It has been estimated that globally in 2013 about 700 000 deaths and 55 million disability-adjusted life-years (DALY) are attributable to occupational risks (1). Identification of high-risk jobs for workers’ health is pivotal not only to identify the underlying occupational causal agents but also to implement focused preventive strategies aimed at eliminating or at least reducing exposures and so avoid the associated disease burden (2). Large population-based epidemiological studies are the ideal setting to identify which occupations are associated with adverse health effects because of the broad variety of industries, jobs, and exposure levels found in a general population. However, an important limitation of such large studies is the cost of occupational exposure assessment. There is no standardized method to collect individual lifetime job histories in epidemiological studies but the most common approaches are: (i) face-to-face personal interviews, (ii) self-administered job questionnaires, (iii) retrieval of job/industry titles from contemporary and historical occupational records, or (iv) record linkage with national administrative registry-based employment data, such as those available in the Nordic Countries (3). These data, typically in narrative form, must then be translated into meaningful job/industry titles and subsequently converted into standard codes to be usable in statistical analyses. Traditionally, the most reliable approach is considered to be manual coding by trained staff who assign occupation and industry codes based on standard occupational classifications. This process is very time consuming (and expensive) and thus often unfeasible in large epidemiological studies. An alternative approach is the use of available automatic web tools (eg, CASCOT) (4), but the validity and reliability of these systems ranges from poor to uncertain and is greatly affected by the quality of the input job description data. Text entry errors, misspellings, non-standard abbreviations, transposed occupation and industry, and listing of multiple occupations and industries are examples of why some manual coding is nearly always required following use of an automated coding system (5). Furthermore, manual coders, even if trained, can only access second-hand information, frequently of low quality, and may have limited expertise in jobs in some industrial sectors resulting in important job misclassification or missing job coding.
To overcome the above limitations, a novel, efficient and reliable method to both collect and code occupational lifetime job histories is needed, combining the validity of manual standard coding with the efficiency of automatic software. Our aim was to develop a web-based tool named OSCAR (occupations self-coding automatic recording) to enable individuals to construct their own job history through a simple web interface, based on the premise that only the worker knows exactly what his/her job is and is therefore the best person to collect his/her lifetime job history.
Methods
OSCAR development
OSCAR was originally designed for the UK Biobank study (6), a large population-based cohort including over 500 000 men and women aged 40–69 years recruited throughout the UK between 2006–2010, principally to estimate the burden of work-related chronic obstructive pulmonary disease (COPD). OSCAR can be used to investigate the association of occupational exposures with any health outcome.
We designed OSCAR not only to be easy and quick to use (with a target completion time of 20 minutes), but also reliable. To achieve this we developed a categorical decision tree based on a simplified but faithful version of the hierarchical structure of the UK Standard Occupational Classification (SOC), version 2000, to create job categories to enable participants to quickly and easily find each job they have held. The UK Office for National Statistics (ONS) developed SOC (7) to classify all occupations in the UK general population into standard codes according to the following hierarchical structure: 9 major groups (1-digit SOC codes), 25 sub-major groups (2-digit SOC codes), 81 minor groups (3-digit SOC codes), and 353 unit groups (4-digit SOC codes); the higher the number of SOC digits, the greater the level of detail in the job definition (available at http://webarchive.nationalarchives.gov.uk/20160105160709/http://www.ons.gov.uk/ons/guide-method/classifications/archived-standard-classifications/standard-occupational-classification-2000/index.html). SOC was developed for statistical purposes, and its language (eg, elementary trades and related occupations) is often technical and not easily understood without formal training. For these reasons, we undertook a comprehensive rewording of the job categories and titles to help participants recognize and self-allocate each of their jobs. In addition, we simplified