In Western countries, low-back pain (LBP) is the most common musculoskeletal disorder with high burden for the patient and high costs for society. The recovery process and course of LBP is variable between patients in intensity and duration over time (1). A possible explanation for this heterogeneity is the presence of different unidentified subpopulations of LBP patients. Information about the development of LBP over time is necessary to (i) get an impression of the variation of LBP between patients, (ii) identify patients who will transfer to chronic LBP, (iii) get reliable prognostic information and (iv) be better able to personalize an optimal treatment for each patient (2).
Also the extent to which a patient is at risk to develop chronic LBP is essential to make treatment decisions. Prognostic models may provide the evidence-based input for treatment decisions because they combine a number of patient characteristics that predict prognosis (3). In the past decades, many prognostic models have been developed, including several concerning the prognosis of patients with low back pain (4). These models vary with regard to patient populations and relevant prognostic factors but also with regard to the outcome measures. This hampers the generalizability and implementation of these models in clinical practice.
Von Korff showed that a definition for chronic LBP can better be based on information about the prognosis and course of LBP over time (2). Axén also showed that LBP cannot be considered as a self-limiting condition but one of a longer duration (5). Dunn studied the course of LBP patients in a general practice setting over a year. She identified four groups of LBP patients based on their initial pain values and the development of pain over time, using latent class growth analysis (LCGA) (6). Distinguished groups were a persistent mild group of patients, a recovering patient group, a severe chronic patient group and a fluctuating patient group (oscillating between mild-to-moderate and high pain). These studies explain the dynamic character of LBP in duration as well as intensity. Consequently, the outcome measure of a model to predict chronic LBP should be based on the course of LBP over time. Heymans developed a model to predict chronic LBP in an occupational setting and used the distinguished groups of Dunn to define chronic LBP (7). This model showed a good performance with an bootstrap-corrected area-under-the-curve (AUC) of 0.80 and an explained variance of 37%. However, Dunn et al (6) and Kongsted et al (8) used LBP patients from a general practice setting to classify their patients. These patients may differ in their course and development from LBP patients in an occupational setting which were used in the study of Heymans. This difference may influence the classification of LBP patients and consequently the definition of chronic LBP and the performance of the prediction model (8).
Therefore, this study aims to (i) identify subpopulations of patients in an occupational setting who will still have or develop chronic LBP and (ii) evaluate a previously developed prediction model based on the determined subpopulations.
Methods
Study sample
We merged data from three randomized controlled trials (RCT) conducted among patients with LBP in an occupational setting (7, 9–12). One study (N=299) compared high- and low-intensity back schools with usual care (7, 10). The second study (N=134) compared the effectiveness of a behavior-oriented graded activity program compared with usual care (11). The third study (N=195) compared a workplace intervention with usual care and a clinical intervention after eight weeks of sick-leave with usual care (9, 12). All three studies used the same in and exclusion criteria and the same follow-up measurements.
Measurements
Baseline data comprised: (i) participants’ characteristics [age (in years; continuous outcome), gender (dichotomous outcome), weight and body length (BMI, continuous)]; (ii) intervention groups [high-intensity back school (13), low-intensity back school (13), usual care, workplace management, physiotherapy based on operant behavioural principles, usual care provided by an occupational physician and combinations of these interventions; categorical outcome]; (iii) possible predictors (quality of life (Euroqol: continuous, range 1–100, where 100=good health), fear avoidance (Tampa scale: continuous, range 17–69, where a higher score=higher degree of fear avoidance), coping style [Pain Coping Inventory (PCI): continuous, higher score on scale 1, 2 and 3=active coping style and higher score on scale 4, 5 and 6=more passive coping style), disability (Roland Disability Questionnaire: continuous, higher score=greater disability), smoking (yes/no, dichotomous), radiation in a leg (yes/no, dichotomous), sick leave because of LBP (yes/no, dichotomous), experienced working situation (categorical); and (iv) outcome measure pain using a visual analogue scale (VAS) at four follow-up times: baseline, 3, 6 and 12 months (1–18).
