In the construction industry, the reported burden of occupational traumatic injuries is substantial and exceeds the burden for other industrial sectors, both in the frequency of reported events as well in the mean workers’ compensation costs (1). Considerable progress has been made in identifying factors that contribute to injury risk, addressing barriers to injury prevention and designing interventions to reduce the risk of traumatic injuries in construction (1–8). However, injury rates remain high. Reducing construction worker injuries continues to be a top priority for occupational health research, for example as articulated in the United States National Institute for Occupational Safety and Health (NIOSH) National Occupational Research Agenda (NORA) for construction (9). A better understanding of additional factors that contribute to injury risk may ultimately inform the development of more effective injury prevention efforts.
Occupational heat exposure has long been understood to increase the risk of heat-related illnesses (HRI) such as heat stroke, which can be fatal (10) and may also reduce worker productivity (11–13). The relationship between heat exposure and other adverse health outcomes, such as traumatic injuries, has received minimal attention until recently; however, a positive association is evident in the literature (14–21). For example, a traumatic injury odds ratio (OR) of 1.006 [95% confidence interval (CI) 1.002–1.011] per one °C increase in maximum daily temperature for the construction industry has been reported in Australia (14).
The potential for heat exposure in construction workers is substantial. Exposure to heat, where heat is defined as energy transfer to and from the human body, includes exposure from environmental (or ambient) conditions, metabolic heat production, and the insulating properties of clothing or other near-skin barriers (22–25). Construction workers may be subject to high outdoor temperatures with or without solar radiation and task-related point sources of heat, high metabolic demands, and personal protective equipment (including clothing) that place them at high risk for heat stress. In Washington State (WA), Bonauto et al (26) reported that between 1995–2005 the construction industry, compared to other industries, experienced the highest workers’ compensation incidence rate for HRI at 12.1 per 100 000 full-time equivalent (FTE).
The mechanisms through which heat may contribute to the risk of a traumatic injury are still under investigation. Research in exercise, human physiology, and occupational settings report heat-related changes in cognitive performance (27, 28) and psychomotor vigilance (29) – critical functions that, when impaired, have been documented to compromise balance, mental status, and response time after exercise or in conditions of hyperthermia (29–32). These factors have in turn been linked to injury risk in occupational settings (6, 27, 33). Other factors associated with heat stress, such as muscle fatigue or cramping and dehydration, are also shown to negatively affect performance, particularly when experienced in conjunction with one another (32, 33). Inadequate acclimatization, which can be influenced by work organization and acclimatization training, may also influence injury risk in the heat (34) and unsafe work behaviors, although it is unclear whether this finding is related to cognitive performance effects or behavioral factors such as irritability under heat stress conditions (35). Further research in needed to elucidate the role of these factors in the development of heat-related injuries.
While the existing literature has contributed to the understanding of heat and injury risk, published research to date has primarily assessed the effect of factors such as age, gender, mechanism of injury (16), lagged exposure (16), and business size (14) on injury risk in the heat for all industries combined. In addition, the existing literature has predominantly relied on representative weather monitoring stations that may not adequately measure regional patterns in climate or differences between microclimates. Yet each industry has substantial differences in working population characteristics, heat exposures, and other injury risk factors. Gaps exist for construction industry-specific analyses, which have yet to describe how factors such as age, business size, mechanism of injury, time of day, and work experience affect heat-related injury risk.
The purpose of this study was to assess the relationship between outdoor apparent temperature and traumatic occupational injuries in Washington’s outdoor construction workers. This study adds to the existing literature though the use of high-resolution meteorological data, methods for identifying outdoor occupations, and exploration of factors that may modify the effect of heat on injuries in this population, with the ultimate aim of informing heat-related traumatic injury prevention efforts.
Methods
We assessed the relationship between maximum daily humidex, a measure of apparent temperature, and occupational injuries in outdoor construction workers using a case-crossover design with time-stratified referent selection and linear splines. We obtained occupational injury data through the WA State Fund workers’ compensation system and spatiotemporally paired these data with high-resolution meteorological data.
Injuries and case definition
The outcome dataset included all accepted WA Fund workers’ compensation injury claims, as described in the appendix (www.sjweh.fi/show_abstract.php?abstract_id=3814), with injury dates between 2000–2012. This dataset included variables pertaining to the injury location, worker demographics, work and employer characteristics, and injury and claim characteristics. We defined cases as repeat or first occurrence traumatic injuries occurring in adult (≥18 years old) outdoor construction workers (see figure 1 and appendix).
