Pregnancy outcome is determined by the ability of the fetus to thrive, which depends on a complex combination of genetic, social, and environmental factors (1). If, during pregnancy, a mother is exposed to increased environmental or social stressors, this could result in an increased risk of restricted growth of the fetus or a preterm birth (PTB). These adverse perinatal outcomes are strong predictors for infant mortality and morbidity (2). Moreover, epidemiological evidence has suggested the long-term implications of a sub-optimal in utero environment with links to an increased risk for health conditions in later life, such as cardiovascular disease and type 2 diabetes (3, 4).
A range of environmental exposures during pregnancy have been investigated to understand better the contribution of environmental factors on the vulnerable fetus, including water contamination (5), electromagnetic fields (6) and pesticides (7). A large body of work now exists from around the world investigating the effects of air pollution on pregnancy and the subsequent birth outcomes, which have given rise to several recent reviews (8–10). Numerous studies have found evidence to support the hypothesis that air pollution can increase the risks of PTB (11–13) and impaired fetal growth (14–16); however, the results are not yet consistent enough to confirm a causal link with specific pollutants.
Limitations of previous studies mostly relate to exposure assessment methods that may not adequately capture spatial and temporal pollution variation, small sample sizes, and appropriateness of confounder adjustments (17). Most studies investigating the association between air pollution and adverse pregnancy outcomes use a retrospective cohort study design in order to be better able to achieve the necessary power to detect relatively small effects. A retrospective design presents the challenge of assigning pollution estimates that best represent the spatial and temporal heterogeneity of multi-pollutant exposure during an individual’s pregnancy; some exposure misclassification seems likely. A number of different exposure-estimation techniques have been designed and implemented to address this challenge (18). This heterogeneity of exposure-assessment techniques that exists in the current literature makes it difficult to synthesize results. Different exposure estimation techniques may lead to different effect sizes. Few studies implemented more than one technique in unison on the same population, which would allow a comparison of the different estimates provided and the effect on risk estimates (14, 19).
The objective of this study was primarily to use a novel air pollution estimation technique with strong spatial and temporal resolution to estimate the effects from pollutants known to cause adverse health effects on pregnancy outcomes in a large Northwest (NW) England cohort from 2004–2008. In addition, a more traditional exposure assessment method of using nearest stationary air pollution monitors was implemented as a comparison technique.
Methods
Study design and cohort
A retrospective cohort study was conducted in NW England on births that occurred between 1 January 2004 and 31 December 2008. NW England has a total area of 5469 sq miles and a (2011) population of 7 052 000 (20). Some of the major cities within the region record some of the highest air pollution levels outside of London in the UK (21).
Maternal and perinatal data between 2004–2008 were obtained from the NW Perinatal Survey Unit (NWPSU). The NWPSU is based in Manchester and, during this time period, collected maternal and perinatal data at the time of delivery from 21 of the 29 maternity units in NW England. The eight units that were not included were generally small, and there was no difference between the index of multiple deprivation (IMD) score (based on hospital postcode) between the included and excluded maternity units. Data included: hospital site, ethnicity, postcode, region, mothers’ date of birth, body mass index (BMI) at booking, parity, date of delivery, gestational age calculated from last menstrual period (LMP) and scan measurements, birth weight, multiple birth, live birth/stillbirth, type of delivery, and smoking.
All singleton live births recorded during 2004–2008 were extracted from the NWPSU (N=265 613) and the data were cleaned by removing all entries deemed to be implausible (birth weight <500 and >5500 g, N=336, and gestational age <24 and >44 weeks, N=274). Birth weight and gestational age entries were missing from 1235 and 12 833 births, respectively. The total number of births included in each analysis varied depending on the outcome and exposure measure and adjustment for covariates. Information on missing covariate data is presented in table 1.
Pregnancy outcomes
Three outcome measures were investigated: birth weight as a continuous variable, small-for-gestational-age (SGA) and PTB. SGA was defined as <10th percentile of birthweight for gestational age and sex in the NWPSU population (22). PTB was defined as <37 weeks completed gestation (2). Gestational age (GA) estimates were based on LMP data, wherever possible, and scan data when LMP data was missing or when the discrepancy was >7 days. The GA estimates were made in this way to reduce the risk of bias that may arise in scan estimates if fetal growth is influenced by air pollution in early pregnancy, resulting in the scan underestimating GA and thus overestimating PTB risk (23).
Covariate data
Potential confounders were determined primarily on a priori decisions based on previous evidence. Further exploration of data availability and the relationship between the considered covariates with the adverse pregnancy outcomes under study (table 1) and air pollution estimates (table 2) were used to make final decisions regarding confounders.
We considered the following variables as potential confounders: maternal age, ethnicity, socioeconomic status (SES, measured using the IMD score), birth season, parity, BMI, and smoking. All variables adjusted for in the analyses were treated as categorical variables.
