Perceived fairness at work refers to the subjective evaluation of an individual’s contribution to work in relation to their resulting economic or social compensation (Adams, 1965 cited in 1, 2). It is a key component of two job stress models: effort–reward imbalance and organizational justice. In the model of effort–reward imbalance, fairness and reciprocity at work are conceptualized as part of a social contract, whereby an individual is rewarded in terms of money, esteem or career opportunities (3, 4). According to Siegriest et al (5), perceived lack of fairness or appreciation may result from the failure to obtain these rewards and lead to sustained stress reaction and dissatisfaction. The effort–reward model predicts that individuals who are particularly committed to work would be most affected by this perceived lack of fairness (5). The organizational justice model posits that justice involves perceptions of fairness at the organizational and individual level (1). According to this model, there are three main types of organizational justice: distributive (distribution of resources and benefits), procedural (processes and procedures for the distribution of resources), and interactional [either degree of dignity and respect received from managerial authority (relational) or presence or absence of explanations from the managerial authority about new procedures (informational)] (1).
There is growing research evidence that perceived fairness at work is an important determinant of physical and mental health (1, 6, 7), sickness absence (8), and turnover intentions (9, 10). In particular, evidence from a past review indicates that the most frequently studied aspects of justice (relational and procedural justice) can be shown to be reliably associated with mental health outcomes, in that poorer perceived justice is associated with worse mental health (1). There is also some evidence that distributive justice (eg, salary, rewards in relation to other employees) is associated with depression and sickness absence (1). Past review studies have consistently found that an imbalance in efforts to rewards is associated with a range of health outcomes, including cardiovascular disease, psychosomatic health, and job-related wellbeing (11).
Workers with disabilities may be particularly vulnerable to unfair and unequal treatment in the workplace. There is a range of potential reasons for this. Research suggests that employers perceive those with disabilities as difficult to accommodate due to assumed high cost associated with their employment (in terms of modifications to the workplace, as well as time off and sickness absence) (12–14). There is some evidence that, once in employment, people with disabilities are seen as less competent than coworkers without disabilities in similar roles (15) and are disproportionately relegated to entry-level occupations with lower levels of pay (16). Further, those persons with chronic conditions and disabilities are more likely to be in temporary employment, independent contracting, or part-time employment (17) and are much more vulnerable to job loss (12–14, 18, 19). Disability and fairness is a significant research topic from a policy perspective considering the recent Australian government attention to promoting the workforce participation of people with disabilities and reducing reliance on welfare services (20–22). This topic also has international relevance, as emphasized in the 2011 World Health Organization and World Bank Group report on disability (23). In Australia, as in many countries in the Organization for Economic Cooperation and Development (OECD), the labor force participation of those persons with disabilities (52.8%) is substantially lower than those without disabilities (83%), and this disparity has not changed over the past 20 years (24).
Past studies in this area have suffered a number of methodological problems that limit the extent to which disability has been shown to be the cause of unfair treatment at work. First and foremost is that people with disabilities have a number of demographic and socioeconomic characteristics (eg, age, socioeconomic position) that make them difficult to compare to people without disabilities. This means that it is difficult to determine whether disability is independently associated with perceived fairness at work, which, in these past studies, may also be due to other unadjusted demographic or socioeconomic factors. That is, confounding is an important possible explanation for observed associations of disability with perceived fairness.
One way to account for confounding, and thereby improve causal inference (25), is propensity score analysis (26). Propensity score matching estimates the probability of individuals being “exposed” to disability and allows comparison with “unexposed” people with similar demographic and socioeconomic characteristics, that is, with a similar profile of potential confounders. This approach has been successfully used in studies on working conditions (27, 28) and disability (29, 30). In this study, we use propensity score analyses on an employed cohort participating in the Household, Income, and Labor Dynamics in Australia (HILDA) survey between 2001–2012 to assess whether the presence of a disability is independently associated with lower fairness of pay. Using a propensity score approach to estimate differences in perceived pay between groups of people with and without disabilities is an important alternative method to “standard” multivariable regression adjustment to estimate the unbiased association of disability with perceived unfairness, and hence the causal association in the absence of confounding, random error, measurement error, and selection biases.
