In recent years, various authors aiming to assess quantitatively the direct and indirect effect of low educational level on laryngeal cancer risk have mentioned the relationship between social inequality and laryngeal cancer. Conway and colleagues (1) calculated the percentage difference in odds ratios (OR) across sequential models including smoking, alcohol consumption, and diet and found that 67% of the excess risk for those with low education was explained by the above behaviors. This suggests that low socioeconomic status (SES) confers risk through pathways other than risky behavior. Two other studies quantitatively assessed the proportions attributable to lifestyle and occupational exposure adjusted for sequentially included covariates. Menvielle et al (2) explained about two-thirds of the differences observed for educational level by alcohol and tobacco consumption, varying according to tumor site. Occupational exposure explained about 50% of the remaining inequalities. Boing and colleagues (3) explained 45% of the association between low education and disease by smoking and alcohol consumption. However, they used a different definition of low education (<4 years of school).
In these studies, the extent of the association between SES and laryngeal cancer risk was measured by the difference in the regression coefficients of the same variables across models with different control variables (1–3) using the attributable fraction (AF) (ORR-ORA)/(ORR-1), where ORR is the reference model’s OR and ORA is the adjusted model’s OR. However, according to Mood and others, coefficients from different nested models are not measured on the same scale in logit models and are, therefore, not directly comparable, a situation known as rescaling (4, 5). Moreover, Boing and colleagues (3) assessed the association of occupation and education using models where SES variables were derived from correlated dimensions. If education and occupational class are structurally linked, inclusion of either variable would result in a similar distribution of any specific outcome (6).
The aim of our study was to estimate the contribution of behavioral and occupational risk factors to laryngeal cancer and educational aspects using a decomposition method for logit models proposed by Karlson et al (7). This approach enables assessment of the effect of confounding without the influence of rescaling (ie, the logit coefficients are not measured on the same scale). When estimating models with nonlinear/binary outcomes, as in the logistic regression, rescaling the model after including additional covariates causes the error variance to change. Therefore, the interpretation of differences in coefficients between nested models is biased if the impact of rescaling is not taken into account (8). In our study, we used occupational indices that have, on the one hand, the ability to differentiate between physical-, psychosocial-, and carcinogenic-agent-dimensions of occupation (9). On the other hand, these indices summarize occupational information for all International Standard Classification of Occupations (ISCO)-coded jobs as valid job exposure matrices for many industrial sectors are not available and would not cover the range of 178 jobs (on a 3-digit ISCO-68 basis) considered in our study. The occupational dimensions are represented by recently developed occupational indices, which are simple to apply to occupational history data using standard ISCO coding. These indices might be able to explain a large part of the educational differences among laryngeal cancer patients.
Methods
This population-based, case–control study was conducted in Germany with 208 histologically confirmed male cases of laryngeal cancer (response rate 89.2%) between 1998– 2000. The study region covered a population of 2.7 million people in the Rhein-Neckar-Odenwald region of Southwest Germany. Cases were obtained from all relevant hospitals in the region (ie, the clinics of Heidelberg, Mannheim, Ludwigshafen, Darmstadt, and Heilbronn). Cases and controls were restricted to Germans aged ≥80 years who were registered as citizens in the study region. From the study population, 702 controls were randomly selected from the population registries of the study area and originally 1:3 frequency-matched to cases for age (response rate 62.4%). After checking the clinical-pathological records, 28 patients had to be excluded due to other diagnoses or recurrence of an earlier tumor. The ethical committee of the University of Heidelberg cleared the study and written consent was obtained from the participants through collaborating physicians.
Information on smoking, alcohol consumption, and occupational exposure was collected by well-trained interviewers using a comprehensive standardized questionnaire, which has been applied in almost identical form in previous large studies (10, 11).
SES was assessed in terms of education and grouped in three levels corresponding to the years of school attended according to the German educational system: ≤9 (low, “Hauptschule”), 10 (middle, “mittlere Reife”), and >10 [high, “(Fach-)Hochschulreife”] years.