Growth curve analysis
LCGA is a technique that assign individuals to distinct classes (subpopulations) based on individual pain trajectories so that individuals within a subpopulation are more similar than individuals between subpopulations regarding their course of LBP over time (19). In other words: the variance within the subpopulations is fixed to a value of zero compared to the variance between subpopulations, which is as large as possible. Each subpopulation has its own pain growth parameters (ie, intercept=pain starting value, (linear) slope=pain increase or decrease over time) which are the unobserved (or latent) continuous variables. To determine the optimal number of classes, a forward procedure was performed, starting with one class (ie, there are no subpopulations in the study sample), then adding more classes one at a time. To evaluate if the fit between these models with a different number of classes improved, ie, if more subpopulations can be identified, several steps can be taken:
Step 1
Fit indices, as recommended in the literature, were used as the Bayesian information criterion (BIC), the bootstrap likelihood ratio test (BLRT) and the entropy (2,20,21). The BIC considers both the likelihood of the model as well as the number of parameters in the model; a lower BIC value shows a better model fit. The BLRT provides a P-value, indicating that a model with one less class (k-1 class model) has to be rejected in favor of the k-class model. The entropy is a fit index for the classification quality and is defined by the posterior probabilities. These fit indices have shown to be consistent indicators of the optimal number of classes (21–23).
Step 2
We looked at the posterior probabilities of each class membership. Study participants were assigned to their most-likely class based on a probability of ≥0.7 (2, 21).
Step 3
We considered the usefulness and clinical relevance of the separate classes in practice for instance by the number of individuals in each class, which has to be at least one percent of the sample.
The number of distinct classes was first determined in patients that were treated with usual care (UC) (N=240). In this way, the intervention effect could not influence the number and type of pain subpopulations. Subsequently, the analyses with the determined number of subpopulations (classes) were repeated in each intervention group to study if the intervention influenced the course of the subpopulations. These subpopulations were used to derive the definition for chronic LBP. We defined a subpopulation as developing chronic LBP when the baseline measured pain >4 on the VAS scale and the one year pain score ≥4 on the VAS scale or when the baseline pain score is between 2–4 on the VAS-scale and after a year the decrease of pain <2 points on the VAS scale (6,7,24). Finally, subpopulations were recoded to form the dichotomous outcome (recovering or developing chronic LBP).
Missing data
Pain values at previous follow-up moments were related to missing pain values at later follow-up moments which resembles the missing at random (MAR) assumption. Mplus provides full information maximum likelihood estimation, meaning that when the missing data is MAR, Mplus uses all available outcome data to correctly estimate the growth parameters (25, 26). Five patients missed outcome data on all follow-up measures and were excluded from all models. We excluded one patient with missing data on the covariate age. Finally, we performed single stochastic regression imputation on the baseline measured variables with 1–7% missing data on disability, quality of life, anxiety, coping style, smoking, sickness, work situation, baseline pain and radiation (27).
Statistical analyses
To analyze differences in characteristics between the patients that were assigned to the different subpopulations, class membership was coded as a categorical variable representing the different subpopulations. ANOVA for continuous and Chi-square analyses for categorical variables were used to test differences in patient characteristics between the subpopulations. These analyses and the single stochastic regression imputation were conducted using SPSS 20 (IBM Corp, Armonk, NY, USA). P<0.05 was regarded as statistically significant. The LCGA were conducted using the Mplus 7.11 software package.
Testing a prediction model
The outcome of the prediction model developed by Heymans et al (7) was based on the LPB trajectories of the study of Dunn, ie, outcome based on non-occupational data (6). The prediction model that was developed in the study of Heymans et al included the predictors kinesiophobia, pain intensity and a clinically relevant change in pain intensity and functional status in the first three months. The performance of this prediction model was now in the occupational setting evaluated by using the newly defined definition of chronic LBP derived from the LCGA analysis as described above as outcome measure. For this evaluation a logistic regression model (enter method) was used. The performance of the model was evaluated by the explained variance (R2), the AUC and the Hosmer and Lemeshow goodness-of-fit test. Next, the prediction model was internally validated by performing 250 bootstrap draws.
Results
Study sample
In total, 622 patients were included in all analysis. The baseline characteristics and pain outcome of these patients are presented in table 1. Two of the three merged RCT measured duration of complaints (10, 12). In these studies, the median LBP duration was 5.8 weeks (range 2–780 weeks) so most patients suffered from subacute and chronic LBP.
Table 1
Baseline characteristics of the study population. [SD=standard deviation; RDQ=Roland Disability Questionnaire; EuroQOL=European Quality of Life measure of health outcome.]
Growth curve analyses
Table 2 shows that the model with four classes had the highest P-value in the BLRT (P=0.04), a higher BIC value compared to the 3-class model (3832.20) and a posterior probability <0.70. The model with two classes had a higher BIC value (3832.98) and a lower entropy (0.476) compared to the 3-class model. So, these indices seemed to favor the 3-class model in the UC intervention groups (N=240).