the SOC hierarchical structure to a three-level decision tree displayed in OSCAR as job lists on three linked webpages starting with the major job groups (proxy for 1-digit SOC codes), followed by the job subgroups (proxy for 2- and 3-digit SOC codes) and ending in specific job titles (ie, the original 353 4-digit SOC codes). On selection of a final job title, a hidden 4-digit SOC code is automatically assigned to that job and retained in the database. Further, in the grouping of job categories, we did not follow the same education-based criteria used by SOC, but rather an analogy-based one: eg, engineer and engineer technician were grouped into the same major job group category (based on the similarity of their jobs) while in the original SOC 2000 they are categorized separately because of their different respective educational levels (professional versus technical) and were then distinguished at the final job-title level (eg, civil engineer coded as “2121” versus engineering technician as “3113”). All the original 353 4-digit SOC codes are included in OSCAR to ensure that almost all jobs in the UK general population are covered. There is no standard classification that can cover in detail all existing jobs, for which reason the SOC classification includes categories for jobs “not elsewhere classified”. In instances where an exact job title could not be found, participants were asked to select the closest match. Because some jobs are intrinsically generic (eg, secretary) and are therefore found in different job sectors, we duplicated these job titles across different job categories to ensure that a participant could find his/her job easily. “Warning” and “hint” messages including examples of key jobs included in each job category were displayed as “tool tips” throughout the process to assist participants in their progression through the decision tree to the final job title. Finally, we used a color system to differentiate the major job groups (designed to be a proxy for industry sectors) and the subsequent job groupings, and job titles to help participants self-allocate the correct job while progressing through the three webpages.
Implementation of OSCAR on the UK Biobank website
OSCAR was implemented as a web-based platform and hosted by the Clinical Trial Services Unit (CTSU), at the University of Oxford, Oxford, UK. It should be noted that OSCAR is also compatible with any kind of electronic device (eg, phones, tablet devices).
An introductory screen informed Biobank participants about the OSCAR process and inclusion criteria for the collection of jobs as specified by our particular research requirements (ie, that participants only needed to consider all paid jobs held for ≥6 months and for an equivalent of 15 hours per week since they left full-time education (school/college/university).
In order to internally validate OSCAR, we also asked participants to type their job title (in free-text format), as well as the year they started and ended each job; they then proceeded to find their job category for the specific job using the standard OSCAR process. We also asked them to enter dates of any gaps in their work history and the reasons for these (eg, maternity leave, unemployment, further education, etc.). A job-history timeline table was progressively built up as they entered each job, which allowed participants to visualize their job history over time, with the option to amend any entries at any time in the process. Participants were allowed to count several consecutive similar jobs for different employers as one (eg, a doctor at different hospitals).
Further submodules included questions for each job on the number of hours worked per week, shift patterns, and exposure to specific occupational agents. After completion of their job history, Biobank participants were asked to complete some questions on their respiratory health and smoking habits to address specific questions posed within our research project.
As an illustration of the overall process, an example of the selection of the job “bricklayer” using OSCAR is displayed in figures 1–4.
Figure 2
Major job groupings webpage for participants to select the most suitable job sector (construction sector is selected as an example).
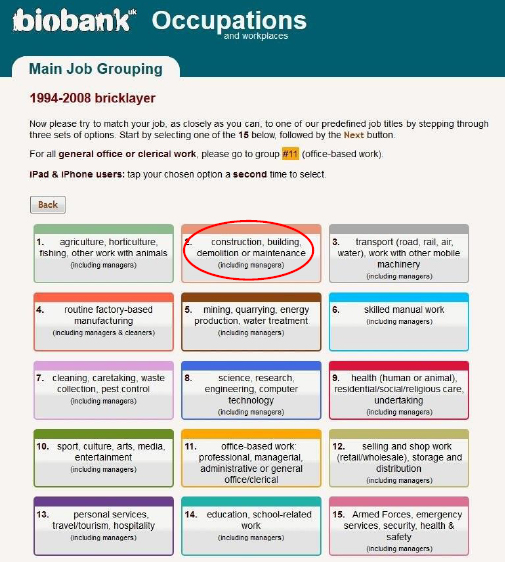
First, the Biobank participant is asked to type in the job title and the corresponding year or age at the start and end of this job (figure 1). The participant is then invited to find the same job through a series of job lists; of note only one selection among the multiple job categories displayed for each job held was allowed by the system. The first job list displayed on the webpage corresponds to the first SOC major job groupings (displayed as 15 colored boxes in figure 2). A red circle has been superimposed to indicate the most appropriate selection for the bricklayer job.