Maternal age was categorized as <20, 20–24, 25–29, 30–34, 35–39 and ≥40 years. In the NWPSU population, 79% were recorded as white, therefore, ethnicity was dichotomized into white and non-white. The other ethnicities recorded in the NWPSU database were black African (2%), black Caribbean (1%), black other (1%), Indian (3%), Pakistani (8%), Bangladeshi (2%, Chinese (1%), and other (3%). SES was quantified using IMD scores for the maternal residence (24). The score comprises seven indices of deprivation weighted as follows: income (22.5%), employment (22.5%), health and disability (13.5%), education (13.5%), living environment (9.3%), crime (9.3%), and barriers to housing and services (9.3%). These scores were calculated at a lower super output level (a housing range of 400–1200) (25). The scores were then categorized into quintiles of deprivation based on English National Standards (24). Birth season was categorized as: Winter (December–February), Spring (March–May), Summer (June–August), and Autumn (September–November). Parity was included as a dichotomous variable: first birth or a higher order birth. BMI was recorded at the midwife booking appointment in the early stages of pregnancy and categorized using the standard cut-off points set by the World Health Organization (WHO) (26): underweight (<18.5 kg/m²), normal (18.5–24.9 kg/m²), overweight (25–29.9 kg/m²), and obese (≥30 kg/m²). BMI data was only available for 2006–2008. Maternal smoking data were recorded as a dichotomous variable stating if the mother was smoking at the time of delivery or not. Smoking data were only available for 2007 and 2008.
Exposure data
Air pollution exposure was estimated for each pregnancy for nitrogen oxide (NOx), nitrogen dioxide (NO2), particulate matter (PM2.5 and PM10) and carbon monoxide (CO). The primary exposure estimation technique implemented in this study was determined a priori under the assumption that the chosen method would result in the least exposure misclassification (27). The primary method was a spatio-temporal (S-T) air pollution model, which utilized annual mean background modelled concentration maps with a temporal adjustment from nearest stationary monitors. As a comparison to the primary exposure estimation technique, a more traditional method with cruder spatial resolution using traditional nearest stationary monitor technique (NSTAT) was implemented.
Nearest stationary monitor
All available postcodes of maternal residence at the time of delivery from the NWPSU (N=203 562) along with eight available stationary monitors located within NW England were geocoded using the geoconvert software (27) and mapped in ArcGIS [map of study area presented in supplementary material: figure S1, www.sjweh.fi/data_repository.php (28)]. Roadside stationary monitors were excluded because the estimates were not deemed representative of the wider study area. Automatic stationary monitor data were obtained from the UK automatic urban and rural network (AURN) managed by the Department for Environment and Rural Affairs (DEFRA) and Bureau Veritas (29). Each maternal residential location was linked to the nearest stationary monitor using the “join to nearest” function in ArcGIS. Monthly mean pollution estimates from the relevant monitor and time period were matched to the relevant corresponding pregnancy periods.
Background modelled concentrations
DEFRA’s pollution climate mapping (PCM) model (28) was used as the basis of the primary exposure assessment technique in this study. The PCM model calculates background annual concentrations at a resolution of 1 km². The modelling process is described in detail elsewhere (29, 30). The main points of the model development are the summing together of concentrations from several layers of data including: (i) large and small point sources from the National Atmospheric Emissions Inventory (NAEI), (ii) distant sources characterized by rural background concentrations, and (iii) area sources from the NAEI modelled using a dispersion kernel technique (29). Sources specific to PM formation were included in the calculation of PM estimates including: secondary inorganic and organic aerosol, iron and calcium rich dusts, sea salt (from measurements of chloride at rural sites), area, and regional sources of primary particles (from emission estimates from the NAEI).
The PCM model has demonstrated strong correlation and agreement with verification sites across the UK (29); the Air quality Modelling Review Steering Group recently found it performed well in independent comparison tests and deemed it suitable for use (31). The PCM model with an additional monthly temporal adjustment has been previously compared, along with other estimation techniques that can be implemented in large scale epidemiological studies, with personal exposures from a cohort in NW England and was found to be one of the strongest exposure estimation techniques (32). The model has been recommended for use in epidemiological studies investigating health impacts from air pollution; as yet however, it has only been implemented in one recent epidemiological study to the authors’ knowledge (33). The advantage of the PCM model over commonly used regression based methods is the incorporation of detailed data from emission inventories and meteorological data to model the dispersion of pollutants at a fine spatial level (30, 34).
Temporal adjustment
The PCM-modelled annual estimates were multiplied by monthly adjustment factors (MAF) enabling investigation of pollution effects during specific pregnancy periods. The temporal adjustments were derived from data from eight stationary monitors that had collected the relevant pollutant data and were situated in an urban background location. Stationary monitor site “types” are classified based on their location eg, suburban, urban, industrial, etc. An urban type of stationary monitor was selected to establish the most representative adjustment factors for the area. Each centroid point from the PCM map was linked to the nearest stationary monitor. The mean distance between the maternal residences and the stationary monitors was 465 m [standard deviation (SD) 967 m]. MAF were calculated for each monitoring site, where data was available, for each of the 60 months between January 2004 and December 2008 by dividing the monthly mean by the annual mean concentration of the pollutant concerned. The PCM annual mean concentrations were multiplied by the relevant MAF values. These temporally adjusted modelled estimates are referred to hereafter as the S-T model.
Data linkage
Each available maternal residence was spatially linked to the nearest PCM centroid point using the “distmatch” STATA command. Monthly exposure estimates from the S-T model and the nearest stationary monitor were assigned to each month of pregnancy and the three months prior to conception. Date of conception was calculated based on the recorded gestation subtracted from the date of delivery.