Smoking data were assessed by lifetime smoking periods for which daily, weekly, and monthly tobacco consumption of cigarettes (rare use of cigars, cigarillos, and pipes was added corresponding to their average weight relative to that of cigarettes) and were used to calculate pack-years of smoking [ie, the cumulative number of cigarettes smoked (1 pack-year corresponds to 20 cigarettes/day for one year, equivalent to nearly 7300 cigarettes)]. Pack-years were included in multivariate analyses as a log-transformed continuous variable (Ln), which showed statistically the best model fit using the fractional polynomial technique (12). A categorical variable was used for descriptive purposes.
Alcohol consumption was calculated from the reported daily, weekly, or monthly alcohol consumption ten years before interview for all common alcoholic beverages, assuming the following ethanol content: beer 5%, wine, fruit or sparkling wine 10%, aperitif and liquors 20%, and spirits 40%. Average daily consumption (g ethanol/day) was included as an untransformed continuous variable, again following the fractional polynomial technique.
A detailed lifetime occupational history section collected data on every occupation since the point in time when people started working, including working period, job title, industry, and nature of work. Each job title was coded corresponding to the ISCO and converted from ISCO-68 to ISCO-88 (13) as the latter served as a basis for the application of the previously published occupational indices used in our study (9).
The indices were constructed with data from a representative study of the working conditions of 20 000 employees in Germany in 2006 and applied in a study on mortality related to SES (14). Five different working dimensions were used as a basis for the indices: ergonomic stress, environmental pollution, mental stress, social stress, and temporal loads. The overall job index is defined as the sum of all these dimensions. A physical job index was constructed using ergonomic stress and environmental pollution only, whereas the psychosocial index includes mental and social stress and temporal load. An additional index defined as the carcinogenic agent index (CAI), considering only jobs with a likely exposure to smoke, dust, gases, and vapors, was extracted from the environmental pollution dimension. Every job category coded according to ISCO-88 is represented in the index: 100% of all 2-digit ISCO-88 codes, 94.8% of the 3-digit codes, and 78.5% of the 4-digit codes can be matched to the index (9). An index is based on the random intercept of a 4-level hierarchical linear regression model, with levels defined by the 3-digit codes of ISCO-88 and the respondents of the survey. It is controlled for respondent characteristics such as age, gender, working hours, and experience on the job and can therefore be considered objective. For each of the four indices, a separate model was estimated and a best linear unbiased prediction for random intercept on the ISCO-88 digit levels was performed (15). In our study, the indices were first matchde to all jobs mentioned in the lifetime job history. The linkage between jobs and CAI was originally done on the 4-digit basis, as this better differentiates between the occupational burdens of various jobs. In order to improve readability and reduce job categories, the results are presented in 2-digit job categories thereafter. Thus, a 2-digit category might appear twice in the figure (ie, ISCO-88 code 83), but refer to different jobs (“earth-moving operators” having a high CAI, but “heavy truck, lorry, bus and tram drivers” having a slightly lower CAI). In the original construction of the index by Kroll (9), 39 items considering 5 dimensions of occupational burden were summarized using a hierarchical linear regression model, presented in ascending order, and finally divided into deciles. For every index, the values refer to those deciles in ascending order: jobs in index group one were among those with the lowest occupational burden (like draftsmen, bookkeepers and teachers), index group ten refers to a particularly heavily loaded group (such as miners, bricklayers, and metal and machinery workers) among all occupational groups.
The indices were categorized in four levels (9): “high” (9–10), “upper middle” (6–8), “lower middle” (3–5), and “low” (1–2) index values. For descriptive analysis, the indices were used as categorical variables and as ordinals for the main analysis. For logistic regression modeling, the average of all indices per person for all lifetime performed jobs was calculated (ie, a weighted index by years on the job).
For both cases and controls, percentage of years worked in each job was calculated relative to the sum of years of the lifetime occupational history. The jobs were then related (via ISCO-88) to their corresponding value of CAI, as described above. The percentages in figure 1 represent the sum of the percentages of all jobs falling into the different values of CAI. This method was chosen as a person might contribute to different categories of the index having worked in different jobs during his occupational history. For example, a bricklayer might have worked as a building electrician in later years, thus contributing to the “high” and “upper middle” category respectively.