Figure 1
Injury claim case definition with the number of claims meeting the criteria. [NAICS=North American Industry Classification System; SIC=Standard Industrial Classification; SOC=Standard Occupation Classification; O*NET=Occupational Information Network; FIPS=Federal Information Processing Standard; WMSD=work-related musculoskeletal disorder.]
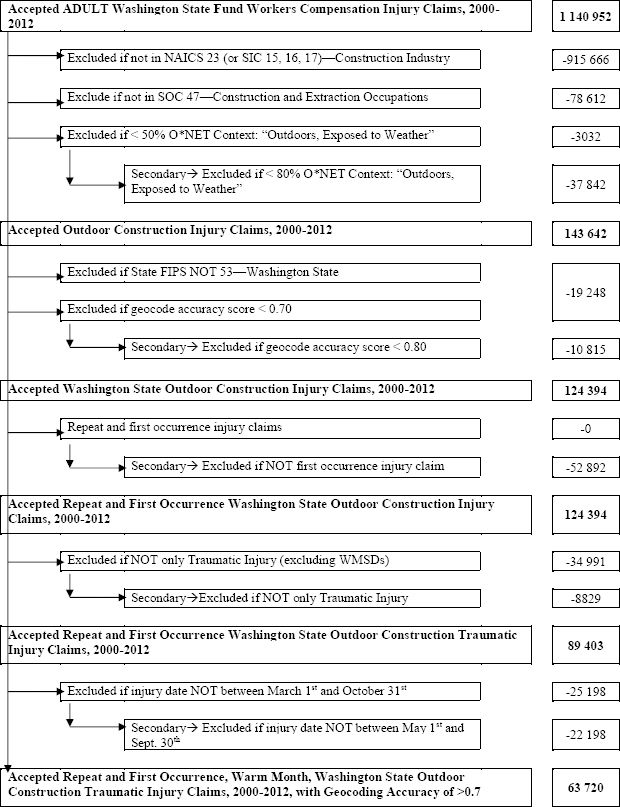
Referent selection
We used time-stratified referent selection to select referent dates (36). Referent dates were selected as days in the calendar month in which the injury occurred, on the same day of the week, excluding major holidays. This approach controls for seasonality and day of the week, respectively. The severity of the injury may influence individuals’ at-risk time periods in the case of fatalities or where claimants are removed from work to recover from the injury. Including referent dates that fall after the occurrence of an outcome may introduce bias, but for rare events the bias introduced by the inclusion of these referent dates is less than the bias introduced by alternatives to the time-stratified referent selection design, such as unidirectional sampling (37). Referent dates after the date of injury were therefore included.
Heat exposure
Meteorological data on a ~1/16th resolution grid (4 km × 7.5 km) were produced using the Parameter-elevation Relationships of Independent Slopes Model (PRISM) (38–42) (see appendix). Exposure was defined as the maximum daily humidex, which is a function of heat index and dewpoint (43), at the grid point of the closest Euclidean distance to the location at which the injury occurred. As heat stress includes both ambient heat exposure and internal metabolic heat generation, estimates of metabolic equivalent (MET) values from the Compendium of Physical Activities and American Time Use Survey (ATUS) by occupation were assigned to each injury claim. These data are provided by the National Cancer Institute (44, 45) and have been used in other occupational health research (46). MET data were not combined in this analysis with ambient heat exposure but rather were used as a separate variable in stratified analyses. MET values range from 1–16, where 1 MET is defined as the average adult resting metabolic consumption rate of 3.5 ml O2/kg body weight/minute, or 1 kcal/kg/hour. Based on studies using calorimetry, light physical activity, such as standing or walking slowly, corresponds to <3.0 MET, moderate physical activity, such as bicycling or weight lifting, corresponds to 3.0–6.0 MET, and vigorous activity, such as running, corresponds to >6.0 MET.
Geocoding and spatial pairing
We assigned one address to each injury claim, as described in the appendix. Spatial joining of the assigned injury coordinates with the meteorological grid point coordinates was executed in ESRI ArcGIS (47) using the nearest neighbor function for a point-to-point join. Since we restricted the case definition to include only claims assigned a WA address and the meteorological data cover a large region of the Pacific Northwest, including the entire State of Washington, the Euclidian distance between the location of the injury and the exposure grid point may have exceeded one-grid cell only in rare cases along coastal water. For each joined coordinate pair, the corresponding meteorological data were then determined for all dates associated with the injury claim (injury date and referent dates) and for one-day prior to the injury and referent dates.