Table 2
Model Fit indices and subpopulation membership of models based on the usual care intervention groups (N=240). [LCGA=latent class growth analysis with x-classes; BIC=Bayesian information criterion; BLRT=bootstrap likelihood ratio test P-values.
Indices | LCGA(C2) | LCGA(C3) | LCGA(C4) |
---|---|---|---|
BIC | 3832.98 | 3829.11 | 3832.20 |
Entropy a | 0.476 | 0.559 | 0.681 |
BLRT | 0.0000 | 0.0000 | 0.0400 |
Lowest posterior Probability b | 0.82 | 0.77 | 0.69 |
Class 1: Chronic high persistent pain | |||
N | 110 | 110 | 72 |
% | 45.8 | 45.8 | 30.,0 |
Intercept | 6.446 | 6.778 | 6.953 |
Slope | -0.529 | -0.660 | -0.506 |
Class 2: Recovering high intensity pain | |||
N | 130 | 79 | 110 |
% | 54.2 | 32.9 | 45.8 |
Intercept | 5.223 | 6.552 | 6.692 |
Slope | -1.269 | -1.817 | -1.778 |
Class 3: Chronic mild persistent pain | |||
N | 51 | 31 | |
% | 21.3 | 12.9 | |
Intercept | 3.162 | 3.829 | |
Slope | -0.247 | 0.484 | |
Class 4: Recovering mild intensity pain | |||
N | 27 | ||
% | 11.3 | ||
Intercept | 2.946 | ||
Slope | -0.619 |
We performed the analyses with linear as well as quadratic techniques, however the quadratic techniques were not applicable in all smaller sample size intervention groups. Therefore we deemed the LCGA 3-class linear model as the most appropriate. The three trajectories also showed the most clinically relevant pain trajectories.
As an example, figure 1 shows the trajectories stratified by the intervention group’s workplace intervention-graded activity (WIGA) and UC. We chose the WIGA group because this was one of the smallest groups and the UC group was the largest. The trajectories in this figure are representative for all intervention groups. This means that the effectiveness of the interventions not strongly influences subpopulation membership. However, the rate of decline in pain during the first time period of an intervention seems to predict recovery.
Figure 1
Trajectories of pain on basis of a latent class growth analysis (LCGA) 3-class model in the usual care (UC) group (N=208) and in the workplace intervention-graded activity (WIGA) group (N=27.)
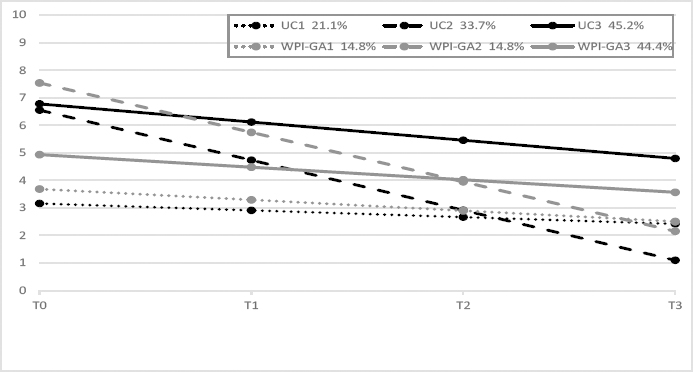
The three classes differed in slope and baseline value on the VAS score. These values were 6.778 VAS score with a slope of -0.660 for class 1 (chronic high persistent pain), 6.552 VAS score with a slope of -1.817 for class 2 (recovering) and 3.162 VAS score with a slope of -0.247 for class 3 (chronic mild persistent pain) (see table 2). After coding all patients, 353 patients recovered from LBP and 269 patients developed chronic LBP.
Characteristics of the classes
The characteristics of the patients in the three subpopulations chronic high persistent LBP, recovering from LBP and chronic mild persistent LBP were subsequently examined. Kinesiophobia, quality of life, active and passive pain coping, baseline pain intensity and radiation of pain showed significant differences between the classified patients (see table 3).
Table 3
Patient characteristics per class. [SD=standard deviation; RDQ=Roland Disability Questionnaire; EuroQOL=European Quality of Life measure of health outcome; Tampa=scale for kinesophobia; BMI=body mass index.]