OR and 95% confidence intervals (95% CI) were assessed by conditional logistic regression models conditioned on age (5-year age groups) (16). We performed a set of logistic regression models to compare the coefficients related to larynx cancer across nested models using the Karlson et al method [details for this method can be found elsewhere] (7). To compare the results with previous publications, the attributable fraction (AF) using either an additive [(ORR - ORA)/(ORR-1)]×100 or a multiplicative scale [ln(ORR)-ln(ORA)]/[ln(ORR)]×100 was used (17). First, we estimated the magnitude of association between education and laryngeal cancer, thus obtaining unadjusted OR (univariate model). Second, we added smoking and alcohol consumption to the model, which was thereafter used as a reference for the comparison with models including the different occupational indices: overall job index alone, the physical and psychosocial indices together, and finally CAI alone. All the analyses were performed in STATA 12 (StataCorp, College Station, TX, USA). We calculated the attenuation percentages between parameter estimates (β) in different logistic models as:
where x, y, and z denote education, outcome, and predictor variables respectively using the Karlson et al command (18). The bootstrap approach (19) was used to calculate the confidence intervals for the percentages.
Results
This study comprised 208 male cases and 702 controls, with an average age of 63 years. Cases were heavy smokers and drinkers in comparison to controls: 51.3% versus 17.1% smoked >40 pack years and 39.4% versus 17.1% drank >75 g ethanol/day. Low educational level was prevalent among both cases and controls: 87.0% and 62.1%, respectively, attended <10 years of school. A Cochran-Armitage trend test gave highly statistical evidence that the risk of laryngeal cancer increased when educational level decreased. More detailed results on these basic characteristics have been previously published (20, 21). Cases had a lifetime mean number of 3.6 jobs and total job duration of 40 years, compared to 3.3 jobs and 41 years worked among controls. In their lifetime occupational history, a majority of cases worked in construction, machine operation, and assembly in comparison to controls (67.2% versus 42.8%). Thus, this is clearly reflected in the distribution of CAI values: linking the occupational indices to the ISCO-88 codes, cases showed higher values in the distribution of all four indices than controls: 19.2% of cases were in the highest category of the overall job index compared to 9.4% of controls (table 1). This was much more pronounced for the CAI (40.2% versus 20.2%).
The number of years worked by cases (N=8440) and controls (N=28 894) reflect the matching ratio. Figure 1 shows the distribution of the index values for CAI and the corresponding job titles separately for cases and controls and for all jobs performed according to the lifetime job history. The percentage of years worked in jobs with higher index values (9–10) of CAI was statistically larger among cases (55.1%) than controls (33.8%), and vice versa in jobs with low values (10.1% versus 24.2%, respectively, for deciles 1–3). Jobs like bricklayers, carpenters, miners, mechanics, metal, and machinery workers (ISCO-88 groups: 71, 72, 81, and 82) contributed with a higher percentage to the higher CAI values. The percentages for the overall job index were similar but less pronounced than in CAI (data not shown).
Figure 1
Distribution of the Carcinogenic Agent Index (CAI) among cases and controls and the according job titles.