Statistical analysis
Logistic regression analyses were used for the dichotomous outcome variables SGA and PTB. Linear regression analyses were used for the continuous outcome measure of birth weight. Odds ratios (OR) for SGA and PTB are presented as the 4th quartile of pollution exposure compared to the 1st (quartile concentration cut-offs are presented in table 3). OR were calculated for the following pregnancy time periods: 3 months pre-conception, 1st trimester (weeks 1–13), 2nd trimester (weeks 14–27), 3rd trimester (weeks 28–to birth), and an average for the whole pregnancy period (conception to birth).
A test for trend was performed by including the exposure variables as continuous measures for SGA only because this was the only outcome where an effect was observed.
Adjustments were made for maternal age, parity, ethnicity, birth season, IMD, BMI, and smoking. Statistical significance was defined as P<0.05.
A further investigation of fetal gender as an effect modifier was performed by stratifying the results by female/male and calculating the OR for the whole pregnancy period. To determine if pollution effects varied by gender, a term representing the statistical interaction between pollution and gender was included in the logistic regression models and tested for statistical significance.
Results
Table 1 describes the covariates and their associations with the outcome measures PTB and SGA; the associations with birth weight change are also presented. The strongest association with SGA and reduction in birth weight was with maternal smoking (OR 2.23, 95% confidence interval (95% CI) 2.04–2.45 and birth weight reduction of 231 g, 95% CI -246– -217). The equal strongest associations with PTB were with maternal smoking and being categorized as underweight based on BMI scores (OR 1.47, 95% CI 1.20–1.80).
Table 1
Associations between population characteristics and preterm birth (PTB, <37 completed gestation weeks), small for gestational age (SGA, weight for GA and sex <10th centile of population) and birth weight. [OR=odds ratio; 95% CI=95% confidence interval; IMD=index of multiple deprivation; BMI=body mass index]
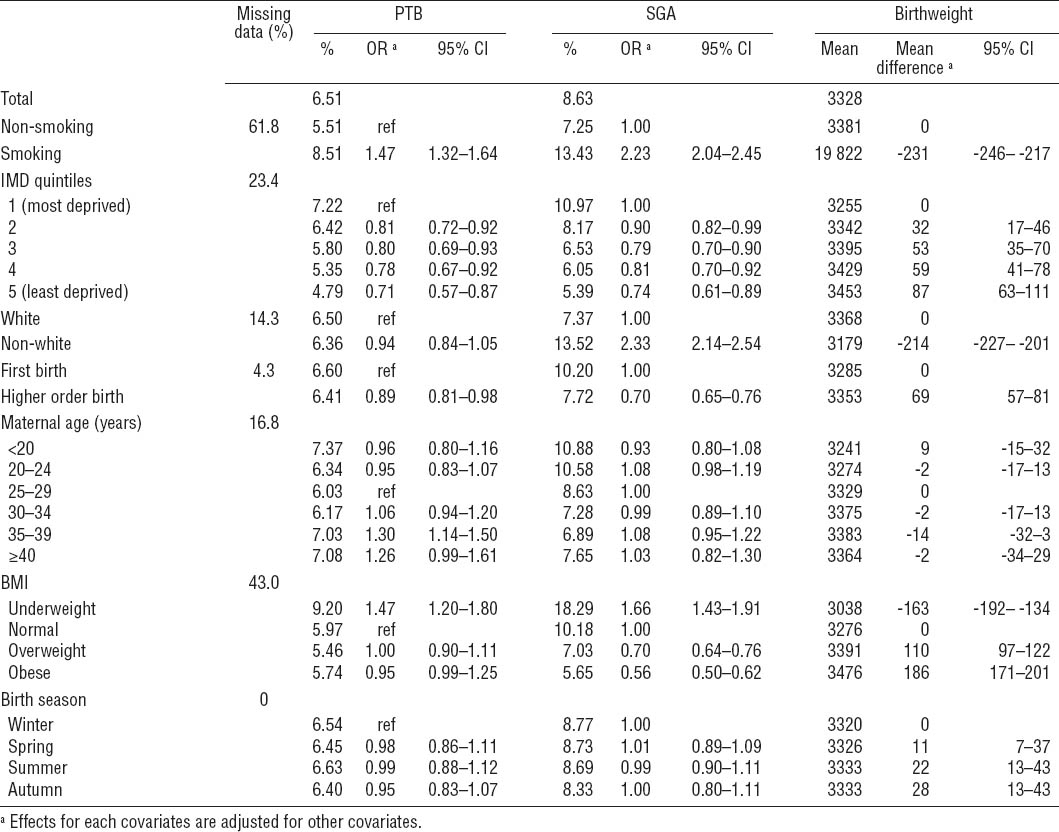
The descriptive statistics of each pollutant by estimation technique and pregnancy period are presented in table S1 of the supplementary material (www.sjweh.fi/data_repository.php). Table 2 demonstrates how each covariate relates to the exposure estimates and presents data based on first trimester NO2 estimates from the S-T model by quartiles. Maternal smoking rates did not vary much between the pollution quartiles: 3% more smokers were in the 4th compared to the 1st pollution quartile. A higher percentage of younger mothers (<30 years) lived in more polluted areas and vice versa for older mothers (>30 years). The strongest associations were with SES and ethnicity. There was a clear pattern of more deprived women living in more polluted areas. In the lowest air pollution quartile, 25% women are in the most deprived IMD quintile compared to 64% in the highest pollution quartile. Compared to white mothers, a higher percentage of non-whites lived in more polluted areas: in the highest air pollution quartile, 65% were white and 35% were non-white while in the lowest air pollution quartile, 9% were non-white and 91% were white.
