the occupational setting may influence the correctness of self-reported sitting (17), models for office workers likely differ from those developed for blue-collar populations.
Previous studies developing calibration models have rarely validated their models on a dataset other than that used to build the model. This likely leads to overly optimistic model performance. Validation has been pursued using bootstrapping by which the model can be tested on randomly selected replicates from the original dataset (17, 19), but bootstrapping offers only a suboptimal validation. Studies describing a validation of calibration models for self-reported sitting using a different dataset are currently absent.
In standard research devoted to time-use in physical activities, such as sitting, standing, walking, or vigorous physical activity, these behaviors are addressed one at a time, independent of one another. This includes previous attempts to estimate “true” sitting time from self-reported information (12, 17, 19). However, time-use in different activities inherently add up to a whole, eg, “a full day” or “100%”, and thus form interdependent and constrained parts of a so-called “composition” (28, 29). Compositional data have particular properties, which require a set of analysis techniques, ie, compositional data analysis (CoDA) (30). CoDA accommodates that information is essentially contained in the ratios of time in different behaviors rather than in their individual values, and that data need to be log-transformed to operate on the entire scale of non-CoDA values. In other words, in operating on log-transformed ratios, CoDA effectively addresses that times spent in different activities are inherently dependent and can be presented only with values of 0−100%. Both of these properties invalidate standard analysis of non-transformed compositional data. Using standard procedures anyway may lead to bizarre results, such as confidence intervals (CI) >100% time and erroneous conclusions (31). After a log-transformation according to CoDA, data can, however, be analyzed using standard statistical methods. CoDA has received considerable attention in some research fields (30), but has only recently been implemented in studies of physical activities and sedentariness (29, 31–34).
The aim of the present study is to develop calibration models, within a CoDA framework, estimating “true” sitting time among office workers, as objectively measured by accelerometry, from self-reported sitting and additional self-reported information. As a secondary objective, we aim to determine the extent to which these models are valid when used on new data from the same group of workers.
Methods
Design and participants
Data were used from a study on the effect of relocating workers from either cell or open plan offices to activity-based offices. This study, which has been described in more detail elsewhere (35), was conducted among office workers of a large Swedish governmental agency, ie, the Swedish Transport Administration, using a controlled trial design including intervention and control groups. Data were collected between May 2015 and January 2017 at three time points: prior to the relocation (baseline), three months after relocation (T1), and 12 months after relocation (T2). In the present study, we built a calibration model using data from the baseline measurements and then validated this model using data from the same group of workers at T1 and T2.
Workers were recruited from five offices located in different parts of Sweden. A relocation to activity-based offices was implemented in four of these offices (intervention group) but not in the fifth (control group). Potential participants were provided with a web-based questionnaire that could be opened through a web-link. In this questionnaire, participants could tick a box to show their interest in participating in objective measurements of physical activities, including sitting. Workers who were on sick or maternal leave, who were not moving to the new activity-based office, and/or who knew in advance that they would change jobs or retire during the course of the study were excluded. The Regional Ethical Review Board in Uppsala, Sweden approved this study (registration no. 2015/118), and all participants provided written informed consent prior to participation.
Measurements
During baseline and follow-up measurements, participants took part in a protocol of objective measurements of time spent in physical activities and sitting and were asked to complete a web-based questionnaire on sitting time and other variables that could, in theory, predict sitting behavior (referred to in the section “Self-reported sitting time and other predictors”). The questionnaire was answered either shortly before or after taking part in the objective measurement protocol. The participant flow chart is shown in figure 1. Of 901 workers invited, 493 returned their questionnaire at baseline, and 117 agreed to take part in the protocol of objective measurements. A total of 99 workers who provided valid data on both objectively and self-reported sitting time at baseline were included in the present study; 18 workers did not provide sufficient data from both assessment methods and were therefore excluded. Of the 99 workers with complete data at baseline, 74 and 67 also provided sitting time data from T1 and T2, respectively, and were included in the validation of the calibration models developed using baseline data.
