Occupational injuries pose a significant burden on the workforce worldwide. Globally, occupational injuries account for a loss of 3.5 years of healthy life per 1000 workers each year (1). In 2009, the US Bureau of Labor Statistics recorded nearly 3.3 million non-fatal workplace injuries among private industry, over a half of which involved days away from work, job transfer, or job restriction (2). In 2010, the direct workers’ compensation costs of disabling workplace injury were estimated to be US$51 billion (3). In addition to the direct medical costs incurred, injuries at work may result in decline in workers’ long-term earnings (4) and reduction in home activities (5). Leigh (6) estimated that in 2007 the total cost (direct and indirect) of non-fatal occupational injuries in the United States was US$186 billion, as large as the cost of cancer.
Obesity is a widely recognized public health problem. A significant proportion of populations in developed countries in Europe and North America are obese (7), and obesity has also become more common in the developing world (8). Obesity is known to increase the risk of cardiovascular diseases and diabetes (8); lately obesity has been suggested as a risk factor for occupational injury (9–12, 5). Despite such observations, obesity’s contribution to occupational injury risk remains far from clear in epidemiological literature. A review by Pollack & Cheskin (13) with respect to traumatic workplace injury found that many of the estimates for the relationship between obesity and workplace injury in published papers were not statistically significant (14–24). However, the review also noted that many of these studies were limited by small sample sizes, inadequacies in addressing confounding, and/or lack of robustness in statistical methods, hence inconclusive. Moreover, these studies relied on cross-sectional data where it cannot be determined whether exposure to excessive body weight occurred before the incidence of injury.
A limited number of other studies based on longitudinal samples have reported an association between obesity and workplace injury (20, 25, 26). While these study designs were sound and robust, the samples were restricted to certain industry groups. It remains unclear whether the relationship observed in these studies could have been driven by industry- or company-specific factors. Therefore, to better assess the contribution of obesity to occupational injury, we used data from a nationally representative cohort: the National Longitudinal Survey of Youth 1979 (NLSY79). We hypothesized that obesity contributes to workplace injury and tested the hypothesis using logistic regression with generalized estimating equations (GEE) and random-effects logistic regression.
Methods
Data and sample
The NLSY79 sample was designed to represent the entire population of youth aged 14–21 years as of 31 December 1978 and residing in the US as of January 1979 (27). Since our focus was occupational injury in the civilian labor force, we did not discuss or include the military subsample of the NLSY79. In 1978, approximately 75 000 civilian housing units were selected through a multi-stage stratified area probability sampling for a “screening interview”, and interviewers went to these houses to collect basic information such as house members’ age, race, and poverty status (27). Black, Hispanic, and poorer Caucasian American housing units were oversampled to produce statistical efficiency for these subgroups. Based on the screening interviews, those who were aged 14–22 years as of December 1978 in these 75 000 housing units were asked to participate in the first NLSY79 interview.
In 1979, 14 574 people aged 14–22 years were initially designated for interviewing; 12 686 actually completed the first interview (27). The NLSY79 re-interviewed these 12 686 people every year from 1979–1994 and every other year after 1994. During 1988–2000 (except 1991 due to budgetary limitations) the NLSY79 administered a “workplace injury module” for all respondents who had employment history since the previous interview. Our study sample consisted of those NLSY79 cohort participants who completed this module in 1988–2000. The module has been used previously in other studies of occupational injury (28–31).
Overall, the NLSY79 has experienced a low degree of sample attrition/non-response (32). As of 1988, 127 respondents were deceased. Response rates, defined as the percentage of living and eligible respondents who were interviewed, were 91% and 82% in 1988 and 2000, respectively. Our pre-analysis showed that during 1988–2000 non-response was associated with being male and minority status, but not body mass index (BMI). In 1988, of the 10 234 interviews of the civilian NLSY79 sample, 8941 (87%) completed the injury module in that year. The gap was the result of 1293 respondents who lacked employment history since the previous wave. The analysis also indicated that gender, minority status, or being over- or underweight were associated with lack of employment history.
