The prevalence of low-back pain (LBP) is high, especially in Western countries where it ranks the highest cause of disability (1). The costs of productivity loss and both sick leave and disability pension benefits are higher than the medical costs of LBP diagnosis and treatment (2). LBP sick leave is therefore a considerable societal burden and an important topic of research.
LBP sick leave is a multidimensional problem considering that it is associated with various individual, psychosocial, physical and work-related factors (3–5), which complicates the identification of workers at risk of LBP sick leave. Combining these factors in prediction models would improve the ability to determine the risk of LBP sick leave of workers. Several studies have developed prediction models for the duration or outcome of LBP sick leave among sick-listed workers (6–11). The ability of these models to discriminate between workers with and without long-term LBP sick leave was fair to good. After identifying these high risk workers, healthcare providers can refer them to interventions aimed at facilitating return to work shortly after reporting sick.
Prediction models that identify workers with an increased risk of LBP sick leave before they report sick would enable healthcare providers to refer workers at high risk of future LBP sick leave to preventive interventions. However, only few studies have examined models for predicting the risk of LBP sick leave in non-sick-listed workers (12, 13). Melloh et al (12) developed a prediction model that predicted the risk of LBP sick leave during 6-week follow-up of workers experiencing LBP, with job control, depression and functional limitations as predictor variables. The authors reported that the prediction model failed to predict LBP sick leave during longer follow-up periods. Salathé et al (13) succeeded in developing a prediction model for LBP sick leave during 1-year follow-up of workers experiencing LBP. The strongest predictors were a history of sick leave, work-related fear-avoidance behavior, and life satisfaction. There is still a need for more research on predicting the risk of LBP sick leave among healthy workers as the aforementioned studies only included workers who reported LBP at baseline, and it is well conceivable that these models do not apply to healthy workers without LBP. Furthermore, only the overall predictive performance of these prediction models was presented in terms of explained variances. Melloh et al (12) reported 47% explained variance for the 6-week model and Salathé et al (13) found 25–28% explained variance for the 1-year model.
The aim of the present study was to develop a prediction model specifically for the risk of LBP sick leave in non-sick-listed workers based on variables commonly measured in occupational health checks.
Methods
Study design and population
In the Netherlands, employers are obliged to enable their workers to participate in occupational health checks addressing health and working conditions every 2–4 years. Occupational health checks consist of a questionnaire followed by a consultation with an occupational physician (OP). Participation in occupational health checks is voluntary for workers. The present study was designed as a cohort study with the occupational health checks as baseline. The occupational health check variables were used as predictors of LBP sick leave during 1-year follow-up. We retrieved the occupational health checks performed among 32 383 construction workers between 2010 and 2013 because LBP is a major cause of sick leave among construction workers (14). The study population consisted of 22 648 manual workers (eg, stone masons, carpenters and plasterers) and 9735 non-manual (eg, technical workplace and office) workers in the construction industry as shown in Figure 1. Workers gave informed consent during the occupational health check for the use of the questionnaire data and sick leave data for research purposes. We wanted to investigate the performance of the LBP sick leave prediction model in different occupational settings. Therefore, the prediction model was developed among the manual workers and validated in the non-manual workers. Sick leave data recorded in the year following the occupational health check was obtained from an occupational health register.
Outcome variable
The outcome variable of this study was non-specific LBP sick leave, which was diagnosed by an OP. In the Netherlands, sick-listed workers consult with an OP within six weeks of reporting sick. OP certify sick leave with a diagnostic code derived from the 10th version of the International Classification of Diseases (ICD-10). Sick leaves OP-certified within the ICD-10 categories M54.3 (sciatica), M54.4 (lumbago with sciatica) and M54.5 (low-back pain) were defined as non-specific LBP sick leave. Since we used OP-certified sick leaves, the study only included long-term LBP sick leave as outcome variable; LBP sick leave had a median duration of 52 days (range of 3–730 days).
Predictor variables
Variables included in the occupational health check questionnaire were selected as potential predictors based on the literature on LBP sick leave (15–20) and based on the distributions of the variables. Variables with a narrow distribution (eg, gender 99.8% men) were discarded as predictors (21).
Health factors
The health check questionnaire measured age, pain/stiffness in the back (no=0, yes=1), pain/stiffness in neck, upper, and lower extremities (range 0–3 areas), number of physician-diagnosed musculoskeletal disorders/injuries (range 0–3 diagnoses), health complaints caused or worsened by work (no=0, yes=1), feeling healthy (no=0, yes=1), and sport activities (ranging from <1 time/month=0 to ≥3 times/week=4).
The hereafter mentioned variables were scored in accordance with a previous study on work stress among Dutch construction workers (22). The health check questionnaire items on “feeling irritated, agitated and/or depressed”, “difficulties with relaxing after work”, and “not feeling able to cope with the situation” were answered with “no” (=0) or “yes” (=1) and summed to the variable stress (Cronbach’s α=0.79).
Fatigue was measured with three items “feeling tired”, “feeling fit after dinner”, and “feeling exhausted at the end of a workday” (α=0.73). Items were answered with “no” (=0) or “yes” (=1) and were summed. Vitality included four items on “being active”, “being healthy”, “enjoying daily activities”, and “having confidence in the future” (α=0.74). Items were answered on a frequency scale ranging from “never” (=0) to “always” (=4), and were subsequently summed.