Objective measurements of sitting time
Physical activities, including sitting, were objectively recorded from each participant continuously for a period of 5–8 days, using a tri-axial accelerometer (Actigraph GT3X+) secured to the thigh, according to a standardized protocol (36, 37). Data were sampled at 30 Hz and processed off-line using customized Acti4 software (National Research Centre for the Working Environment, Copenhagen, Denmark), resulting in a time-series of uninterrupted periods of sitting and other activities (including standing and walking) with high sensitivity and specificity (37, 38). These data were further processed using Spike2 software (version 7.03, Cambridge Electronic Design, Cambridge, UK), excluding periods of non-work and non-wear time as well as recordings showing technical errors. Here, non-workdays and non-worktime were identified based on information from a diary kept by the worker. Non-wear time was defined as periods of >4 hours without any change in body position. Recording periods showing technical errors were identified by visual inspection and an automatic algorithm.
For each worker, the average daily sitting time (minutes/day) across valid measurement days was calculated for further analysis. Also, total daily time spent at work in non-sitting activities (ie, standing, walking, and other physical activities) were estimated for each worker.
Self-reported sitting time and other predictors
The International Physical Activity Questionnaire (IPAQ) (39) was used, asking for sitting time in hours and minutes during the preceding seven days by the question (translated from Swedish): “During the past 7 days, how much time did you spend sitting during a typical workday?”. From these reports, self-reported sitting time (minutes/day) were estimated and used for further analysis. Daily times spent in non-sitting activities (ie, standing and walking) were self-reported using similar questions.
A number of additional factors that could likely influence workers’ ability to assess sitting time (18) were added as candidate predictors to the calibration model based only on self-reported sitting. We obtained age (in years – calculated from the date of birth), gender (male/female) and smoking (yes/no). BMI was estimated by dividing self-reported body weight (in kg) by the squared self-reported stature (in cm). Moreover, participants were asked about their highest level of education with response options primary, secondary, vocational and university. Post-hoc, we merged the first three answer categories to obtain sufficient numbers of participants in the response categories.
The participant’s worksite was noted at study recruitment. Type of office was self-reported with alternatives cell office, shared office, flexible office, or open-plan office. For further analyses, two categories were formed: cell office and open-plan office (merging all non-cell office types). Being in a managing position (yes/no) and seniority in the work task (in years, answering the question “for how many years have you worked with your current work tasks”) were also obtained from the questionnaire. The questionnaires addressed the time spent using a screen and the extent to which the physical load at work was perceived to be varying (both with six outcome categories dichotomized into: not/never versus a little or more). Psychosocial work demands were reported using a two-item short form of the Copenhagen Psychosocial Questionnaire (COPSOQ), with outcomes converted into a continuous scale from 0 (no demands at all) to 8 (highest demands possible) (40). Social support at work was measured using three items of the long form version of the COPSOQ, and according to the COPSOQ manual (40). Answers were converted to a continuous scale ranging from 0 (no support at all) to 10 (highest support possible).
General health was obtained using a single-item from the Swedish version of the SF-36 health survey (41) with five response categories that were combined into a dichotomous answer, ie, excellent/very good/good versus reasonable/bad. Wellbeing at work was assessed using the question “Here are some faces that express different degrees of well-being. Which of the faces expresses best how you have experienced your well-being at work over the past four weeks?”. The 7-point scale uses seven emoticon images with expressions ranging from very happy/satisfied to very sad/dissatisfied. This way of using emoticons was modified from a children’s pain scale (42), and has been used in the context of measuring well-being at work before (43). Scales were reversed so that higher values indicate higher wellbeing. Musculoskeletal symptoms were obtained using items from the Nordic questionnaire (44), asking for symptoms in the past four weeks. We only focused on symptoms in the upper body (yes/no; yes if pain or discomfort was reported in any of the areas neck, shoulder, arm and/or hand) and back (yes/no; yes if pain or discomfort was reported in any of the areas upper and/or lower back).