Method
Data source and eligibility for sample
The HILDA survey is a longitudinal, nationally representative study of Australian households established in 2001. It collects detailed information annually from over 13 000 individuals within over 7000 households (31). The response rate to wave 1 was 66% (31). The survey covers a range of dimensions including social, demographic, health, and economic conditions using a combination of face-to-face interviews with trained interviewers and a self-completion questionnaire. Although data are collected on each member of the household, interviews are only conducted with those aged ≥15 years.
The initial wave of the survey began with a large national probability sample of Australian households occupying private dwellings (31). Interviews were sought in later waves with all persons in sample households who turned 15 years of age. Additional persons have been added to the sample as a result of changes in household composition. For example, if a household member left his or her original household (eg, children left home, or a couple separated), he/she formed an entirely new household including all persons living with the original sample member. Inclusion of these new households is the main way in which the HILDA survey maintains sample representativeness. A top-up sample of 2000 people was added to the cohort in 2011 to allow better representation of the Australian population using the same methodology as the original sample (ie, a 3-stage area-based design) (32). The response rates for the HILDA survey are >90% for respondents who have continued in the survey and >70% for new respondents being invited into the study (31).
The analytic sample was restricted to those in paid employment aged 25–64 years and with a consistent pattern of disability reporting. To be classified as exposed, a person reported at least two consecutive waves of disability (T1 and T2), while to be unexposed, a person reported two consecutive waves of no disability (T1 and T2) and had no reports of prior intermittent disability (eg, single reports) in HILDA. Fairness of pay was measured in the year following two consecutive reports of disability (T3) to ensure that the outcome was measured subsequent to exposure. Covariates were also measured in T3, contemporaneous with fairness of pay. Individuals could only contribute once to the analysis. If there were individuals who experienced both two consecutive waves of disability as well as two consecutive waves without disability (qualifying as both exposed and unexposed), they were allocated to the exposed group in order to maximize the numbers in the exposed sample. A conceptual description of the sample can be seen in supplementary file 1 (www.sjweh.fi/data_repository.php) with accompanying description. As a sensitivity analysis we analyzed persons who reported three consecutive waves of disability or non-disability (results in supplementary file 2, www.sjweh.fi/data_repository.php)
Outcome variable
The primary outcome was perceived fairness of pay, as measured through the item “I get paid fairly for the things I do in my job”, which has been measured in all waves of the HILDA study. This item was assessed using a 7-point scale ranging from 0 “strongly disagree” to 6 “strongly agree”. This item has been used in previous research as a measure of fairness, as described in job-stress models such as effort–reward imbalance, organizational justice, and psychosocial job quality (33).
Exposure variables
The measure of disability used in the HILDA survey was based on the definition used in the International Classification of Functioning, Disability, and Health (34). Disability was defined based on reporting over two consecutive waves, ascertained from the following survey question “…do you have any long-term health condition, impairment or disability that restricts you in your everyday activities, and has lasted or is likely to last, for six months or more?” While this question was being asked, specific examples of long-term conditions were shown on a card, such as limited use of fingers or arms, or problems with eyesight that could not be corrected with glasses or contact lenses. These questions were asked at every wave. Data were available from 2001–2012.
Covariates/variables used in the propensity model
Covariates were included that could reasonably be considered confounders of the relationship between disability and perceived fairness of pay, as well as variables associated with the outcome (disability) (35). This approach to propensity score analysis has been shown to be associated with the largest reduction in bias in estimates due to confounding compared to alternative methods (26, 36, 37). Variables included in the propensity score were measured at T3 to be contemporaneous with the measurement of the outcome variable. As many of these factors are time invariant, they are also likely to reflect a person’s experience at the time of exposure (T1 and T2).