Table 2
Associations between spatio-temporal modelled nitrogen dioxide (NO2) exposure (trimester 1) and covariates (column percentages). [SD=standard deviation; IMD=index of multiple deprivation; BMI=body mass index]
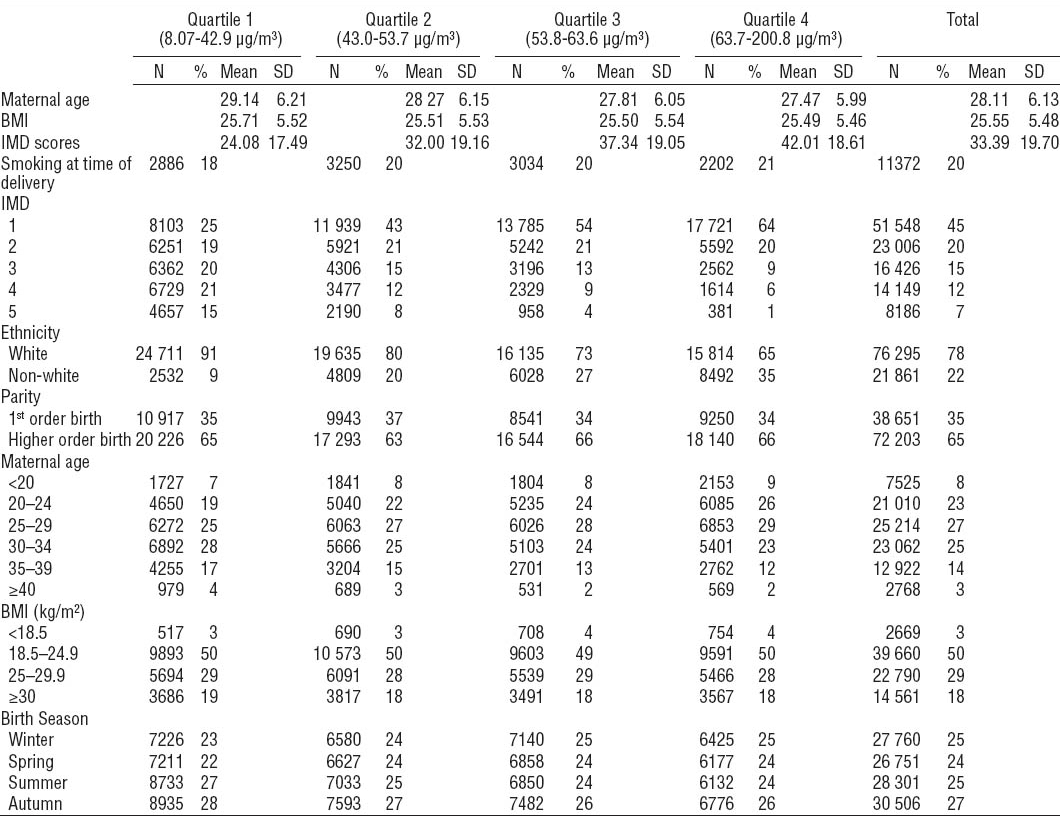
Table 3 presents the Pearson correlation coefficients and the descriptive statistics for the five pollutants and the two exposure-estimation techniques investigated. The mean pollutant concentrations were significantly higher based on the stationary monitor estimates compared to the S-T model. The strongest correlations were between NO2 and NOx (r=0.96) and with PM10 and PM2.5 (r=0.90). Correlations between the two exposure techniques were stronger for PM than NOx and NO2.
Table 3a
Pearson correlation coefficients and descriptive statistics for the included air pollution metrics. [NOx=nitrogen oxide; NO2=nitrogen dioxide; PM=particulate matter; CO=carbon monoxide; NSTAT=nearest stationary monitor technique; S-T model=spatio-temporal model]
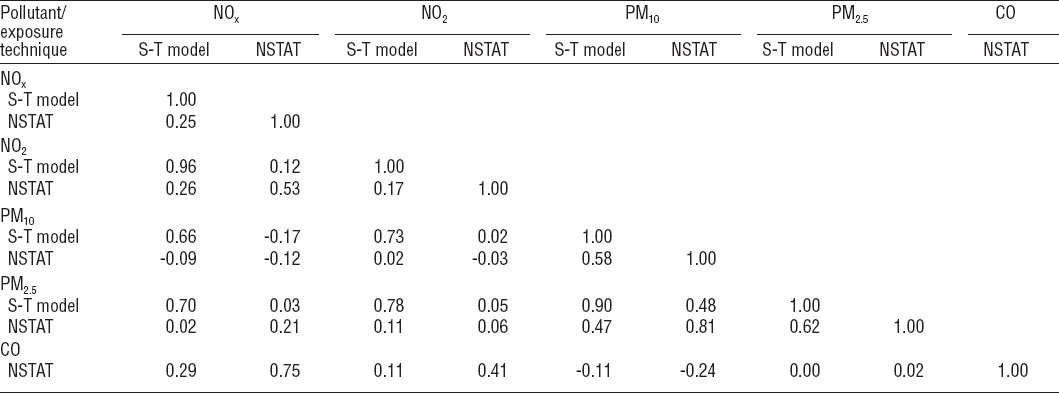
Table 4 presents the fully and unadjusted effect estimates for PTB, SGA, and mean birth weight change from both pollution estimation techniques based on exposure averaged for the entire pregnancy period. There were no significant positive associations between any of the air pollutants with PTB. Based on the S-T model, there was a significant increased risk of SGA with PM10 in the adjusted model (OR 1.14, 95% CI 1.01–1.29). CO estimates could only be made based on stationary monitor estimates and, although not statistically significant in the fully adjusted model, the effect estimates for SGA were suggestive of an association (OR 1.32, 95% CI 0.90-1.92). Generally, the effect estimates calculated from NSTAT estimates found lower effect sizes compared to the S-T model, especially for SGA and PM10. In sensitivity analyses, including only the mothers within the NWPSU who we were able to assign both S-T model and NSTAT estimates, the stronger effect estimates in the S-T model remained (table 5).