Variables | N | Class 1 Chronic high persistent LBP (N=216) | N | Class 2 Recovering from LBP (N=353) | N | Class 3 Chronic mild persistent LBP (N=53) | P-value a | |||
---|---|---|---|---|---|---|---|---|---|---|
|
|
|
||||||||
Mean | SD | Mean | SD | Mean | SD | |||||
Continuous | ||||||||||
RDQ | 10.85 | 5.324 | 11.69 | 5.149 | 11.58 | 5.131 | 0.166 | |||
EuroQuol | 0.4902 | 0.29 | 0.5533 | 0.258 | 0.7007 | 0.20 | 0.000 | |||
Tampa | 40.77 | 6.437 | 39.68 | 6.7 | 36.26 | 5.943 | 0.000 | |||
Active coping | 6.8392 | 1.27 | 6.7696 | 1.19 | 6.1862 | 1.12 | 0.002 | |||
Passive coping | 6.7904 | 1.31 | 6.4049 | 1.26 | 5.5124 | 1.17 | 0.000 | |||
Age | 39.77 | 9.866 | 40.84 | 9.128 | 42.02 | 10.469 | 0.219 | |||
BMI | 25.94 | 3.95 | 25.85 | 4.10 | 25.95 | 3.63 | 0.966 | |||
Pain baseline | 6.86 | 1.555 | 6.35 | 1.710 | 3.25 | 1.413 | 0.000 | |||
Dichotomous b | ||||||||||
Radiation | 126 | 90 | 238 | 115 | 43 | 10 | 0.004 | |||
Smoking | 102 | 114 | 191 | 162 | 32 | 21 | 0.132 | |||
Sick Leave | 66 | 150 | 114 | 239 | 23 | 30 | 0.197 | |||
Gender (male) | 55 | 161 | 110 | 243 | 15 | 38 | 0.349 | |||
Job satisfaction | 0.717 | |||||||||
Good | 94 | 159 | 28 | |||||||
Fair | 90 | 144 | 20 | |||||||
Moderate | 22 | 38 | 5 | |||||||
Bad | 10 | 12 | 0 |
Evaluation of the prediction model
The regression coefficient estimates, odds ratios (OR), 95% confidence intervals (95% CI) of the variables in the prediction model and the performance are shown in table 4.
Table 4
Prediction model based on subpopulation patients developing chronic, adjusted for intervention groups low-back pain. [OR=odds ratio; 95% CI=95% confidence interval; PIMCIC=pain intensity minimal clinical important change; RDQMCIC=Roland Disability Questionnaire minimal clinical important change; AUC=area under the operating characteristic curve]
Coefficient | OR | 95% CI | P-value | ||
---|---|---|---|---|---|
Variable | |||||
Kinesiophobia | 0.024 | 1.024 | 0.996–1.053 | 0.090 | |
Pain intensity | 0.011 | 1.011 | 0.910–1.124 | 0.833 | |
PIMCIC | -0.894 | 0.409 | 0.224–0.746 | 0.004 a | |
RDQMCIC | -0.469 | 0.626 | 0.327–1.198 | 0.157 | |
Performance measures | |||||
Explained variance (R2) | 0.30 | ||||
Internal validated R2 | 0.26 | ||||
Hosmer & Lemeshow P-value | 0.54 | ||||
AUC | 0.77 | ||||
Internal validated AUC | 0.75 |
Where higher scores on pain intensity and kinesiophobia were responsible for an increasing risk of developing chronic LBP, the clinically relevant change in pain intensity and functional status in the first three months indicated a lower risk of developing chronic LBP.
The explained variance of the prediction model was 0.295. The performance of the model resulted in a non-significant Hosmer and Lemeshow test (P=0.543) and an AUC of 0.773.
Finally, we performed 250 bootstrap draws for the validation of the model. After bootstrapping, the R2 of the model decreased to 0.26 and the AUC of the recovery model decreased to 0.75 (see table 3). The prediction model based on these LBP pain trajectories showed a promising predictive performance.
Discussion
Main findings
The present study used prospective data of 622 LBP patients with a 12-month follow-up period to identify patients with distinct trajectories of LBP development in an occupational setting. All patients suffered at baseline from subacute or chronic LBP and were sick-listed for not more than 8 weeks because of their LBP complaints. Three LBP subpopulations were identified: one subpopulation with chronic LBP patients on a high pain level, one subpopulation with chronic LBP patients on a lower pain level and one subpopulation with patients recovering from LBP. The average probability of subpopulation membership for each estimated subpopulation was 73% or higher, suggesting that most participants were correctly classified. These subpopulations were dichotomized in a recovering (N=353) and a chronic (N=269) subpopulation and have been used to evaluate a previously developed prediction model for patients with LBP in an occupational setting with a promising predictive performance (AUC 0.773). An important finding is that if patients with high pain levels do not recover within a reasonable time limit during an intensive intervention, it is highly likely that they will remain having chronic LBP.