The variables selected for the propensity score model included gender (male or female), age group (25–34, 35–44, 45–54, and 55–64 years), education (did not complete secondary school, diploma and finished year 12, completed a university degree) and country of birth. Father’s occupational skill level was included as a measure of childhood socioeconomic status [low (laborers, machinery operators and drivers, sales workers), medium (technicians and trades workers, community and personal service workers, clerical and administrative workers), or high (managers and professionals)] coded according to the Australian and New Zealand Standard Classification of Occupations (ANZSCO). We also included year of the HILDA survey as a control variable to account for contextual effects of year on the relationship between disability and perceived fairness of pay.
Analytic approach
Stata (version 13) was used to conduct the propensity score analysis using psmatch2 commands (38). We first estimated the propensity for each individual to have a disability using a binary logistic model with disability as the dependent variable. As described above, covariates in the model were selected based on an assessment of their likelihood as confounders of the relationship between exposure and outcome.
We then used nearest neighbor matching with replacement, which matches each person with a disability with individuals who do not have a disability who have similar propensity scores, within a caliper of 0.01 (using 1:5 matches per observation).
Estimates for fairness of pay were calculated using the average treatment effect (the population average of the difference between the observed and potential outcomes for each subject) with 50 bootstrap replications. Diagnostics included assessing whether matching on propensity score reduced the overall imbalance in potential confounders, based on the standardized difference between persons with and without a disability (aiming for below a 10% reduction in imbalance). The standardized difference was calculated as follows:
If and
represented the prevalence of confounders observed in the exposed and unexposed groups, the percentage standardized difference
is (36):
Alternative propensity techniques aside from matching were tested including stratification, covariate adjustment using the propensity score, and inverse probability of treatment weighting.
We also compared the propensity score approach against standard multivariate regression analysis. The model included perceived fairness of pay as the outcome, disability as the exposure, and all the variables included in the propensity score as potential covariates. As a sensitivity analysis, another model included variables in the propensity score analysis as well as employment arrangement and occupational skill level as covariates, which were not included in the original propensity score approach as they were seen as potentially being on the causal pathway from disability to fairness of pay (ie, potential mediators). Thus, we have two regression models: one that includes confounders excluding potential mediators (including age group, sex, country of birth, education, father’s occupation and year of survey) and one that estimates the effect of disability on fairness of pay including employment arrangement and occupational skill level along with confounders (age group, sex, country of birth, education, father’s occupation and year of survey).
Results
The mean of fairness of pay was 4.65 (standard deviation 1.68). Table 1 demonstrates the standardized difference in covariates before and after the matching procedure, as well as difference in the sample size between the unmatched and matched analysis. Persons not included had no suitable match in the propensity score analysis and were therefore excluded. As can be seen, for all variables apart from age, the difference in covariates after propensity score matching is reduced for most variables to <5%. While some imbalance on age remains, the largest reduction in difference was for the youngest age group with a reduction of 5%. There was a 4.2% reduction in bias for the oldest age group. We assessed the likelihood that the remaining imbalance in age affects results in regression analysis (described below).
Table 1
Percentage standardized differences (SD) before and after matching on the estimated propensity score.