Data analysis
All analyses were conducted using SPSS, version 22 (IBM, Armonk, NY, USA). Because time-use data, such as sitting time expressed as parts of the day, are compositional in nature, we used a CoDA approach (45). This method, which has been explained in more detail in the background section, is based on the notion that a log-ratio transformation of compositional variables will result in data expressed in an Euclidian space, which can then be analyzed using conventional statistical methods developed for non-constrained, normally distributed data (29). Thus, we expressed sitting, both self-reported and objectively measured, in terms of an isometric log-ratio (ILR), as used in several other studies applying CoDA to data on time spent in physical activities (18, 29, 32, 34, 46, 47). The ILR expresses sitting in terms of the ratio of the percentages of time spent sitting to time spent non-sitting, ie, %sit/%non-sit, log-transformed and multiplied by 1/√2, ie, (noting that %non-sit equals 100 - %sit):
with z being the ILR and x being %sit. In this binary case (sitting versus non-sitting) the ILR can, essentially, be interpreted as the log-transformed odds of being seated, multiplied by 1/√2. Since this transformation cannot be performed if x=0 or x=100, workers reporting to sit for the entire working day, ie, spending 100% time in sitting, were assigned 2 minutes of non-sitting in a 480-minute working day, corresponding to 0.42% of the day. This replacement procedure is similar to that used in many other CoDA studies to handle essential zeroes (30).
Using ILR, we built a calibration model, using data from baseline measurements. First, a simple calibration model was developed using linear regression for objectively measured (“true”) sitting as the dependent variable and self-reported sitting as the independent variable. A full calibration model was built by adding the aforementioned candidate predictors to the simple model in a forward stepwise regression procedure. Variables contributing to the model, at a statistical significance level P<0.1, were retained. Prior to building this multivariate model, Spearman correlations between all pairs of variables were estimated, seeking correlation coefficients >0.70. However, no correlations this strong were found (supplementary table S1 www.sjweh.fi/show_abstract.php?abstract_id=3827); hence all candidate variables were allowed into the multivariate stepwise analysis. In both the simple and the full model, regression coefficients (beta) with 95% CI were estimated. As a measure of estimation error or model performance, root-mean-square (RMS) differences between estimated and objectively measured “true” sitting were calculated for uncalibrated self-reports as well as for estimates obtained by the simple and the full calibration models in the ILR space. Also, we calculated 95% CI on estimates of individual values from the simple model (48).
For the ease of application, estimates obtained by the simple model were back-transformed from the ILR space to a standard scale, using the inverse of equation (1), ie,:
Back-transformed results of the simple regression were compared to results obtained by a regression of objectively measured “true” sitting on self-reported sitting developed without using CoDA, in order to examine effects of using CoDA.
The two calibration models (ie, simple and full) developed on baseline data were validated using follow-up data from T1 and T2. Thus, at both T1 and T2, we processed self-reported sitting and the additional predictors through the regression equations obtained at baseline, to get calibrated sitting. Estimation performance was then assessed using the same metrics as at baseline, ie, the RMS errors when only using self-reported sitting, as well as calibrated from the simple and full model estimate.
Results
Descriptive statistics of the study sample are shown in table 1. Participants were on average 47.1 [standard deviation (SD) 9.1] years of age and 50% were female. Objective measurements were available from, on average 4.6 (SD 0.9) workdays per worker, with in total 481.3 (SD 60.9) minutes/day of measurement (table 2). Average self-reported sitting time was 363.2 (SD 96.1) minutes/day, and objectively measured sitting was 353.2 (SD 87.1) minutes/day. Expressed in percentage, sitting occupied 71.2 (SD 20.6)% and 69.9 (SD 14.7)% of the workday according to self-reported and objectively measured data, respectively. Expressed in ILR, sitting averaged 0.81 (SD 0.90) and 0.66 (SD 0.51) for self-reported and objectively measured data, respectively. Frequency distributions of sitting at baseline are shown in figure 2. Averages and standard deviations were comparable at follow-up T1 and T2. Notably, the relocation to activity-based offices had only limited effects on the workers’ sitting time (35).
Table 1
Descriptive statistics of the study sample (N=99). [N=Number of participants; SD=standard deviation.]