Outcome and exposure
Our outcome variable was injury at work, analyzed as a dichotomous variable, which we obtained from participant responses to the workplace injury module. In this module, the NLSY79 interviewers started with the question: “Since (date of the last interview), have you had an incident at any jobs we previously discussed that resulted in an injury or illness to you?” Then, the survey focused exclusively on the most recent incident, and interviewers went on to collect the characteristics of the injured worker’s job, such as industry and wage. The survey also differentiated injuries from illnesses by asking “Did the incident result in an injury or an illness?” thus we were able to focus on injuries. Note that the wording of the question in 1988 differed slightly from other years; the timeframe used was “over the past 12 months” instead of “since the day of the last interview”.
Our exposure variable was obesity. We measured obesity as BMI based on self-reported height and weight. BMI is defined as weight divided by the square of height (kg/m2). Using the definition published by the US Center of Disease Control (33), we used the following classification: <18.4 (underweight), 18.5–24.9 normal weight, 25.0–29.9 (overweight), and ≥30 kg/m2 (obese). Between 1988–2000, 105 cases reported a BMI <14 or >55 kg/m2, which we considered to be implausible and set as missing. Initial analysis suggested that setting different cut-off points for implausible values did not influence the study results. We used the weight in each previous round to ensure the temporal precedence of the exposure. For example, the weight in 1989 was used to predict injury in 1990. The only exception was 1988; the NLSY79 did not record respondents’ weight in 1987; thus weight in 1986 was used to predict injury in 1988. Accordingly, there were nine measurements for obesity level (’86, ’88, ’89, ’91, ’92, ’93, ’94, ’96, ’98) and nine measurements for injury (’88, ’89, ’90, ’92, ’93, ’94, ’96, ’98, ’00).
Covariates
We included the following covariates as controls or confounders: age, gender, race and ethnicity (indicators for Black and Hispanic, respectively), education (in years), worker’s weekly wage (in log scale), weekly working hours, and industry indicators (1970 Census code). Similar to two previous studies (29, 30), we also constructed two measures from the Dictionary of Occupational Title (DOT) (34) to control for an occupation’s inherent risk of injury. The first constructed measure, environmental hazard, was the sum of six DOT items, each of which ranged from 0–1: (i) extreme cold, (ii) extreme heat, (iii) wet conditions, (iv) extreme noise, (v) other hazards, and (vi) negative atmospheric conditions. The second measure, physical demand, was the sum of four DOT items, each of which ranged between 0–1: (i) climbing and/or balancing, (ii) stooping, kneeling, crouching, and/or crawling, (iii) reaching, handling, fingering, and/or feeling, and (iv) seeing. We calculated the average scores of the two DOT measures for males and females in each occupation and then linked them to the main NLSY79 data using gender and occupation.
Statistical analysis
To estimate the relationship between obesity and occupational injury, we first use logistic regression with GEE.An approach often used to analyze binary outcomes in longitudinal data (35), GEE produces population-averaged estimates (or marginal effects), ie, the changes in the outcome’s population mean given changes in the covariates. This approach has an advantage that the marginal effects can be estimated consistently even if the within-individual correlation is not correctly specified (36). For our analysis, an unstructured correlation was selected to adjust for within-individual correlation because the data were not evenly spaced in time and an autoregressive structure might not have been appropriate.
Since obesity is often viewed as a risk factor attributable to individuals, it is desirable to obtain subject-specific estimates, ie, whether increases in given individuals’ BMI are associated with injury at work. To this end, we supplemented the GEE analysis with random-effects logistic regression (37, 38), which assumes the unobserved differences between individuals are represented by a probability distribution (the random-effects). Note that by nature, the estimates from GEE are different from random-effects (39); one describes population-averaged and the other subject-specific results. And unlike the case of linear models – where estimates from the two approaches tends to be similar – in the case of logistic regression, estimates from a population-average and subject-specific model may diverge because the link function (ie, log) is not a linear transformation (36, 38). All data analyses were performed using Stata 11 (Stata Corporation, College Station, TX, USA); P<0.05 was considered statistically significant.