By selecting the major job grouping, the participant automatically progresses to the next webpage where more detailed job categories included in the construction job sector are displayed. Again, the participant would drill down to find the job title that is most suitable, in this case the first option (figure 3).
After this selection, the participant progresses to the final webpage where he/she will select the most suitable job title and, without being aware of the underlying coding, will automatically assign a 4-digit SOC code to the selected job (figure 4). The corresponding job-history timeline is displayed in figure 5.
OSCAR administration and validation
OSCAR was administered by email to all UK Biobank participants with an available email address (N=324 653) between June and September 2015; reminder emails were sent to partial- and non-responders up until December 2015. If needed, participants could telephone UK Biobank’s Participant Resource Centre and access an online set of frequently asked questions.
Among the verified and complete responses only, we used the freetext job title provided by each participant to perform an internal validation of OSCAR. An expert occupational coder, who was blind to the OSCAR SOC coding and health status of participants, manually coded a random sample of 400 (based on power calculations) (8) job titles. The agreement between the expert- (reference) and OSCAR-derived SOC codes was measured by calculating the Cohen’s Kappa (κ) coefficient and bias-corrected 95% confidence intervals (95% CI). Cohen’s Kappa is considered a more robust quantitative measure of inter-rater agreement (or reliability) than simple % agreement calculation because it takes into account the agreement occurring by chance. The κ values of agreement are often interpreted as follows: >0.80 (very good), 0.61–0.80 (good), 0.41–0.60 (moderate), 0.21–0.40 (fair), and <0.2 (poor) (9). All analyses were performed using Stata version 14 (StataCorp LP, College Station, TX, USA).
Results
Administration and internal validation
Overall, 108 784 UK Biobank participants, aged 45–81 years (63 years on average) at time of questionnaire administration, fully completed OSCAR (response rate: 34%). The duration for completion was around 20 minutes [median 17 (interquartile range: 11–30) minutes]. Participants included, on average, three jobs throughout their job history (up to a maximum of 40).
The agreement between the 4-digit SOC codes assigned by OSCAR and the manual coder was moderately good (κ=0.45, 95% CI 0.42–0.49). OSCAR performance improved, as expected, when considering coding agreement between broader job categories. In particular, the overall agreement increased at the 3-digit level to 52% (κ=0.52, 95% CI 0.49–0.54), at the 2-digit level to 62% (κ=0.62, 95% CI 0.59–0.63), and at the 1-digit level to 64% (κ=0.64, 95% CI 0.61–0.69). We tried to estimate the κ for each of the 353 4-digit SOC codes, but the few observations within each SOC code in our sample of 400 job titles prevented computing reliable estimates; the same applies at the 3-digit level. At the 2-digit level, considering SOC codes with ≥5 observations, the highest agreement was for the “54-” job groups (κ=0.76, 95% CI 0.54–1.00) corresponding in the original SOC classification to “textiles, printing and other skilled trades”, and the lowest for the “52-” (κ=0.11, 95% CI 0.08–0.19) corresponding to the SOC “metal machining, fitting and instrument making trades”. At the broader 1-digit level, the highest agreement was for the “2-” job-groups (κ=0.58, 95% CI 0.57–0.63) corresponding to the SOC “professional occupations”, and the lowest for the “1-” (κ=0.26, 95% CI 0.14–0.39), corresponding to “managers and senior officials”. Of note, while OSCAR assigned a 4-digit SOC code to all the job titles reported by the participants, the manual coder was unable to code two jobs due to lack of sufficient information in the job title input by the participant. In some cases, OSCAR codes appeared more specific than those obtained through manual coding. For example, the job title “research scientist” was coded as the generic 4-digit SOC “2321” by the manual coder but by OSCAR as “2113” corresponding to the more specific job title “geophysicist”.