Table 2
Number of participants for which sitting time was available, at baseline, T1, and T2; objective measurements in total; and sitting time according to both self-report and objective measurements, expressed in min/day as well as isometric log ratio] (ILR) (group mean values with standard deviation between participants in brackets). [SD=standard deviation.]
Baseline N=99 | T1 a N=74 | T2 b N=67 | |
---|---|---|---|
|
|
|
|
Mean (SD) | Mean (SD) | Mean (SD) | |
Objective measurements, total | |||
Days per participant | 4.6 (0.9) | 4.4 (0.8) | 4.6 (0.5) |
Minutes/day and participant | 481.3 (60.9) | 477.5 (41.8) | 477.2 (47.8) |
Sitting time | |||
Self-reported (minutes/day) | 363.2 (96.1) | 374.4 (96.1) | 378.6 (81.4) |
Self-reported (ILR) | 0.81 (0.90) | 1.12 (1.09) | 0.87 (0.85) |
Objectively measured | |||
Minutes/day | 353.2 (87.1) | 342.1 (82.1) | 345.5 (74.0) |
ILR | 0.66 (0.51) | 0.75 (0.55) | 0.68 (0.57) |
Figure 2
Distribution at baseline of self-reported (left panels) and objectively measured (right panels) sitting, expressed in min/day (upper panels) and by isometric log-ratios (ILR; lower panels); N=99.
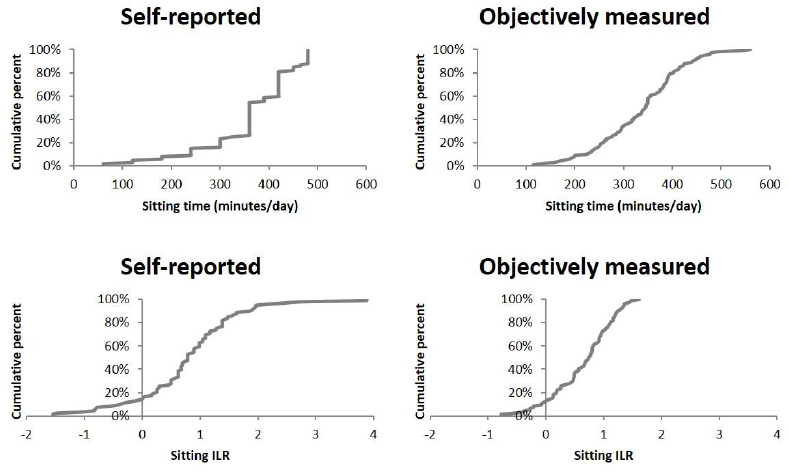
Table 3 shows results of the regression models obtained using the CoDA approach. The RMS error when estimating sitting using uncalibrated self-reports was 0.767; the simple and full calibration models succeeded in reducing this estimation error to 0.422 (55%) and further to 0.398 (52%), respectively. Regression models including variables that were not allowed into the full model are shown in supplementary table S2, (www.sjweh.fi/show_abstract.php?abstract_id=3827).
Table 3
Calibration models in the CoDA (compositional data analysis) space predicting objectively measured (‘true’) sitting time. Results from a simple model only incorporating self-reported sitting time, and a full model which also incorporates body mass index, office type and gender. The table shows regression coefficients (beta) with 95% confidence intervals (CI), and the root-mean-squared (RMS) error of estimates obtained by the model. All values refer to parameters in the compositional data analysis (CoDA) space. [ILR=isometric log ratio.]