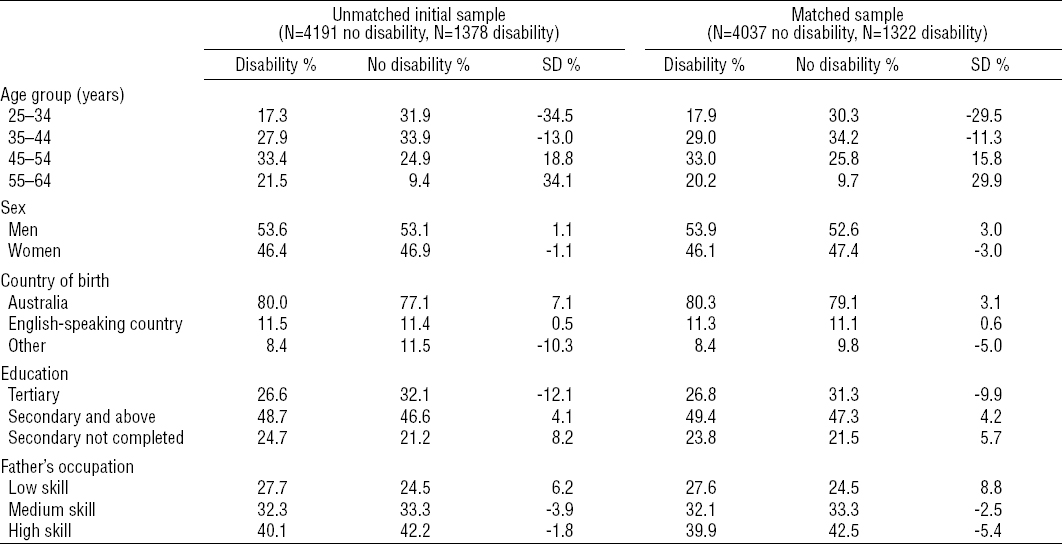
Results of the propensity matched analysis can be seen in table 2 and shows the average difference in fairness of pay between those with and without a disability was -0.31 [95% confidence interval (95% CI) -0.46– -0.16]. These are small effects and represent less than half a standard deviation difference. Results for the other propensity score techniques can also be seen in table 2, including adjustment for the propensity score in regression analysis (-0.26, 95% CI -0.37– -0.14) and additional assessment of age and propensity score (given the poor equalization of age as shown in table 1, -0.27, 95% CI -0.39– -0.16). This shows that after adjustment for both the propensity score and age the estimated mean difference in fairness in pay was similar to results in the matched analyses. Effect estimates are broadly consistent in inverse probability of treatment weighting (IPTW) (-0.26, 95% -0.39– -0.13) and stratification (-0.25, 95% CI 0.37– -0.14) but are of slightly lower magnitude. The sample described in table 2 refers to the propensity matched sample, which is smaller than the sample used in the unmatched regression analysis (table 3).
Table 2
Parameter estimate from propensity score (PS) models of the mean difference in perceived fairness of pay between people with and without a disability (N=4037 no disability, N=1322 disability) [95% CI=95% confidence interval; IPTW= inverse probability of treatment weighting]
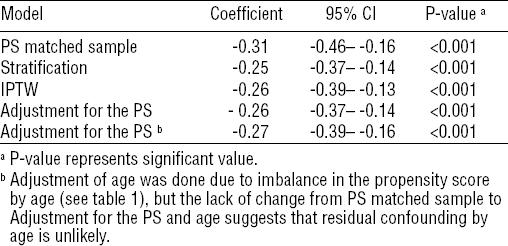
Table 3
Parameter estimate from the multivariable regression models of the mean difference in perceived fairness of pay between people with disabilities and those without a disability (N=4191 no disability, N=1378 disability) [95% CI=95% confidence intervals]
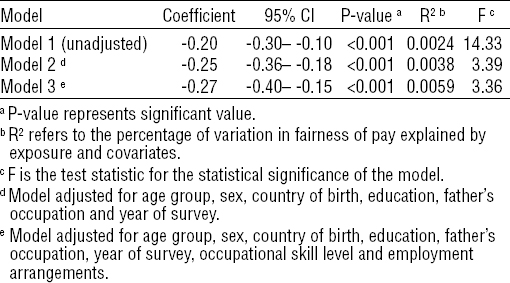
Table 3 shows the results for the standard multivariate regression analyses. In the full sample, the average difference in fairness of pay between those with and without a disability was -0.20 (95% CI -0.30– -0.10), ie, those persons with a disability reported a 0.20 lower score on the 7-point scale on average than those without a disability. After adjustment for the confounders age group, sex, country of birth, education, father’s occupation and year of survey, mean difference increased to -0.25 (95% CI -0.36– -0.18). This result closely agrees with that for the propensity score matching described above. When further adjusting for two potential mediators, occupational skill level and employment arrangements, the average difference was essentially unchanged (-0.27, 95% CI -0.40– -0.15) suggesting no detectable mediation through these variables.