Results
Sample description
Table 1 presents the number of participants in each round from 1988–2000, the number of respondents who reported having been injured at work since the previous wave, and the distribution of weight status in each previous wave. The number of injuries dropped from 752 in 1988 to 447 in 2000; the corresponding weighted percentages were 8.7% and 6.9%, respectively. Overall, the NSLY79 respondents were gaining weight steadily, from 35.2% being obese or overweight in 1987 to 59.7% in 1998.
Table 1
Number of participants, injuries, and distribution of weight status in the National Longitudinal Survey of Youth 1979 (NLSY79) in 1988–2000.
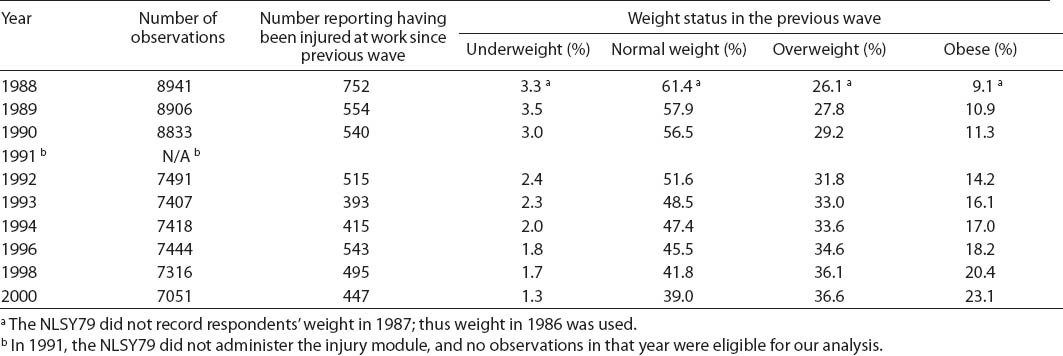
Table 2 presents other characteristics of those who completed the injury module in 1988. On average, respondents were 27.5 years old, had completed 13.2 years of education, and had spent 40.6 hours at work per week. We further broke down the incidence of injury by industry. Table 3 indicates that while injuries occurred in all industries (716 cases reported in total), the majority clustered in manufacturing (189 cases) and wholesale and retail (170 cases).
Table 2
Characteristics of National Longitudinal Survey of Youth 1979 participants who completed the workplace injury module in 1988. [SD=standard deviation; N=8941 a].
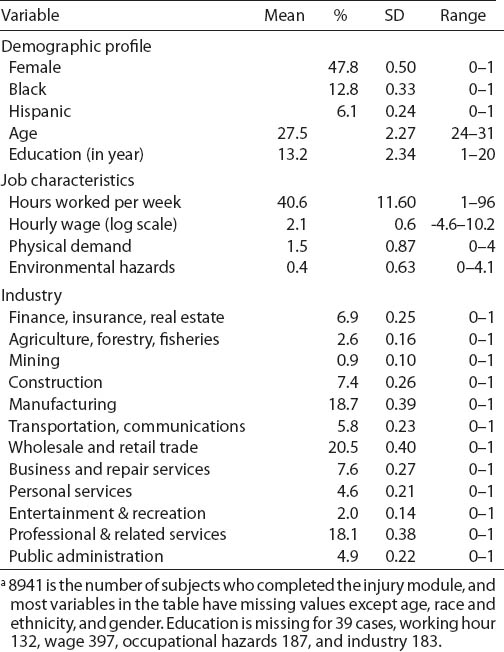
Table 3
Number of respondents reporting workplace injury in 1988 by industry, National Longitudinal Survey of Youth 1979.