Analyses
We compared exposures on injury dates at injury locations to exposures on referent dates at the same location. Analyses were performed for the warmer months of the year, March 1st – October 31st, within WA. This time period was selected to be inclusive of calendar days where temperatures exceeded the 95th percentile of full-year maximum daily humidex for all of WA (34.1 °C) and to exclude cold weather and cold weather-related injury risk factors, such as slippery surfaces due to ice and decreased dexterity from cold temperatures or extra clothing.
Descriptive statistics were calculated for worker demographics, work and employer characteristics, injury and claim characteristics, and exposures on injury and referent days. The variability in exposures on injury and referent days within each worker (ie, stratum) was described as the square root of the mean of the within stratum variances.
We assessed the relationship between maximum daily humidex and traumatic injuries using conditional logistic regression implemented with the clogit function from the survival package (48, 49) in R (R Foundation, Vienna, Austria) (50). Based on previous studies, we anticipated a non-linear effect (14, 15) and selected three knots a priori (25, 29, and 34 °C humidex) to construct linear splines. This allowed us to model potential differences in the relationship between heat and traumatic injuries between the selected knots. The lower knot was selected as the Canadian Center for Occupational Health and Safety’s (CCOHS) lowest humidex threshold in guidance aimed at reducing the risk of heat-related illness (25 °C) (43). The upper knot was selected as the value identified by Spector et al (15) as an inflection point in the relationship between humidex and traumatic injury risk in agricultural workers in Washington State (34 °C). The middle knot was selected as the midpoint between the upper and lower knots. Model fit was explored for other CCOHS recommended thresholds and lower humidex values using the Akaike Information Criterion (AIC). Based on model fit, the knots were adjusted to 21, 25, and 37 °C humidex. Results using the best-fit knots are reported alongside those using the a priori knots.
Secondary analyses were conducted for dry temperature, a one-day lag in exposure, continuous exposure (no splines), and categorical exposure (17–20, 21–24, 25–29, 30–34, 35–39, and ≥40 °C) compared to a reference category (<17 °C). Knots for the spline analysis using dry temperature were set as the mean of all temperature values for a given humidex knot: 19.3, 22.7, and 32.2 °C dry temperature. We conducted stratified analyses by climate region (eastern versus western WA), size of the employer (≤10, 10–49, and ≥50 FTE), time of day of injury (5:31–9:30, 9:31–12:30, 12:31–16:30, and 16:31–19:30), age of the worker (18–24, 25–34, 35–44, 45–54, and ≥55 years old), MET level of occupation (<4, 4–5, and >5), experience [more versus less, where more experience was defined as either working in the previous quarter based on employment security data (ESD) data or self-report of working for the employer ≥90 days], Occupational Injury and Illness Classification System (OIICS) event (falls, bodily reaction and exertion, and other), and body part injured (upper extremity, lower extremity, trunk, and multiple body parts).
Sensitivity analyses were conducted to evaluate the robustness of our results. Sensitivity analyses included claims with occupations more likely to involve outdoor work (≥80% outdoor context, as described in the appendix), geocoding accuracy score ≥8.0 (see also appendix), May 1st through September 30th claims, claims assigned the accident address only (versus the first medical provider or employer address, as described in the appendix), claims with <2 days between the injury and first medical provider visit, only new, first occurrence injury claims, and claims with less than seven days of time loss, as described in the appendix.
The WA Institutional Review Board reviewed the study protocol and determined the study to be exempt.
Results
Worker demographics and injury claims
Of the 225 286 claims in the construction industry WA State Fund workers’ compensation system from 2000–2012, 63 720 met the case definition (see figure 1). Of these claims, 97.9% were male, with a mean age of 34 years old and mean body mass index (BMI) of 27.2 kg/m2 (Table 1). The most common North American Industrial Classification System (NAICS) sub-sector was “specialty trades contractors” (67.1%) followed by “construction of buildings-residential” (18.1%). Most claims were classified in SOC minor group “construction trades” (85.4%). The majority of claimants (80%) were considered to have more experience (as defined above), and slightly more claims were classified under small employers (<10 FTE, 36.2%) than large employers (≥50, 24.1%). Occupations with MET >5 included carpenters (SOC 472031; MET=6), construction craft laborers (SOC 472061; MET=6), and structural iron and steel occupations (SOC 472221; MET=7.5); these claims comprised 46.6% of claimants.