Results when sample-restricted to three consecutive waves of reported disability or non-disability can be seen in supplementary file 2 (www.sjweh.fi/data_repository.php). As can be seen, results are broadly consistent with those reported in table 2 and 3.
Discussion
This study used two methods to address measured confounding factors of the disability and fairness of pay association, finding similar results. Assuming that we have captured the key measurable confounders of the association, and there are no major biases due to measurement error, selection bias or random error, our results indicate that disability is independently associated with perceived workplace fairness of pay. We would acknowledge that the magnitude of differences is small. However, this finding is of policy and public health significance given the known links between fairness of pay and health (1, 6, 7) and the increasing national (20–22) and international (23) attention encouraging the participation of people with disabilities into work.
The findings of this study support previous research suggesting that people with disabilities experience discrimination and inequity, both in finding work (39) and once in the workplace (15, 39). Employer discrimination is a problem, particularly for those from low-income backgrounds (40). In addition to this, there is evidence that people with disabilities are paid less than those without disabilities in Australia. For example, previous analyses of the HILDA survey reports a 7% hourly wage gap between workers with and without disabilities in Australia (35). Our study represents a novel contribution to previous research as it examines perceived pay fairness, which provides an indication of the extent workers feel they are fairly renumerated for their efforts. Study results not only support past quantitative research demonstrating real inequities in pay, but also highlight that workers with disability perceive these inequities. As Clark (41) argues, in relation to women, disabled workers may have such low expectations about work that they report higher job satisfaction, irrespective of their working conditions. If this logic is true then it is possible that the gap in perceived fairness of pay between disabled and non-disabled workers is lower than the difference that would be estimated using objective measures of pay differentials. This is problematic given that perceived inequity at work is related to a range of adverse health-related and organizational outcomes (2, 4, 6–9, 11, 42). Although explored in qualitative research (13, 43), there has been limited quantitative assessment of fairness and equity among workers with disabilities. Thus, the study represents an important contribution to disability research.
This study also extends the general body of literature on fairness at work. Most previous studies on fairness or organizational justice have been conducted in the general employed population or clinical (hospital based) samples (11). There has been no research investigating perceived fairness of pay among workers with disabilities, compared to counterparts without disabilities. Therefore, our investigation provides a description of fairness in a subgroup of workers that has thus far been neglected in job stress research. Further, past studies on fairness at work have focused on health outcomes (4, 7, 8, 11), and have failed to provide a robust assessment of the causal factors that lead to perceived unfairness at work in different sectors of the workforce. This study is distinct from previous studies in its particular focus on disability as an independent cause of unfairness at work, after ruling out the role of person-related, demographic, and other socioeconomic factors.
The OECD has emphasized encouraging employment among people with a disability (44). There are a number of social and economic reasons for this, including increased economic productivity, reduced costs associated with disability pension, and improved health. In Australia, reducing unemployment rates among those with disability by just one third would result in a $43 billion increase in GDP over a decade (equivalent to an increase in 2011 GDP of $12 billion) (45). This is a feasible target given that countries similar to Australia such as New Zealand have achieved this (44). Our previous research suggests that, compared to when they are employed, persons with disabilities experience a considerably lower mental health when they are unemployed or economically inactive (46). This research supports the large body of work showing that people who are employed have overall better health than those that are unemployed or economically inactive (47, 48). The OECD report highlights key factors that will help people with disabilities find and stay in work. These include greater employer commitment to hiring and retaining workers with disabilities in the workplace, promoting positive attitudes towards these workers, and creating high-quality work. Educating employers is central to overcoming many of these barriers. For example, employers could take a role in resolving perceived unfairness at work, both by taking the time to listen to workers concerns about why they feel they are not being adequately rewarded for their work, as well as matching the skills (and pay) of workers to the work they are doing. Employers may require government support in order to do this, both financially and in terms of strong social employment policy. In Australia, as in many other developed countries, employers can receive financial assistance to make workplace adjustments (eg, communication technologies, physical workplace adjustments, disability awareness training), however it is likely that many employers are not aware of these entitlements.