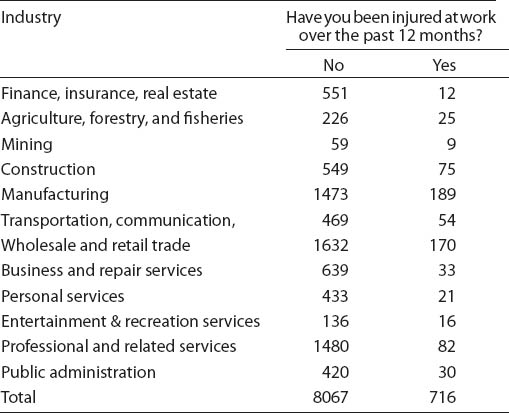
Table 4 presents the GEE and random-effects estimates of the logistic regression controlling for other covariates. The GEE analysis shows that obesity was associated with a 25% higher odds of workplace injury [odds ratio (OR) 1.25, 95% confidence interval (95% CI) 1.12–1.39; P<0.001]. Overweight was marginally significant (OR 1.08, 95% CI 1.00–1.18; P=0.06) but underweight status was not statistically significant. While both occupational hazard indicators were associated with increased risk of injury, females and minorities had lower odds of injury at work. Workers in all other industries had higher odds of occupational injury than the referent, ie, the finance, insurance, and real estate industry group. The results of random-effects regression were similar to those of GEE. Obese workers were associated with a 29% higher odds of sustaining injuries than those of normal weight (OR 1.29, 95% CI 1.15–1.45; P<0.001).
Table 4
Multivariable generalized estimating equations (GEE) and random-effects logistic regression showing the association between weight status and workplace injury, National Longitudinal Survey of Youth 1979 (NLSY79) in 1988–2000. [OR=odds ratio; 95% CI=95% confidence interval; number of observations = 62 311; Wald chi-square = 783 (GEE) and 801 (random effects); var(random effects)=1.33]
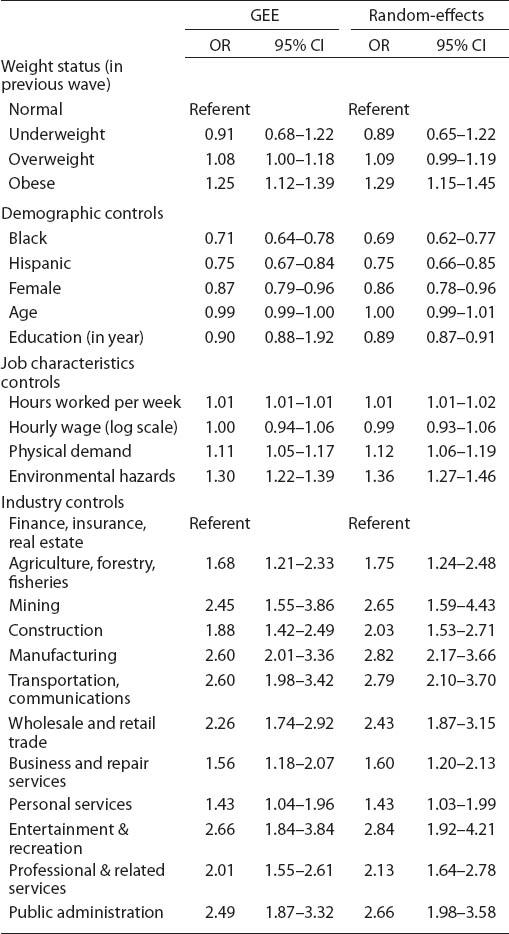
Discussion
We assessed the relationship between obesity and occupational injury using the NLSY79. Results of the statistical analysis indicated that obese workers were more likely to sustain injury at work than workers of normal weight. In both models, we controlled for potential confounders such as industry, work hours, and occupational hazards, in addition to typical demographic characteristics. The demographic profile of the sample was similar to the 1988 national distribution (40), except for a lower percentage of those who were of Hispanic origin in the sample (6% versus 8%) and a lower proportion of females (47.8% versus 49.8%). The prevalence of overweight and obese was comparable to findings based on other population studies (41).
Our result is consistent with prior studies based on smaller longitudinal samples (20, 25, 26) and cross-sectional population studies that address adults’ injuries and are not limited to the workplace (42–45). Overall, these findings suggest that obese workers (BMI ≥30 kg/m2) are at increased risk of injury with an OR range of 1.2–1.8. Additionally, the association for overweight workers (25.0–29.9 kg/m2) attained marginal significance, which suggests that a potential relationship between overweight status and occupational injury cannot be ruled out. Our results also agree with the broader literature regarding the adverse impact of excessive weight on workers. Obesity is associated with workplace disability (45, 46), long-term sick leave (47), and the cost of worker’s compensation claims (26).