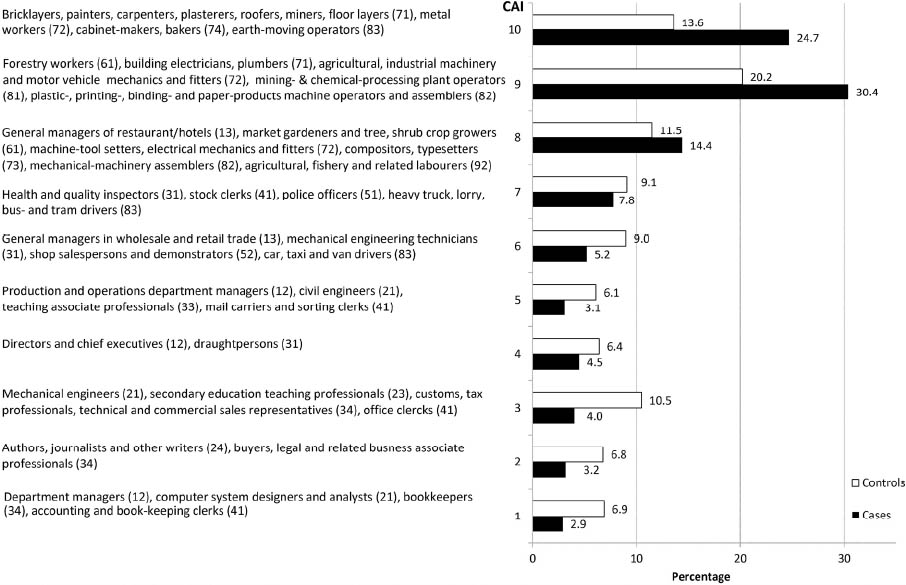
Controlling for age only, laryngeal cancer was strongly associated with a lower educational level, showing an elevated but not significantly increased risk for the middle level (table 2). For lower education, significant OR could be seen in all models using the different occupational indices, all adjusted for smoking and alcohol consumption. These OR were all of the same order of magnitude where the best model fit was given by the model containing CAI; the adjusted OR was 2.7 (95% CI 1.3–5.8).
Table 2
Relationship between low education attainment and laryngeal cancer risk by sequential models including smoking behavior, alcohol consumption, and occupational indices using the lifetime job history and the longest job. All models were stratified by age using 5-year intervals [CAI=carcinogenic agent index; Ln= log-transformed continuous variable; OR=odds ratio; 95% CI=confidence interval].
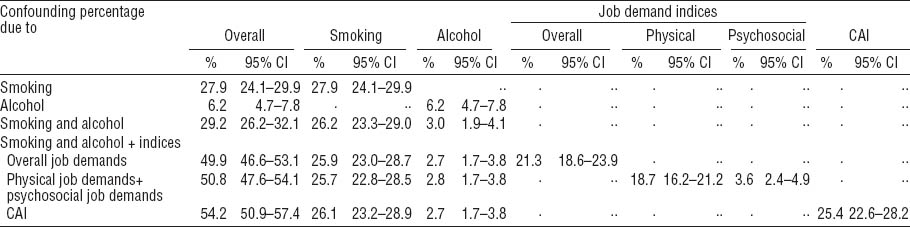
Following the Karlson et al approach, smoking and alcohol consumption together explained 29.2% (95% CI 26.2–32.1%) of the laryngeal cancer risk in the lower educational level, dominated by smoking accounting for 26.2% (95% CI 23.3–29.0%) of the total effect versus 3.0% (95% CI 1.9%–4.1%) for alcohol consumption. After adding the overall job index (based on the lifetime job history) to the decomposition, the confounding percentage (ie, the part explained by the covariates) increased to 49.9% (95% CI 46.6–53.1%). The effect was mainly mediated by smoking (25.9%, 95% CI 23.0–28.7%) and overall job index (21.3%, 95% CI 18.6–23.9%). A similar increase in the confounding percentage was observed when exchanging the overall job index with its components (the physical job and psychosocial indices) in the model (50.8%, 95% CI 47.6–54.1%). Here, a much higher percentage was observed for the physical (18.7%, 95% CI 16.2–21.2%) versus psychosocial job index (3.6%, 95% CI 2.4–4.9%). Finally including CAI in the adjusted model, the indirect effect accounted for 54.2% (95% CI 50.9–57.4%) with the CAI contributing 25.4% (95% CI 22.6–28.2%) (table 3). We obtained lower estimates than calculating the AF according to (ORR-ORA)/(ORR-1) (17). For the latter, smoking and alcohol consumption explained 31.5% of the low educational level associated with laryngeal cancer risk, overall job index 42.9% and CAI 51.4% of the remaining in the models when they were separately included. Alternatively, taking into account the non-linear relationship between OR and the probability of the outcome (18, 22, 23), the same formula on a multiplicative scale [(ln(ORR)-ln(ORA)]/[ln(ORR))×100] resulted in an estimate of 17% of the difference between high and low educational groups attributed to smoking and alcohol, whereas the estimates for overall job index was 27% and 34% for CAI. In total, occupational exposure and lifestyle factors together contributed to 66.7% of the observed educational inequality applying the AF formula on an additive scale and 45.1% on a multiplicative scale (data not shown).