Table 4
Associations between preterm birth (PTB, <37 completed gestation weeks), small-for-gestational age (SGA, weight for GA and sex <10th centile of population), and birthweight with air pollution exposure from entire pregnancy period estimates based on the spatio-temporal (S-T) model and nearest stationary monitor technique (NSTAT) (4th quartile compared to 1st). [OR=odds ratio; 95% CI= 95% confidence interval; NOx=nitrogen oxide; NO2=nitrogen dioxide; PM=particulate matter; CO=carbon monoxide]
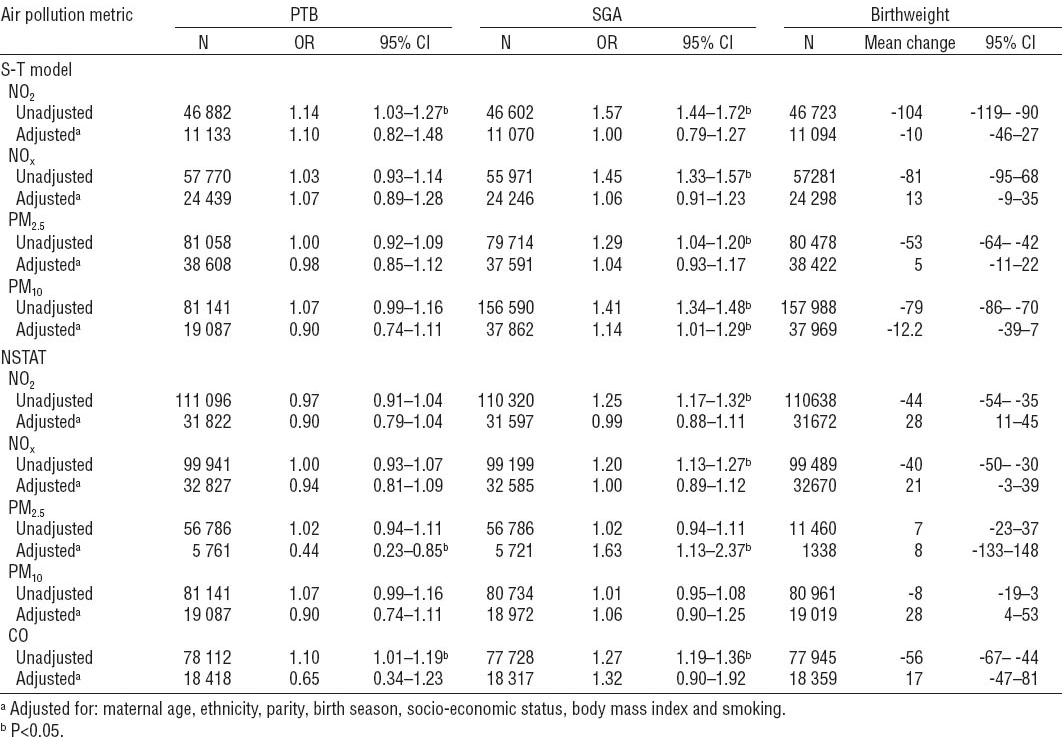
Table 5
Sensitivity analyses exploring the associations between (PTB, <37 completed gestation weeks) and small-for-gestational age (SGA, weight for GA and sex <10th centile of population) with pollutant estimates from the spatio-temporal (S-T) model and nearest stationary monitor technique (NSTAT) with the population who have both pollutant estimates available. [OR=odds ratio; 95% CI= 95% confidence interval; NO2=nitrogen dioxide; PM=particulate matter]
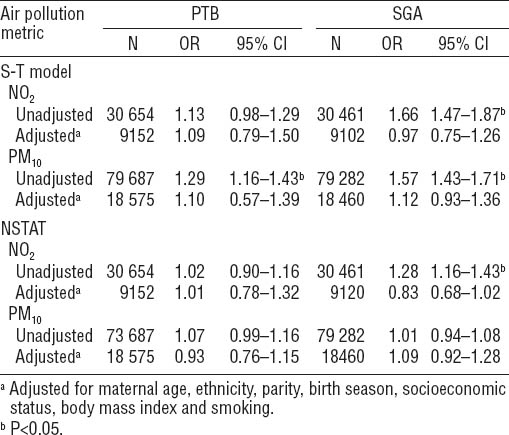
Table 6 presents the adjusted OR and mean birth weight change and the test for trend analysis between the air pollution estimates based on the S-T model and adverse pregnancy outcomes by specific pregnancy periods. Again, no associations were found with PTB. Significant associations were found with SGA in the third trimester for NO2 (OR 1.14, 95% CI 1.00–1.30) and PM2.5 (OR 1.10, 95% CI 1.00–1.21), with evidence of trend with exposure for PM2.5. Although significant associations were also found in the 3rd trimester with PM10 (OR 1.12, 95% CI 1.00–1.25), the effect estimates were very similar across all pregnancy periods. The strongest association was found with CO in the 2nd trimester (OR 1.21, 95% CI 1.02–1.42). In further exploratory analyses of the association between air pollution exposures based on the S-T model during specific pregnancy time periods in the white and the primiparous NWPSU population, the results demonstrated no strong differences with the whole NWPSU population (supplementary tables S4 and S5, www.sjweh.fi/data_repository.php).