This paper uses nationally representative, longitudinal data to examine the relationship between disability and fairness of pay in Australia. It uses an approach to estimate the association between having a disability and perceived fairness of pay that accounts for demographic and socioeconomic differences between people with and without a disability. However, our paper is not without limitations. For example, the main outcome used in this study is only one measure of fairness at work. Therefore, we cannot say that this is a comprehensive measure of fairness at work according to either the effort–reward imbalance or organizational justice models (described in the introduction of this paper). Also, our estimates of the differences between the disabled and non-disabled group in perceived fairness of pay may be still confounded by unmeasured factors (49) or residual confounding due to poorly measured confounders. It is further problematic that we were unable to entirely eliminate imbalance in age therefore there could potentially be residual confounding by age; however, a regression model including the propensity score and age as a covariate showed similar associations between disability and fairness of pay as in the propensity score matching, suggesting that age imbalance may not be too problematic. There is some debate about the variables to be included in propensity score models. Some researchers advocate including all those variables that predict treatment assignment, while others suggest including all variables potentially related to the outcome, and still others advocate including only variables that are associated with both treatment and outcome (ie, satisfying the properties of a confounder) (37). Selection bias, whereby the associations of disability and fairness differ in the full eligible population and those actually retained in the study, may arise with attrition. However, retention was over 90% into the propensity analysis, and for such selection bias to arise would require strong associations of “selection variables” with both the exposure and outcome. This process includes loss to follow up, which is a major issue in all analyses of longitudinal studies (although, retention in the HILDA survey is relatively high as discussed above) (31). We must also acknowledge that both our outcome and exposure variables are self-reported; thus there is a possibility for dependent misclassification, whereby errors in the exposure and outcome are correlated (eg, one’s propensity to self-report disability, given “true” disability status, is correlated with one’s propensity to self-report fairness at work). Further research should include objective measures (if possible) of one or other of disability or fairness to overcome this potential bias.
A propensity score approach can reduce confounding as observed covariates are balanced at each particular value of the propensity score (25). This is analogous to randomization procedures used in clinical trials, as on average the distribution of covariates will be balanced between the exposed and unexposed groups, therefore the exposed and unexposed groups are “exchangeable” (26). This strengthens causal inference and thus improves the methodological quality of observational research. A strength of using a propensity score analysis is that it explicitly forces the researcher to consider imbalance in covariates between the exposed and unexposed. Those participants that cannot be matched are discarded, which reduces sample size but increases internal validity as only exposed participants with exchangeable unexposed participants are retained in the analysis. This procedure ensures that an “off-support” inference is avoided ie, the positivity assumption is not breached (50). In our analysis, we continued to observe imbalance in age even after propensity score matching. In saying this, the propensity scores approach in this paper provides corroborative evidence of the independent and causal contribution of disability on perceived fairness at work, albeit with concern about age imbalance. We recommend that researchers use propensity scores in two ways or steps: (i) to simply plot propensity score distributions for the exposed and unexposed, looking for non-overlapping distributions (ie, levels of the propensity score where there are only exposed or unexposed observations), such observations should be removed from the analysis; (ii) as an alternative analysis alongside other analytic approaches such as regression modeling.
In conclusion, our research suggests that the presence of a disability is independently associated with greater self-reported unfairness of pay. This is problematic considering that lack of fairness in the workforce is related to a range of adverse outcomes, including ill health, turnover and sickness absence. From a policy and employer perspective, there is a need to address lower equity in pay for those people with disabilities. As we suggest above, one of the reasons for this pay inequity may be discrimination against those people with disabilities. This research suggests the need to invest in improvements in the quality, pay, and sustainability of employment for people with disabilities. Not only does this hold the potential for economic and social benefits and reduce reliance on government welfare services, high quality employment can improve the overall wellbeing of working age people with a disability.