Despite the strong documented associations, the mechanism of how excessive body weight relates to occupational injury remains unclear; future studies should examine possible pathways through which increased body weight affects workplace safety. For example, obesity is associated with many chronic conditions such as sleep apnea (48) and osteoarthritis (49) that may bring about sleepiness or physical limitations, potentially leading to a higher risk of workplace injury. Excessive body weight may alter the body’s biomechanics (50) resulting in postural instability (51) and weak balance (50), and conceivably increasing the likelihood of a fall (52). Further, obesity may interact with occupational hazards (10), elevating injury risk. For example, obese workers may find it hard to obtain appropriate personal protective equipment (25), increasing the chance of injuries in hazardous environments.
In our sample, BMI was missing for 4.8% of the observations between 1988–2002, with a high proportion of these being female (5.6%) and Hispanic (5.9%). But those with missing values for BMI did not differ significantly from those with BMI values in the proportion reporting injury. Thus missing values for BMI do not seem to have seriously biased the obesity-injury relationship. Missing data due to attrition or non-response is another source of concern. The random-effects model has an advantage in this regard: under the assumption of missing at random (ie, attrition depends on observed data but not unobserved data), the random-effects estimates remains consistent if the model is correctly specified (36). We note that missing at random is the most frequently invoked assumption in empirical analysis (53), although it is impossible to test whether attrition depends only on the observed variable or not; we do not have data for those who were not observed. Assuming that the missing-at-random assumption is reasonable, and given that the random-effects estimates are close to the GEE results, we feel confident that sample attrition did not substantially bias our study results.
The observed relationship in our analysis may be spuriously driven by other unobserved factors or biased by poor measurements of the predictors. For example, workers of lower socioeconomic status tend to have worse health outcomes including excessive body weight (54) and they are more likely to be involved with risky tasks (55), both elevating the risk of injury. We have used several variables to indicate a worker’s socioeconomic status, such as education, hourly wage, and occupation; still these variables may be far from complete, and the problem of residual confounding due to other social factors exists. To partially address the residual confounding problem, we conducted fixed-effects regression, which focuses exclusively on within-individual differences while controlling for all stable individual characteristics (56). The results from fixed-effects regression were qualitatively similar to our analysis.
There are other limitations to our study. First, the NLSY79 did not document a specific nature of injury or contributing event (fracture, fall, burn, being struck by an object), and we were therefore unable to conduct a more fine-grained analysis on the relationship between obesity and different types of occupational injury. Second, our outcome measure was self-reported, and individuals may have had varying definitions or thresholds for reporting injury. Third, BMI is correlated with body adiposity but does not directly reflect the proportion of body fat. The same BMI may indicate different levels of body fat and result in misclassification of obesity (57). Fourth, people tend to overstate their height and underreport their weight, which results in a self-reported BMI lower than then actual, potentially biasing the results toward the null.
Our study has several strengths. First, the current findings are based on a representative sample with a relatively large sample size, while most of the previous research used smaller company or industry-specific samples (14–26). Second, the NLSY79 cohort has a relatively high participation rate and low sample attrition, and the longitudinal nature of our sample allows us to ensure temporal precedence of the exposure. Third, we fit a population-averaged and subject-specific model; results from both indicated that obesity was associated with a greater risk of occupational injury. Fourth, a wide array of controls was included, such as basic demographic profile, industry, and job characteristics.
Concluding remarks
Occupational injury continues to pose a significant burden on workers worldwide, and lately obesity has been suggested as a risk factor for workplace injury. Many previous studies have included obesity as a covariate in their analysis, but the evidence regarding the relationship between obesity and workplace injury has been mixed. We assessed this relationship using data from a nationally representative cohort and found that obesity is significantly associated with occupational injury. Further research is needed to elucidate the mechanisms through which excessive body weight contributes to injury at the workplace, how obesity interacts with other occupational hazards, and what interactions may pose a hazard to workplace safety.