Baseline model | Validation (T1a) | Validation (T2 b) | ||||||
---|---|---|---|---|---|---|---|---|
|
|
|
||||||
Beta | 95% CI | RMS | RMS (%) | RMS | RMS (%) | RMS | RMS (%) | |
Uncalibrated | 0.767 | 100 | 0.938 | 100 | 0.829 | 100 | ||
Simple model | ||||||||
Intercept | 0.41 | 0.30–0.52 | 0.422 | 55 | 0.419 | 45 | 0.512 | 62 |
Self-reported sitting (ILR) | 0.31 | 0.22–0.39 | ||||||
Full model | ||||||||
Intercept | -0.19 | -0.65–0.30 | 0.398 | 52 | 0.419 | 45 | 0.516 | 62 |
Self-reported sitting (ILR) | 0.34 | 0.24–0.43 | ||||||
Body mass index | 0.02 | -0.00–0.04 | ||||||
Cell office | Ref. | |||||||
Open plan office | 0.18 | 0.01–0.34 | ||||||
Females | Ref. | |||||||
Males | 0.14 | -0.02–0.30 |
As an example of how to perform a calibration using our simple model, consider a worker self-reporting to sit corresponding to 52% of the time. As a first step, this self-reported sitting time is transformed to an ILR using equation 1, ie, 1/√2×ln[52/(100-52)]=0.057. According to the simple calibration model (table 3), the estimated “true” ILR will be (0.057×0.31)+0.41=0.43. Back-transforming this ILR into standard space, using equation 2, results in the estimate of “true” sitting being 65% of the time. Figure 3 illustrates this calibration procedure, leading from self-reported time in standard space (x-axis) via regression in CoDA back to an estimated value of “true” sitting in non-CoDA space (y-axis), for self-reported values from 1−99% time, including the 95% CI of estimates of a single worker’s “true” sitting obtained by the calibration. The regression curve deviates considerably from the line of identity, illustrating that self-reported sitting was extensively biased. On average, workers underestimated their sitting if they actually sat for less than about 70% time, while they overestimated sitting if actually sitting more than that. As an example, if a worker reported to sit for 85% of his/her time, the simple calibration model illustrated in figure 3 estimates that he/she was actually sitting for only 75% time, with a 95% likelihood of objectively measured “true” sitting being 48−91%. The full model performed slightly better than the simple model (table 3). Since the full calibration model includes more than one predictor, it cannot be visualized in a two-dimensional plot.
Figure 3
Black curves illustrate estimated “true” sitting time according to the simple CoDA (compositional data analysis) calibration model, plotted against self-reported sitting time. Thick lines show the regression curve, while thin lines mark the 95% confidence interval of an estimate of “true” sitting for one single individual. Estimates were obtained under CoDA and back-transformed to the standard, non-CoDA space. Original, uncalibrated data values for each participant (N=99) are illustrated by squares, and the linear regression neglecting CoDA (with 95% confidence interval for single individuals) is illustrated by grey lines. The dashed line shows the line of identity.
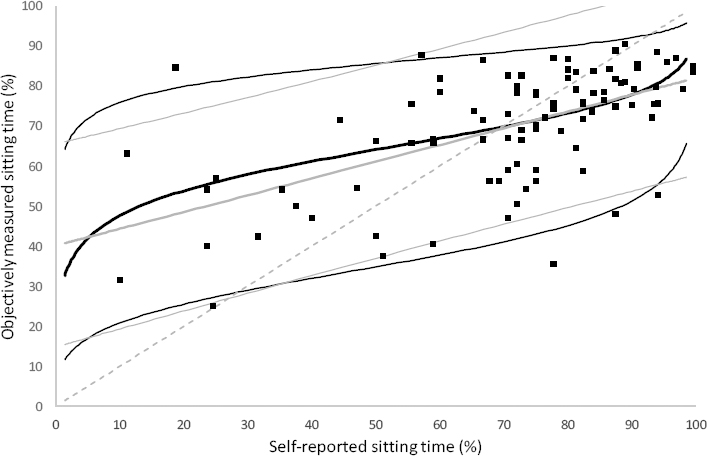
As a sensitivity analysis, the full calibration model was remade using centered, continuous prediction variables (ie, expressing values from individual participants as the difference between the observation and the group mean), which could, in theory, lead to more stable model parameter estimates. While this model had, as expected, another intercept than the original full model, ie, 0.25 (95% CI 0.09–0.40) in the model with variables centered, as compared to -0.18 (95% CI -0.65–0.30) in the original model, regression coefficients of all predictor variables were similar. Thus, centering did not influence the general result of the calibration.