Table 6
Adjusted a associations between preterm birth (PTB, <37 completed gestation weeks), small-for-gestational age (SGA, weight for GA and sex <10th centile of population), and birthweight with air pollution exposure based on the spatio-temporal (S-T) model by four pregnancy time periods. [OR=odds ratio; 95% CI=95% confidence interval; NOx=nitrogen oxide; NO2=nitrogen dioxide; PM=particulate matter; CO=carbon monoxide]
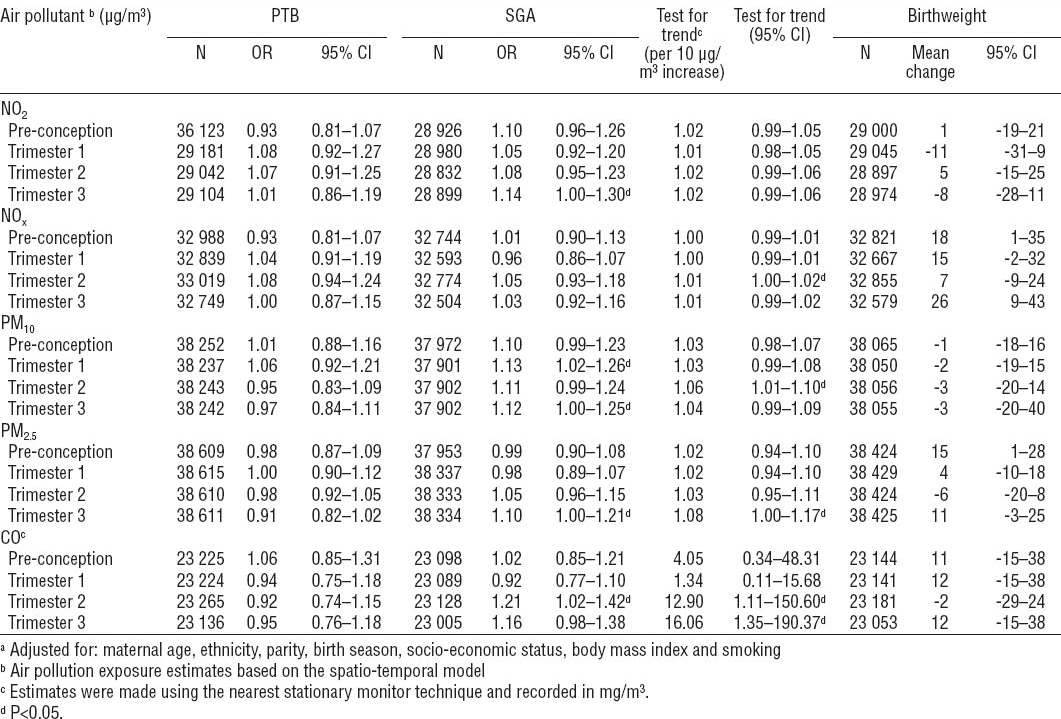
The associations based on the S-T model estimates for the entire pregnancy stratified by gender are presented in table 7. The data were suggestive of a differential effect of air pollution by gender with all the effect estimates higher among female compared to male infants, except for the association between CO and SGA; however, this was not statistically significant.
Table 7
Associations between air pollution a and preterm birth (PTB, <37 completed gestation weeks)/small-for-gestational age (SGA, weight for GA and sex <10th centile of population) stratified by gender (4th quartile compared to 1st). [OR=odds ratio; 95% CI=95% confidence interval; NOx=nitrogen oxide; NO2=nitrogen dioxide; PM=particulate matter; CO=carbon monoxide]
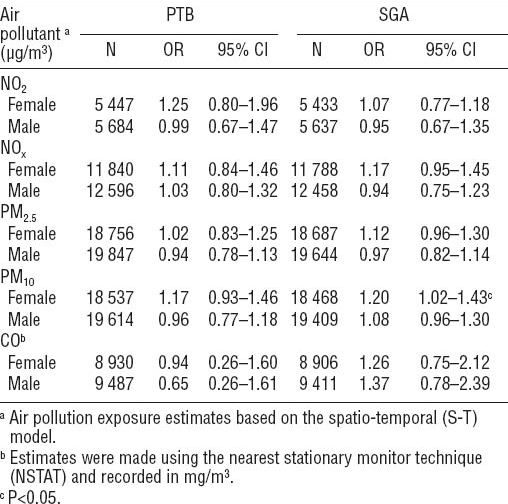
Discussion
Summary of findings
This large retrospective cohort study investigated the effects of air pollution, estimated using a novel high spatial (1 km²) and temporal (monthly) resolution exposure estimation technique as well as an additional comparison technique using nearest stationary monitors, with adverse pregnancy outcomes in NW England.