Table 3
Proportion of low educational effect explained by smoking, alcohol consumption, and occupational indices for the lifetime job history calculated with the Karlson et al (7) method. [CAI=carcinogenic agent index; 95% CI=95% confidence interval].
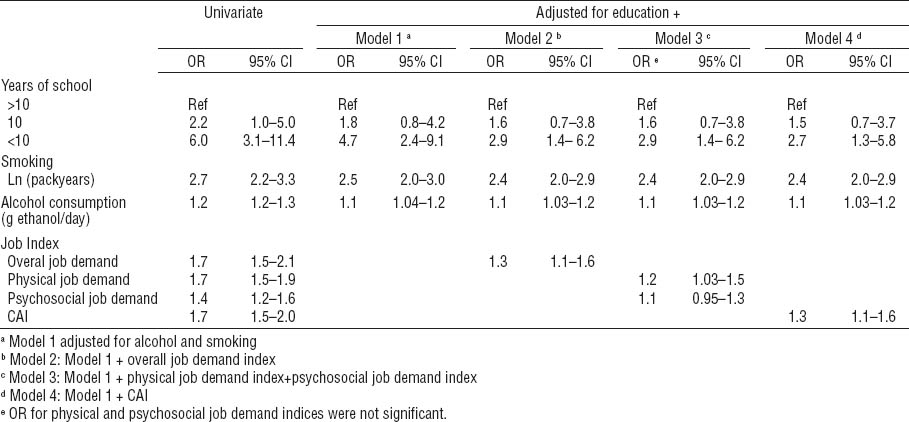
Discussion
This study confirms that the association between lower education and laryngeal cancer among males is mainly explained by smoking, alcohol consumption and occupation, where occupational exposures play an important role. We applied a recently published decomposition method for logit models proposed by Karlson et al (7) to calculate the percentage reduction in OR of education across nested models to estimate the degree to which the association between years of education and laryngeal cancer is mediated by the stepwise-added covariates. Smoking and alcohol consumption alone explained 29.2% of the inequalities attributed to the lowest educational level. Including various job-related indices reflecting different occupational burdens (9), another quarter of the association was explained by occupational exposures. The most promising way to explain educational inequality was to separate specific aspects of the index of environmental pollution (ie, exposure to smoke, dust, gases and vapors), which were summarized as the CAI. This CAI explained 25.4% (95% CI 22.6–28.3) of the total inequality after adjusting for smoking and alcohol consumption. Thus, occupational and lifestyle factors together contributed to 54.2% (95% CI 50.9–57.4) of the observed educational inequality. The residual percentage might partly be explained by other lifestyle factors. For example, second-hand smoking in private life and workplace might have been worse in the past years, when laws to ban smoking were less strict. Possible environmental pollution in residential areas might have affected low income workers. However, these factors are not enough to explain more than 40%, thus an underestimation is likely. Adding covariates to the model, such as smoking and alcohol consumption, in an attempt to separate direct from indirect effects of education may also introduce selection bias from the above mentioned other factors, eg, diet (24). The method by Karlson et al is relatively new and not discussed in detail in epidemiological settings, although already implemented in standard statistical software like Stata. Thus, an underestimation in comparison to the standard approach to calculate attributable fractions cannot be ruled out.