Most injuries tended to occur during daytime business hours of 05:30–16:30 (94.2%), Monday–Friday (95.9%), in Western WA (79.9%). The accident address was assigned to 50.8% of claims as the location at which the injury occurred, followed by 47.3% assigned the address of the first medical provider. The most commonly injured body parts were the upper extremities (57.4%), and injuries were most often the result of bodily reaction and exertion (43.6%) or falls (33.5%). Most claims did not involve time loss (77.2%), however 328 (0.5%) claims resulted in total permanent disability, and there were 31 fatalities.
Table 1
Injury claim descriptive statistics (N=63 720). [SD=standard deviation.]
Exposure
For the March–October period, the mean [interquartile range (IQR)] humidex on injury and referent days was 21.6 (IQR 15.6–26.9) °C and 21.4 (IQR 15.5–26.8) °C, respectively (Table 2). The mean of within strata (injury and corresponding referent days) standard deviations was 4.3 °C. For the May–September period, the mean humidex on injury and referent days was 25.4 (IQR 21.1–29.4) °C and 25.3 (IQR 21.0–29.3) °C, respectively. There was a mean of 3.4 referent days per injury day. Eastern WA tended to be characterized by a slightly higher mean humidex and within strata standard deviation than Western WA.
Table 2
Maximum daily humidex by warm season span, geographic region, and number of strata or days in each humidex category. [IQR=interquartile range; WA=Washington State.]
N | Mean | IQR | Number of strata or days containing humidex category | |||
---|---|---|---|---|---|---|
|
||||||
Strata | Injury days | Referent days | ||||
March‒October a | ||||||
Injury days | 63 720 | 21.6 | 15.6‒26.9 | |||
Referent days | 218 239 | 21.4 | 15.5‒26.8 | |||
Humidex categories | ||||||
<17 | 33 119 | 19 344 | 67 209 | |||
17‒20 | 34 485 | 10 702 | 36 913 | |||
21‒24 | 38 152 | 12 326 | 41 854 | |||
25‒29 | 33 888 | 11 910 | 40 816 | |||
30‒34 | 20 605 | 6198 | 20 723 | |||
35‒39 | 9288 | 2368 | 8121 | |||
≥40 | 3327 | 869 | 2593 | |||
May‒September b | ||||||
Injury days | 41 522 | 25.4 | 21.1‒29.4 | |||
Referent days | 142 251 | 25.3 | 21.0‒29.3 | |||
Humidex categories | ||||||
<17 | 11 186 | 3720 | 12 910 | |||
17–20 | 21 260 | 6561 | 22 966 | |||
21–24 | 30 922 | 10 479 | 35 991 | |||
25–29 | 31 527 | 11 398 | 39 183 | |||
30–34 | 20 248 | 6127 | 20 488 | |||
35–39 | 9277 | 2366 | 8114 | |||
≥40 | 3327 | 869 | 2593 | |||
Western WA c | ||||||
Injury days | 50 779 | 21.2 | 15.6‒26.3 | |||
Referent days | 173 972 | 21.0 | 15.6‒26.3 | |||
Eastern WA d | ||||||
Injury days | 12 941 | 23.1 | 15.6‒30.6 | |||
Referent days | 44 267 | 23.1 | 15.4‒30.7 |
Inferential analysis
The OR and 95% CI for the association between humidex and traumatic injuries in adult, outdoor construction workers from March–October in Washington are presented in Table 3. For both the a priori and best-fit spline analyses as well as the 6-group exposure category analyses, we observed higher OR for a traumatic injury with greater heat exposure. In the best-fit spline analysis, we observed an OR of 1.003 (95% CI 1.000–1.007), 1.0087 (95% CI 1.000–1.017), 1.0045 (95% CI 1.000–1.009), and 1.0131 (95% CI 0.999–1.028) per one °C change in humidex <21, 21–25, 25–37, and >37 °C. Using continuous exposure and no splines, the OR was 1.005 (95% CI 1.003–1.007) per one °C change in humidex. The OR using dry temperature were highest for moderate and high exposure categories. We observed an overall increasing trend in the association between a 1-day lag in heat exposure and traumatic injuries, however the spline model suggests some nonlinearity in the trend with a fairly high increased risk <21 °C as well as from 25–37 °C, but a decreasing risk between these two exposure strata from 21 to 25 °C.