Our results found that there are no significant associations between any air pollutants and PTB. We found small statistically significant associations of PM10 averaged across the whole pregnancy period and NO2, CO, PM10 and PM2.5 at specific time points in pregnancy with an increased risk of SGA. The critical window of exposure of this association differed between pollutants. No association was found with NOx at any time point during pregnancy. There was no strong evidence of a particular critical window of exposure for PM10. For NO2 and PM2.5, an association was found in the 3rd trimester. CO was the only pollutant to exert the strongest effects during the 2nd trimester. The associations were stronger among females compared to males. Effect estimates based on NSTAT monitoring sites with poor spatial resolution were weaker than those based on the S-T pollution model.
In relation to other studies
The results from our study are consistent with much of the published evidence to date. Many previous studies have found no effects of air pollution on PTB with NO2 (11, 35), CO (11, 36), and PM (37). A recent comprehensive meta-analysis (8) found that the majority of studies reported an increased risk of LBW/reduction in birth weight in relation to the same pollutants that were found to have some effect on SGA in this study: CO, NO2, PM10, and PM2.5.
We found stronger effect sizes for PTB and SGA from air pollution exposure among females compared to males (aside from CO and SGA). However, this was not significant in the fully adjusted models. Other recent studies (14, 39) and a review (38) investigating the relationship between gender and air pollution reported an increased risk to fetal growth.
We found that significant effects of air pollution exposure with SGA were generally found later in the pregnancy stages. Most studies present results based on an average across the whole pregnancy period. However, more recent studies present results by trimester or month of pregnancy (37, 40). Previous studies have reported mixed results on the critical windows of exposure; based on evidence from the wider field of research, our later gestation findings seem plausible. A recent prospective study investigating the effects of air pollution on fetal growth assessed by ultrasound measurement throughout pregnancy concluded that maternal exposure is inversely associated with fetal growth and weight at birth in the 2nd and 3rd trimesters (41). Maternal smoking, although not directly comparable to ambient air pollution exposure, can help in ascertaining mechanistic pathways and critical windows of exposure for reduced fetal growth effects. A prospective study investigating maternal smoking habits and fetal growth using ultrasound measurements at 11 and 20 weeks gestation found that maternal smoking is also associated with reduced fetal measurements in the 2nd and 3rd trimesters (42). Recent work investigating critical pregnancy time windows to air pollution exposure through cord serum antibody response measurements found elevated Immunoglobulin E (IgE) levels in relation to PM2.5 exposure in later gestation (a peak in month 6) (43).
Biological plausibility
The extent to which pollutants have a direct toxic effect on the fetus or are involved in a cascade of events consequently affecting fetal growth is yet to be established. It has been suggested that air pollution (particularly NO2, CO, and PM10) are related to hematologic factors such as alterations in blood viscosity and coagulability (44, 45). This will likely lead to reduced umbilical blood flow, preventing adequate placental transfer of oxygen and other low molecular weight lipophilic molecules, consequently resulting in restricted fetal growth and an SGA outcome. This is a likely mechanism of effect in which air pollution may be restricting growth but not necessarily increasing the risk of a spontaneous PTB. Oxidative stress occurs due to excess free radicals (eg, NO2) and a decrease in antioxidant defences (46). Oxidative stress can have direct effects on placental function (47). Oxidative stress may lead to DNA damage causing disruption to DNA transcription resulting in an increased number of DNA adducts (48). No direct associations between increased DNA adducts and adverse pregnancy outcomes have been demonstrated, however, there is evidence of an increase in DNA adducts in areas with elevated air pollution (48) and links to reduced fetal growth (49).
This study found no association between air pollution and PTB. Although there is not a strong body of evidence to date that suggests an association between PTB and air pollution, those that have reported this association have postulated a range of likely mechanisms of effect. Air pollution may increase maternal susceptibility to infection (50), which is strongly associated with PTB [thought to account for 25–45% of PTB (51)]. Air pollution may induce an inflammatory response, which is linked to PTB (52). It is thought that the relative balance between pro- and anti-inflammatory cytokines may determine the timing of delivery. Air pollution exposure has been found to be associated with the pro-inflammatory cytokine Interleukin-6 (44). The results from this study do not provide evidence to support these mechanisms of effect.
Strengths and weaknesses
The main strengths of this study are the large sample size, the comprehensive adjustment for confounding factors, and the exposure assessment technique which takes into account both fine spatial and temporal variation of air pollution. However, although this spatial and temporal accuracy will help to reduce exposure misclassification, as with most similar large scale epidemiological studies, it remains that case that estimates are based on the maternal residence at the time of delivery. Individual mobility and time-activity data was not available for the cohort, therefore, we could not account for exposures that the subjects inevitably had when they were not at their residence (eg, in work or commuting) or if they moved during pregnancy. Modelled estimates and stationary monitors were based on outdoor ambient concentrations and not inside the home where most of the time is spent (48). However, strong correlations in air pollution levels between indoor and outdoor environments have been shown, particularly with particulate matter (r=0.73) (49).