Comparison with the literature
In the literature, patients with chronic LBP are mostly classified based on their number of days in pain or on their pain history with a focus on LBP persistence (28, 29). Classifying patients based on trajectories of LBP is fairly new and provides a changed insight regarding prognostic and treatment strategies to reduce future risks of significant pain and or dysfunction. It offers opportunities to understand how patients differ in their course of LBP and helps identifying persons at risk for chronic LBP. This is, as far as we know, the first study in patients with LBP in an occupational setting that used LCGA to examine inter-individual differences in change in the course of LBP. Dunn examined the characteristics of the course of LBP with LCGA in a general practice setting and found four subgroups with a relatively stable development of LBP over a 12 months period (6). She defined two groups as chronic LBP, one group as fluctuating and one group as recovering LBP. Compared to the study of Dunn, our study showed a clear difference in the development of LBP over time between patient groups with patients showing an improved LBP trajectory, a chronic LBP trajectory on a high pain level and a chronic LBP trajectory on a lower pain level. We analyzed all intervention groups separately and compared these with the analysis in the total group (N=622). These analyses showed that some pain trajectories were different in some treatment groups, ie, showed a slightly different starting value or pain development over time, although these differences were small. Therefore it seems that the treatment that patients followed did not affect the course of the pain strongly. We may therefore conclude that the classification of patients into the newly defined outcome chronic or non-chronic LBP, which was derived from the pain trajectories, was not affected by the treatment effect. This was also to be expected because in the original studies the treatment effects on pain at follow-up were also small and non-significant (7, 10–13). Also the study of Kongsted found different trajectories of LBP. They determined trajectories that differed on LBP intensity, course patterns and the frequency of LBP. Their study identified slow recovering/severe ongoing LBP patients, recovering patients and moderate/mild ongoing LBP patients (8). These results are similar to our study. Kongsted et al also split the moderate/mild ongoing LBP patients group further in the subgroups labelled: ongoing, fluctuating and episodic pain. We were not able to identify these subgroups, probably because we measured pain intensity four times (baseline, 3, 6 and 12 months) in contrast to Kongsted et al who measured pain intensity each week for 1 year (8).
Other prediction models developed in an occupational setting showed a similar performance as our prediction models. Jensen at al developed also a prediction model in patients with LBP in occupational setting but only in a second health care setting. The AUC of this model was 0.79 so also a little better than the model of this study. This may be explained by the more homogenous patient group because all patients had only hospital-based interventions. Also the outcome measure was not a decrease in pain but unsuccessful return to work (30).
Strengths and limitations
Strength of our study is that we investigated the feasibility of the new modelling technique LCGA and that we showed that this technique has advantages in detecting different LBP patterns over time compared to simpler approaches of defining prognostic outcome by using single time points (19–21). Also, the longitudinal design is an additional strength of the study with four measurements over 12 months. A limitation may be the number of follow-up measurements. With a larger number possibly other pain subpopulations could also be identified. Another strength of this study is that we have actually examined whether the missing data of the outcome variables were missing at random. Although LCGA is an attractive analytic method to identify homogeneous subgroups, the method is still very young and explorative. The choice of the most appropriate number of subgroups remains somewhat arbitrary, requiring a trade-off between clinical and statistical judgement (8). Based on model fit and clinical arguments, we judged the three-class model as most appropriate.
The definition of chronic LBP depends on the cut-off point of the decrease of pain intensity after one year. Ostelo and de Vet explained the differences between the minimal clinical important change (MCIC) in pain intensity in acute back pain and subacute/chronic back pain (24). They suggest in case of chronic LBP a decrease of two points on the VAS scale as MCIC. Therefore we defined patients as suffering from chronic LBP when the baseline pain score as well as the pain score after one year was >4 on the VAS scale or when the baseline pain score was <4 on the VAS scale but after a year the decrease of pain was <2 points on the VAS scale. The explained variance (0.26) of the prediction model is not very high but is explicable by the heterogeneity of the study population. Further, all participants were extracted from an occupational setting so there were only 28.9% female participants. This is explicable because of the low work participation in heavy duty of women in the Netherlands. However adding gender as covariate to the unconditional model showed no significant differences between groups so there was no direct effect of gender on the classification of the participants.
Concluding remarks
We distinguished three homogeneous subpopulations of LBP patients in an occupational setting including one subpopulation with chronic LBP patients with a high pain level, one with chronic LBP patients with a lower pain level and one subpopulation with recovering patients. The prediction model with the outcome “developing chronic LBP” based on pain trajectories in patients with LBP in an occupational setting showed a promising bootstrap-corrected performance with an AUC of 0.75.