Table 3
Estimated odds of traumatic injury for a one degree increase in exposure (a priori and best fit spline models) and a one degree increase or categorical exposure (secondary analyses). N=281 946, number of events= 63 717. [OR=odds ratio; CI=confidence interval]
Stratified and sensitivity analyses results using the continuous humidex model are depicted in figure 2. We used the continuous humidex model for comparison of the stratified and sensitivity strata because of the near-linear trend in the spline analyses and limited sample size resulting from some case definition and exposure combinations. Overall, there was considerable overlap in the CI across categories. Analysis by age category revealed a higher OR for younger (18–24-year-old) and older (>54 years) claimants. Lower extremity injury claims were also characterized by a higher OR as were those associated with less worker experience. Lower OR were observed for injuries occurring later in the afternoon and for the large employer size (≥50 FTE). The OR for Eastern WA was slightly lower than for Western WA. Sensitivity analyses did not result in substantially different relationships between humidex and traumatic injuries.
Discussion
Our findings of a positive association between occupational heat exposure and the risk of occupational traumatic injuries in WA outdoor construction contributes to the growing body of evidence suggesting occupational heat exposure impacts the health and safety of workers beyond HRI. Our results of potentially higher risk in younger and older workers, workers with lower extremity injuries, workers with less job experience, smaller employers, workers working in Western WA, and time of injury before 12:30 hours provide opportunities for further study of the nuances of this phenomenon in outdoor construction workers. A better understanding of these nuances could lay the groundwork for construction injury prevention approaches that address the role heat plays in the risk of an injury.
In the best-fit spline analysis, increasing humidex during warmer months of the year was characterized by a nearly linear association with the risk of a traumatic injury. A similar trend in effect estimates was seen in the a priori spline, continuous, and categorical exposure analyses. This positive relationship is consistent with findings in the existing literature. Xiang et al (14)reported an injury incidence rate ratio (IRR) of 1.006 (95% CI 1.002–1.011) per °C increase in maximum daily temperature between 14.2–37.7 °C for construction workers in Adelaide, Australia (14). This study is similar to our study in that the association between workers’ compensation injury claims and heat exposure was assessed for non-linearity using splines and similar stratified analyses were performed. However, the exposure data in the Xiang et al study were sourced from a representative weather station, the region is characterized by a hotter climate, and the reliance on industry-level data to identify outdoor workers likely includes some predominantly indoor occupations grouped with outdoor workers. In addition, the Xiang et al study did not use a case-crossover design. Instead, workers’ compensation data were transformed into a time series format and merged with meteorological data, and the association of temperature with daily workers’ injury claims was assessed using generalized estimating equations. The increase in risk reported by Xiang et al is on the same order of magnitude as the OR reported in this study for the continuous analyses using maximum daily humidex (OR 1.005 per °C; 95% CI 1.003–1.007) and maximum daily temperature (1.007 per °C; 95% CI 1.005–1.009). Similarly, Adam-Poupart et al (16) reported an IRR of 1.003 (95% CI 1.000–1.006) per °C increase in maximum daily temperature in Quebec, Canada for construction workers, and McInnes et al (21) reported an OR of 1.008 (95% CI 1.001–1.015) per °C increase in maximum daily temperature in young workers (<25 years of age) in Melbourne, Australia. Using wet bulb globe temperature (WBGT) as the metric for exposure, Garzon-Villalba et al’s study of acute injuries in Deep Water Horizon disaster cleanup workers resulted in a relative risk (RR) for acute injuries of 1.13 (95% CI 1.09–1.17) above a maximum WBGT of 20°C (20).
In a study of agricultural workers in Eastern WA using a categorical exposure approach, Spector et al (15) reported a peak OR of 1.15 for a maximum daily humidex between 30–33°C, relative to < 25°C. This estimate is higher than the OR reported for a similar range in exposure in this study (OR 1.09 for a maximum daily humidex between 30–34 °C, relative to <17 °C). The higher OR in agriculture at lower humidex values may reflect differences in safety culture, task-related hazards that are not characterizable with the available data, or reliance on a piece-rate pay structure, which is more common in agricultural work and has been reported to be associated with a greater risk of HRI and heat strain symptoms compared to hourly-payment methods (51).