Presenting results from epidemiological studies investigating the effects of air pollution on health outcomes using more than one exposure estimation technique has been encouraged (17). This allows for comparisons between studies, but also enables transparency in how effect estimates can be impacted by choice of exposure estimation technique. This study includes effect estimates from two estimation techniques one of which, primarily based on the PCM model, was considered a priori to be superior based on evidence from a specifically designed exposure estimation technique comparison study (32). The more traditional NSTAT with a relatively poor spatial resolution (based on eight stationary monitors throughout NW England) was included as a comparison because many studies have used this technique. The pollution estimates made from nearest stationary monitors only were on average higher than those made from the S-T model. There was a decreased strength of an association with adverse pregnancy outcomes based on estimates from stationary monitors compared to the S-T model. Under the assumption that the S-T model will have less measurement error than NSTAT, this is consistent with the well-known epidemiological concept that random error in exposure measurements generally biases an effect measure toward the null (50). It has been suggested that researchers should be more cautious in concluding there to be no causal association from a negative study if measurement error is thought to exist in a study (50). Under the most likely assumption that NSTAT is more prone to error, we may conclude that some previous studies implementing techniques with a poor resolution for estimating exposure may have attenuated effect sizes; our study gives some indication of the degree of attenuation. There may also be the possibility of a geographical influence on the different observed effect estimates due to small differences in the distribution of missing values between the two estimation techniques. Although presenting effect sizes from more than one exposure estimation technique provides additional strength to a study, further research is required to better understand the reasons behind observed effect size differences between estimation techniques.
A limitation of this work is the missing data within the NWPSU dataset for smoking and BMI, adjustments for these drastically reduced the sample size available. Although both variables were related to outcomes, neither was strongly related to exposure (table 2); as such they are not important confounders in this study, although adjustment is still preferable even for a small degree of confounding. The variables that had the most amount of missing data were maternal smoking (62% missing) and BMI (43% missing). This missing data was primarily due to the fact that data was not requested from the midwives on maternal smoking until 2007 and BMI until 2006. In view of the conflict between sample size and the need for adjustment, sensitivity analyses were performed in which there was adjustment only for the confounders with relatively little missing data (maternal age, ethnicity, parity, birth season, and IMD). As shown in table S3 (www.sjweh.fi/data_repository.php), the results were consistent between the fully adjusted analyses and the analyses without adjustment for BMI and smoking as expected from table 2. This suggests that the confounding effect lies with the variables maternal age, parity, birth season, and SES, rather than the variables that were a priori identified as potential confounders, namely, BMI and smoking. However, we felt it was important to show the fully adjusted results here despite the smaller sample size. Although administrative decisions were the primary reasons for missing BMI and smoking data in earlier years, there could still be some selection bias in our results due to other reasons for missing data. For example, it is possible that the women with missing smoking data in the years when it should have been recorded may have included a higher percentage of smokers. Due to the strong correlation between smoking status and low SES, this may have resulted in a selection bias towards women with a higher SES being included in the adjusted analyses. The possibility of residual confounding cannot be ruled out in this study. Although the confounders were decided a priori based on previous evidence, there were variables that would have been included if the data had been available, for example, noise pollution during pregnancy. There was also a risk of residual confounding from the included covariates, particularly with the variables maternal smoking and SES. Maternal smoking data was collected based on subject recall at the time of delivery with a yes/no response. This variable is unlikely to adequately capture all the women who smoked during their pregnancy. The IMD score provided a comprehensive measure of SES and is currently one of the strongest estimation techniques of SES at a population level in the UK (51). However, the score was calculated based on data at a lower super output area level (LSOA) and not at a street or individual level. A LSOA includes a range of 1000–3000 people and 400–1200 households (25). The NWPSU data was initially collected for audit purposes collecting information on all births within the participating units. Therefore, there is no reason to assume that there was any systematic bias in the reporting of data from particular birth outcomes that could result in misclassification of exposure effect estimates (eg, reporting more frequent in births with a poor outcome).
Guidelines from the WHO on air pollution thresholds based on epidemiological evidence of health effects published in 2005 and the most recent UK air quality legislation enforced in 2010 set annual concentration limits for NO2 and PM10 at 40 µg/m³ and 25 µg/m³ for PM2.5. Our data showed no evidence of effect on adverse perinatal outcomes for exposure below these limits (supplementary material, table S2, www.sjweh.fi/data_repository.php). Therefore, this study suggests that, in this study area, the current limits seem appropriate for protecting pregnant women from increased risks of an adverse perinatal outcome. However, based on the pollution estimates made in this study (2004–2008), a significant number of women were exposed to concentrations above the recommended (and now legal in the UK) concentrations. Of the NWPSU women to whom pollution estimates could be assigned for the whole pregnancy, 80% were exposed to mean concentrations of NO2 >40 µg/m³, 54% were exposed to concentrations >40 µg/m³ of PM10, and 19% >25 µg/m³ (the PM2.5 limit).
Concluding remarks
Based on a strong exposure estimation technique, this study suggests that there are small increased risks of an SGA outcome with maternal exposure to high concentrations of PM10 and similar effects observed for NO2, PM2.5, and CO in later pregnancy, particularly among female babies. The air quality standards currently set in place in the UK seem to be adequate in protecting fetal health; however, in some areas of the UK, these limits are not being observed. Up to 2008, long-term improvements in ambient air pollution have been identified in the UK, particularly with PM, but this has since remained stable (52). Up-to-date country-specific epidemiological studies investigating the health effects of air pollution are important to ascertain if limits set in place are (i) appropriate in protecting population health, including susceptible sub-populations, and (ii) being met.