Discussion
OSCAR is an innovative web-based tool that combines the efficiency of computer-based automatic software with the validity of standard occupational coding in order to ensure fast and reasonably reliable collection and coding of individual job histories.
The response rate to OSCAR was 34%, which is similar to previous questionnaires administered to the UK Biobank cohort (eg, 33% for the dietary 24-hour recall questionnaire) and was completed within the target time of 20 minutes. In contrast, manual coding of a subset of 400 job titles took approximately 16 hours, emphasizing the efficiency of OSCAR and its application in large population-based studies where alternative occupational exposure approaches would be unfeasible. The number of jobs included by each participant also suggests the completeness of the job-history collection process; in addition, the automatic coding method ensures no missing data in the coding phase.
The internal validation supports the reasonable reliability of this method. In the absence of an agreed gold standard, OSCAR has shown moderately good agreement with the expert manual coding approach that is traditionally considered the most reliable method. Such comparisons are, however, difficult to interpret, not only because of OSCAR’s original approach, but also because the reported inter-rater agreement of available occupational exposure methods is quite broad, ranging from very poor to moderate (2, 3, 10). Moreover, the innovative, underlying concept of relying directly on a workers’ ability to self-collect and code their own job history, allows a level of information accuracy otherwise impossible with alternative methods (including expert manual coders) and suggests that, after further validation, OSCAR itself could become a potential new reference coder. When compared with occupational records, self-reported job histories have been previously shown to be reliable (11) and repeatable (12–14). What is novel about OSCAR is that workers themselves, when supported by easy and quick web-based tools, can direct their entire occupational assessment process in a more efficient and possibly more accurate way than that available through costly, trained interviewers and manual coders.
We developed the underlying decision tree of OSCAR to be flexible and adaptable to any research project. The web-based interface makes it easily accessible across many different personal devices and negates the need to install or download any special software. The inclusion/exclusion criteria for jobs collection can be amended if needed or additional submodules can be added to fit specific research goals (eg, studies on breast cancer risk among healthcare workers). In addition, the collected 4-digit SOC codes can be linked to job-exposure matrices to automatically translate each job title into potential exposure to specific occupational agents. Lastly, compatibility with smart devices can help maximize response rates among participants.
Some limitations should be acknowledged. First, in our random sample of 400 job titles, the validation of OSCAR both quantitatively and qualitatively could not cover all the 353 SOC codes, but there is no a priori reason to expect that certain categories of workers would be systematically better or worse than others in finding their jobs among the groupings displayed by OSCAR and that the performance of OSCAR should be biased towards or against specific occupations. Second, the level of detail of the 4-digit SOC codes means that some jobs occurring in a general population are not included, but this a caveat of all existing standard occupational classification systems. However, because detailed job titles are usually grouped into broader job categories for statistical analysis, this is not, in itself, a problem. Third, it is impossible to rule out some residual job misclassification, but this is likely to be non-differential with regard to health status not only because participants were not aware of the specific research health outcome, but also because information on jobs rather than specific occupational exposures were collected (3). Finally, OSCAR uses a UK-based standard classification, but SOC codes can be easily mapped onto other national and international classifications [eg, to ISCO-88(15)] by using Excel worksheets developed by the ONS Classifications and Harmonization Unit, and available at the following websites (http://webarchive.nationalarchives.gov.uk/20160105160709/http://www.ons.gov.uk/ons/guide-method/classifications/archived-standard-classifications/standard-occupational-classification-2000/index.html).
In conclusion, OSCAR is a novel, efficient and reasonably reliable web-based tool for collecting and automatically coding lifetime job histories in large population-based epidemiological studies. Further application of OSCAR in a broad variety of research studies and study populations is warranted to validate this efficient occupational assessment tool externally.