Consistent with McInnes et al (21) and Adam-Poupart et al (16), who report linear relationships between increasing heat and increasing injury risk, we report a near-linear relationship, with only slight non-linearity observed in the best-fit spline analysis and categorical exposure analysis. In the analysis using splines, we report greater per °C increases in the OR at humidex between 21–25 °C and >37 °C. The exposures associated with these steeper slopes are noteworthy because they include humidex values in the range of 21–25 °C, which are currently considered to be comfortable and not deemed high enough to recommended prevention actions (52).
The near-linear trend in our results is, however, contrary to the findings of Spector et al (15), Xiang et al (14), and Morabito et al (19), who report a reversed u-shaped association between increasing heat and the risk of injury. While heat exposure metrics and populations vary by study, injury risk has been reported to decline above a maximum daily temperature of 37.7 °C (14), maximum daily apparent temperature of 31.7 °C (19), and maximum daily humidex of 34 °C (15). It has been hypothesized that the reversal of effects at the upper extremes of exposures are not the result of a true reduction in risk at high temperatures, but rather reflect exposure misclassification related to risk reduction practices used to prevent HRI, such as ending work shifts early on the hottest days. Work organization and behavior is challenging to characterize using population data, such as workers’ compensation claims, emergency department visits, or hospitalizations and may lead to exposure misclassification. These factors, however, may be more accurately characterized in workplace studies. Fogleman et al (53) used aluminum smelter company health and safety records combined with hourly weather data to assess the relationship between heat and injuries in aluminum smelter workers. In Fogleman et al’s study, where work hours and hourly weather data were known, elevated acute injury OR were observed above exposures of 32 °C.
Unlike in agriculture, there may be less flexibility to modify work hours to avoid working during the hottest part of the day in construction. For example, noise and light ordinances may prohibit construction activities outside of typical business hours. The pace of work may also be driven by pressure to complete a task due to weather or material considerations, putting workers in a position where they are unable to adequately self-pace or practice recommended work-rest cycles unless encouraged by their employer. In addition, adjustment of work processes so that easier or less strenuous tasks can be completed during periods of high heat stress, when workers often experience greater fatigue and productivity is expected to wane, may be unattainable due to scheduling constraints and the nature of the tasks needing to be completed. Workers often need to work within local regulations and accommodate the schedule of other trades.
Results of stratified analyses showed little statistically significant difference between strata, however they may still provide insight into potential demographic and industry-specific modifiers of the effect of heat on injury risk. Large employers (≥50 FTE) were characterized by a lower injury effect estimate in stratified analyses than medium and smaller employers. This finding is consistent with findings by Xiang et al for large (≥200 FTE), medium (20–199 FTE), and small employers (1–19 FTE) (RR 1.000, 1.004, and 1.007, respectively) (14). Larger employers are required to have safety committees in WA (WAC 296-800-130) (54) and may have greater capacity to implement heat risk prevention strategies, utilize health and safety technology, and employ dedicated health and safety specialists. There are also trends in employer size by other industry characteristics that may result in different occupation- or task-related risk factors by employer size. For example, construction in residential and commercial sectors more commonly involves smaller and larger companies, respectively (4). Additional support for small businesses may be indicated to effectively prevent heat-related traumatic injuries.
Age is a known risk factor for heat-related health effects. We report that younger (18–24 years of age) and older (>54 years) claimants exhibited greater heat-related injury effect estimates. In a setting with high metabolic demand and high ambient heat, older individuals may be more susceptible to the effects of heat due to decreased skin blood flow (55) and decreasing cardiac capacity (56), as well as have a higher prevalence of pre-existing conditions. Young workers may be more likely to exert rather than pace themselves, may be more likely to be assigned tasks with higher metabolic demands than their older counterparts, and have been reported to display more negative attitudes towards safety and personal protective equipment (7, 57). In other similar research, higher risk estimates have been reported for workers <25 years of age per °C increase in maximum daily temperature in Australia (21) and in Canada (16). None of these studies report higher risks for older workers, except for McInnes et al (21), by minimum daily temperature.