When applying the regression equation from the simple model on self-reported sitting at the first-follow up (T1), the RMS error was reduced to an even larger extent (down to 45% of the error in uncalibrated self-reports) than in the baseline data used for developing the model, mainly because the error in uncalibrated self-reports was substantially larger at T1 than at baseline (table 3). The full model performed no better than the simple model at T1 (45% of the uncalibrated self-report error). At T2, the simple model reduced the RMS error to 62%, ie, the model was notably less successful than at baseline, while the full model again performed no better than the simple model (62% of uncalibrated RMS).
Discussion
In this study we developed calibration models estimating “true” sitting time among office workers, as objectively measured by accelerometry, from self-reported sitting time. We also determined the extent to which these models are valid even when used on new data from the same group of workers. We developed a simple model using self-reported sitting as the only predictor of objectively measured sitting, and a full model that included additional predictors. The models reduced the RMS error in estimates of “true” sitting to 55% (simple model) and 52% (full model) of that associated with just using the uncalibrated self-reported sitting. This reduction is comparable to what has been found in a similar study among blue-collar workers, but based on another phrasing of the question asked in the self-report (19). Our finding that “true” sitting is underestimated by self-reports when sitting occurs less, while it is overestimated when sitting occurs more corroborates observations in previous studies (14, 20). Our full calibration model included variables that have previously been suggested to influence bias in self-reported sitting time, such as BMI (17, 19) and gender (17, 19, 21). However, other contributing variables that have been identified in earlier research, such as age (19, 22), musculoskeletal pain (17, 21), psychosocial work demands (17) and education level (22) did not make it into our full model. Adding to findings in earlier research, office type appeared to be a significant predictor in our full model. Previous modelling studies of sitting have, however, been based on samples from other populations, such as blue-collar workers and the general population.
Validation of our calibration models indicated that performance would change if they were used in a new dataset, albeit on the same sample of workers. Although our validation does not provide information on how our models would perform in an entirely new sample of office workers, it provides insight into how our models could behave in the realistic situation of following a particular group of participants over a period of time. While some decrease in performance (‘optimism’ (49, 50)) could be expected, given that the performance at baseline capitalizes on the fact that the model is developed to fit the exact dataset on which performance is then evaluated, we found that at the first follow-up, the models performed even better than at baseline, in terms of the relative reduction in RMS error. However, the absolute RMS errors were very similar at baseline and follow-up. Performance decreased between the first and second follow-up, to the extent that performance was then poorer than at baseline, in terms of both absolute and relative estimation error. To our knowledge, we are the first to report a development in model performance over time. Moreover, at both follow-ups, the full model performed no better in estimating sitting than the simple model based only on self-reported sitting. We propose that this remarkable result is caused by the full model capitalizing even more on chance at baseline than the simple model, and thus being even more sensitive to new datasets deviating in structure from the one on which the model was developed. In any case, the result issues a warning against overfitting models at baseline, and believing that larger models will perform better than parsimonious models when applied to new datasets.
Even our simple calibration model reduced the error in sitting estimates substantially and appears to be useful for populations of office workers with similar tasks and working conditions as our population. Therefore, we believe our models to have the potential to be used in retrospective calibration of self-reported sitting data in published studies on comparable populations of office workers, eg, of associations between sitting and different health outcomes. The calibration models, and in particular the simple model which appears more robust, also have the potential to be used as an efficient method of assessing sitting time in future studies. In particular, large-scale epidemiologic studies might benefit from the likely cost-efficient assessment of sitting by a calibration of self-reported sitting time. In CoDA-space, our simple model expresses that “true” sitting will increase with increasing self-reported sitting, but only at a rate (slope) of 0.31, This essentially means that any linear association between self-reported sitting and some health outcome of interest will be about three times as strong when sitting is calibrated into estimated “true” values than if based on uncalibrated self-reports. In other words: for any outcome, in CoDA-space the “true” effect of increasing sitting by one measurement unit (expressed in the compositional space, ie, as a log-transformed ratio of sitting to non-sitting time) will be three times as large as the alleged effect when sitting is measured by self-reports. Say, for instance, that a study using self-reported sitting has found an increase in sitting by 10% time to be associated with an increase in a given health risk of 1 arbitrary unit. Our simple calibration, illustrated in figure 3, suggests that an increase in 10% self-reported time corresponds to a “true” increase of only 3.2% time. Thus, the 1 unit increase in risk occurs at a “true” increase in sitting which is only one third of that claimed from self-reports. In other words, the risk will be estimated to increase by approximately 3 arbitrary units for every 10% time increase in “true” sitting. This example suggests that current notions regarding health effects of excessive sitting, which are based mainly on self-reported data, may need reconsideration.