According to Karlson et al, in logistic regression the coefficients from different nested models are not measured on the same scale. Therefore their change across the models reflects both the confounding due to other covariates and the rescaling (4, 25). Their basic idea is to substitute the covariates of the fully adjusted model with the residuals of a reduced model from a regression on the covariates of interest. The estimates of the effect of the covariates on the outcome are on the same scale as the parameters from the full model and therefore comparable (7). Applying their method we obtained lower estimates than calculating the attributable fraction (AF), using the formula both on an additive scale, (ORR-ORA)/(ORR-1) and on a multiplicative scale, [ln(ORR)-ln(ORA)]/[ln(ORR)]×100 (17). Regardless of the method applied, CAI showed always the highest AF in the association of low educational level and laryngeal cancer, similar to Menvielle and colleagues findings (2). Their results were criticized as their occupational exposure variable and their job classification were derived from the same variable of origin. Therefore their model contained inherently correlated variables. To avoid this problem of collinearity, Boing at al (3) fitted separate models for education and occupation. As education and occupational class are structurally linked, inclusion of either variable would lead to a similar distribution of any specific outcome. Moreover, a dichotomous classification of job (manual versus non-manual) correlates with many social indicators such as income, health, and educational attainment, as well as conditions of employment broadly defined and therefore less informative (26). We checked different models considering pairwise interaction terms between our educational and occupational variables, as well as smoking behavior and alcohol consumption. However, no significant interactions between these variables were found. Our data showed a trend in the risk of laryngeal cancer through all educational levels. However, no significant OR and their percentage reduction were found for the middle level of education. This category includes people who attended an apprenticeship in a vocational school (“Berufschule”) and had the chance for career development up to low management level, representing a probable lower occupational burden. The limitations of case–control studies that may lead to various sources of bias are well known (27). Our response rate in controls was 62.4%, which is very satisfactory, however it does not rule out a possible bias. The response rates were lower for the youngest (<40 years) and oldest (>80 years) age groups. Unfortunately, no information on non-responders was available for this study. As both smoking and excessive alcohol consumption are strong risk factors for laryngeal cancer, we did a careful investigation on residual confounding. The confounding effect, in particular of smoking, was clearly visible. Most OR estimates reduced toward one after adjustment. We also checked for different methods of adjustment, using smoking/alcohol as categorical variable, resulting in negligible differences (28). The sample size was calculated on the basis of a 5% of prevalence of the risk factor in the control group. Confounding factors were not taken into account, although they might contribute to a reduction of the power but do not affect the relative power increase when increasing the case:control ratio from 1:2 to 1:3. The latter gives a power of 86% thus, the size of our study population facilitated the detection of also small relative risks.
The current approach aims to reflect carcinogenic agent exposure when detailed exposure information to substances is not available. A source of bias in occupational studies that include personal risk factors such as smoking can arise from the relatively better exposure assessment that may be available for smoking than the actual causative chemical exposures. Statistical models will tend to place a greater association with the better-specified exposure and this would of course impact the attributable fraction allocation. However, this source of bias could be considered as non-differential in our study.
Additionally, as occupational exposure through job titles might be even easier to characterize for males than females, we restricted our analyses only to male patients. Additionally, the usage of an index which reflects better working conditions in 2006 compared to those in the 1960s or 1970s might underestimate the real occupational burden and thus also the overall percentage attributed to all three considered factors. Employment pollution was surely higher in previous years, where programs for occupational health and safety were, at most, in the initial phase. Thus, we conducted some sensitivity analyses, changing the CAI levels for those jobs where we assumed a higher burden in the past (like industrial workers and painters). This resulted in a higher percentage attributed to occupation and in the overall percentage. An underestimation might be given for alcohol consumption as well as patients tend to underreport exposures. In a sensitivity analysis, we applied a second independent alcohol variable with a lower mean reported consumption, resulting in a lower percentage. This second alcohol variable asked for alcohol consumption in the period shortly before or at diagnosis. Although this variable might be of doubt as participants tend to underreport their actual consumption when a tumor is already diagnosed, it has the potential to show the effect of underestimating through underreporting of real exposure. The same might hold for smoking.
In conclusion, this study shows that more than half of the contribution often attributed to education is due to smoking, alcohol, and occupational risk factors in male laryngeal cancer. An underestimation of the contribution of these risk factors through underreporting (smoking/alcohol) or underestimation of the true exposure is likely to happen. Indeed, for the lowest educational levels, the occupational aspects contribute nearly with a similar percentage as smoking, which is the main risk factor for laryngeal cancer. Our study helps to better understand the triangle between education, occupation, and cancer outcome. For this, educational and occupational variables should be considered when adjusting multivariable models to separate their effects.