Less experience was also characterized by a higher injury effect estimate. Our definition of experience was limited to working for the employer in the previous quarter or a self-reported ≥90 days and does not describe experience acquired over their tenure of employment within the industry that could influence a worker’s tasks, responsibilities, standing with an employer, or perceived job security. However, this definition is expected to reflect familiarity with factors that are important for adequately preparing workers for the conditions present on a job site, such as processes and tasks, as well as acclimatization to environmental conditions (34). We observed slightly less experience in claimants age 18–24 and slightly more experience in claimants >54 years of age, with 73% and 84% of each respective age group categorized as having more experience compared with 80% for the full population.
Regional differences in effect are likely the result of differences in climate. WA’s climate is characterized by two distinct regions: a relatively milder western region and an eastern region with hotter, drier summers and colder winters. These different climates may affect patterns of acclimatization (timing and threshold tolerance) and could trigger differences in heat-related practices, which were not captured in our study.
We observed higher OR for injuries to the lower extremities than other parts of the body. Of these injuries, the most common precipitating event was falls (48%), whereas in the full dataset, falls accounted for 34% of injuries. Research investigating potential mechanisms of the relationship between heat and traumatic injuries has focused on decrements in balance [related to extreme muscle fatigue (58) and exercise in hot conditions (32)] and cognitive performance (28, 29), including vigilance, and conditions characterized by heat stress. These changes could plausibly increase the risk of falls, for example from heights. Other factors may also contribute to the relationship between heat and traumatic injuries, such as changes in safe work practices (35).
There are a number of potential explanations for higher effect estimates in earlier work-shift hours that should be explored further. Tasks may differ by time of day, and differences in tasks could affect metabolic contribution to heat stress as well as traditional risk factors for injuries (eg, trip hazards). Notably, we did not find evidence suggestive of effect modification by MET level, indicating values provided at an occupational level may not describe the variability in metabolic demands across tasks or even individuals conducting similar work. Heat exposure in the morning may also have been low enough that workers did not recognize early signs of HRI or heat strain. These lower exposures would also likely not have triggered HRI awareness or intervention tactics. Diurnal patterns have also been observed in vigilance and balance research, where performance was worse in early morning tests. In field studies with agricultural workers in WA State, Spector et al (59) observed significantly longer mean reaction time and a greater number of lapses measured using a psychomotor vigilance test (PVT) as well as longer mean total path length, a measure of postural sway, in pre-shift assessments (ie, prior to a mean shift start time of 06:00 hours). Further research should investigate specific tasks and associated metabolic heat production throughout the work shift, construct task-related injury risk factor profiles by time of day, and better characterize vigilance and postural sway within workers over time and between workers.
Strengths and limitations
A strength of this study was our approach to identifying occupations more likely to work outside, which could minimize non-differential exposure misclassification and reduce the chance of bias of results towards the null. However, we were unable to verify whether tasks performed on the day of injury occurred outdoors. Further work is needed to better characterize the work environment by task, job site, or other factors that could improve categorization of indoor and outdoor contexts. An additional strength is the availability of both high-resolution meteorological data and injury location addresses, which enabled spatial pairing of the outdoor conditions with the injury location at a higher resolution than has been achieved in other studies of heat exposure and traumatic injuries in construction.
This study has several limitations. First, in nearly half of the claims, the accident address was either missing or not complete enough to be geocoded accurately. As a result, there may have been exposure misclassification from assigning the address of the first medical provider to the injury location. However, our sensitivity analyses suggest this did not substantially affect results. Second, we were unable to account for variability in clothing. Clothing is an important consideration when assessing heat stress since it can act as an insulating barrier between the body and the environment. WA workers’ compensation records do not contain systematic information about a claimant’s clothing at the time of injury. Third, we did not take into account solar radiation. In occupational settings, WBGT, which is a function of a measure of solar radiation (black globe temperature) and other factors, is often considered to be the gold standard for measuring environmental heat (25). In large studies such as this one, where a metric for clouds or solar radiation is not available in the meteorological data and there exists substantial spatiotemporal variability in weather conditions, use of existing methods to estimate WBGT (60) is challenging. We were also unable to take into account differences in worker microclimates influenced by point sources of heat, shade, or shift breaks. We assumed that referent days represented the distribution of exposure experienced by claimants on non-index days. Finally, we were unable to adjust for several potential unmeasured time-varying confounders, including task and worksite safety practices.