Methodological strengths and limitations
A major strength of our study is the use of a valid and reliable method for objectively measuring “true” sitting time. We used accelerometry data processed by the Acti4 software, which has shown good sensitivity and specificity in identifying postures (including sitting) during standardized conditions (37) and during occupational work (38).
Another strength is that we acknowledged the compositional nature of sitting data. As explained in more detail in the background section, CoDA is the preferable approach for analyzing data expressing parts of a whole (28, 29). For comparison, we also did our modelling using a standard (non-CoDA) approach. Figure 3 illustrates some clear differences in the properties of the CoDA and non-CoDA models, in particular at extreme values of sitting time and when it comes to uncertainty in the estimates. For example, consider a worker reporting to sit for 85% time. For this worker the “true” sitting time would be estimated to be 75% by the CoDA as well as the non-CoDA model. However, in the non-CoDA regression the 95% CI on that estimate ranged from 52% time to >100% time, the upper CI limit obviously being absurd. The CoDA approach resulted in a 95% CI of 48−91% time, illustrating that CoDA effectively addresses the truncated nature of compositional data to have values of 0−100 if expressed on a percentage scale.
The act of self-reporting physical activity behaviors may influence future behavior, which could potentially influence objectively measured (“true”) sitting time in case such measurements are conducted after delivering the self-reports (51). In our study protocol, self-reported sitting time was obtained close in time to the objective measurements. Some self-reports were obtained before the objective measurements and others after and we do not expect any notable influence of changed activity awareness on our findings.
A limitation of our study is the relatively low number of participants, which may have reduced the statistical power of our dataset to validly incorporate predictor variables in our full calibration model. Moreover, our participants were Swedish office workers, and results from our study should be extrapolated to other occupational groups and/or geographic areas with due caution. Non-responders to one or both of the two methods for assessing sitting time were not included in the analyses, and this might represent an additional source of bias. In theory, these 18 non-responders could have shown a different ability to provide correct self-reports of sitting time than the workers included in the study, or they could have shown a deviating behavior, eventually influencing the regression models.
Although our calibration models showed reasonable reductions in errors when estimating sitting time from self-reports, comparable to what has been reported in previous research in other populations and with other self-reports (19), the models still left a substantial error unaccounted for. Not all variables that might potentially contribute to improving model performance were available in our dataset. For example, data on specific occupational tasks were lacking, and should be considered in future studies. Even though our full model did not perform much better than our simple model, we cannot exclude that incorporating additional variables could indeed lead to improvements in the performance of our full model.
Concluding remarks
In conclusion, we found that a calibration model in which “true” sitting was estimated from self-reported sitting alone led to substantially more correct estimates than if self-reports were used without correction for bias. Some further improvement in sitting estimation was obtained by adding BMI, office type, and gender as predictors to the model. Using a CoDA approach, which effectively addresses the constrained and inter-dependent nature of data describing time spent in various activities, led to estimates that differed clearly from those obtained using regression on non-CoDA data; in particular at extreme values. Validation of the calibration models indicated that their performance would decrease if they were used in new datasets. Nevertheless, our models can be used post-hoc to improve self-reported sitting data in comparable samples to provide more accurate estimates of occupational sitting. Also, future studies may consider using a similar approach, ie, collecting data on both objective and self-reported data only in a sub-sample, develop a calibration model, and use that model to reduce errors in self-reported sitting even at follow-up, without having to invest in objective measurements again. As such, this approach can be used in the design of future studies assessing occupational sitting, for example in studies investigating the effect of introducing activity-permissive work stations to reduce sitting.