Work factors
Work factors included work satisfaction, which consisted of seven items on job insecurity, appreciation, rewards, and enjoying work (α=0.69). The organization of work included four items on work planning, communication at work, information on work tasks, and supervisor support (α=0.77). Psychological demands consisted of four items on mentally demanding work, working under time pressure, large amounts of work, and the number of working hours/week (α=0.61). Autonomy was measured with two items: “Can you decide how you do your work?” and “Can you influence your work pace?”. The six occupational health check questionnaire items on sitting, standing, and working in one position for a long time were clustered together with working in bended, kneeled, squatted, and awkward positions into the variable postural physical work demands (α=0.73). The health check items on lifting/pushing/pulling/carrying loads, repetitive movements, exerting large forces, and physically demanding work were clustered into the variable dynamic physical work demands (α=0.79). The items of the aforementioned work factors were answered with “no” (=0) or “yes” (=1) and were summed for each separate work factor.
The occupational health check questionnaire also included the items of the work ability index (WAI), which were summed to a workability score (range 7–49) according to the WAI guidelines (23). Furthermore, the number of years employed in the construction industry was assessed.
Statistical analysis
Statistical analyses were performed using SPSS for Windows, version 22 (released 2013. IBM Corp, Armonk, NY, USA) and R (statistical computing) version 3.2.5.
Prediction model development
The prediction model was developed in the sample of manual workers (N=22 648). Based on the criterion of ten events per variable (24) and the fact that 178 of the manual workers had LBP sick leave during follow-up, we decided to include a maximum of 18 variables in a multivariable logistic regression model with LBP sick leave during 1-year follow-up (no=0, yes=1) as dependent outcome variable. The number of predictors in the 18-predictor model was then reduced by statistical backward selection to develop an easy-to-use prediction model for healthcare practice. The Akaike’s information criterion (AIC) was used as stopping rule for the exclusion of predictors, which meant that all variables with P<0.157 remained in the model (25).
The Nagelkerke’s R-square was regarded as a measure for overall predictive performance (26). Calibration (ie, the agreement between predicted and observed LBP sick leave risks) was assessed by the Hosmer-Lemeshow goodness-of-fit test; P≥0.05 indicates that predicted risks do not deviate significantly from observed risks, meaning that calibration is adequate. The ability of the prediction model to discriminate between initially non-sick listed workers with and without LBP sick leave during follow-up was investigated with receiver operating characteristic (ROC) analysis. The area under the ROC curve (AUC) reflects discrimination.
The performance of prediction models is generally better for subjects used to develop the model than for new subjects, a phenomenon called over-optimism. This caused by the adaptation of the prediction model to the data in the development sample. The prediction model was adjusted for over-optimism by drawing 250 bootstrap samples from the manual workers and comparing the performance of the prediction model in the bootstrap samples with the performance in the original sample (21).
Performance of the prediction model across occupational settings
The over-optimism adjusted final model was then applied to the sample of non-manual workers (N=9735) to compare the performance from both groups.
Based on the 0.8% incidence of LBP sick leave among manual workers, we investigated specificities and sensitivities among non-manual workers for predicted probabilities of 0.2–3.6% (0.25–4× incidence).
Results
The characteristics of the study populations are shown in table 1. The manual workers reported more physician-diagnosed musculoskeletal disorders/injuries, experienced higher (postural and dynamic) physical work demands, and had more LBP sick leaves than the non-manual workers.
Table 1
Study population characteristics. [SD=standard deviation.]
Development of the LBP sick leave prediction model
When all 18 predictors were included in the logistic regression model (Nagelkerke’s R-square 3.8%), pain/stiffness in the back was the strongest predictor of LBP sick leave, followed by physician-diagnosed musculoskeletal disorders/injuries (table 2). After backward statistical reduction, six predictor variables (pain/stiffness in the back, number of physician-diagnosed musculoskeletal disorders/injuries, postural physical demands, feeling healthy, organization of work, and vitality) remained in the final model (Nagelkerke’s R-square 3.5%; over-optimism adjusted Nagelkerke’s R-square 2.9%). Calibration of the 6-predictor model was adequate, ie, the predicted LBP sick leave risks did not deviate significantly (model Chi2=11.168; df=8; P=0.192) from the observed LBP sick leave risks. Discrimination between manual workers with and without LBP sick leave during follow-up was poor (AUC=0.659; 95% CI 0.615–0.702); the over-optimism adjusted AUC was 0.648.
Table 2
Regression coefficients of the 18-predictor and the reduced 6-preditor models in the manual construction workers sample. [SE=standard error.]
Performance of the LBP sick leave prediction model
When applied to the non-manual workers, the explained variance of the 6-predictor model was 3.6%. The model showed adequate calibration (Chi2=8.455 df=7; P=0.294), but poor discrimination (AUC=0.692; 95% CI 0.568–0.815) (Figure 2) between non-manual workers with and without LBP sick leave during 1-year follow-up.
Figure 2
Receiver operating characteristic (ROC) curve of the 6-predictor model among manual construction workers; the diagonal indicated no discrimination above chance.
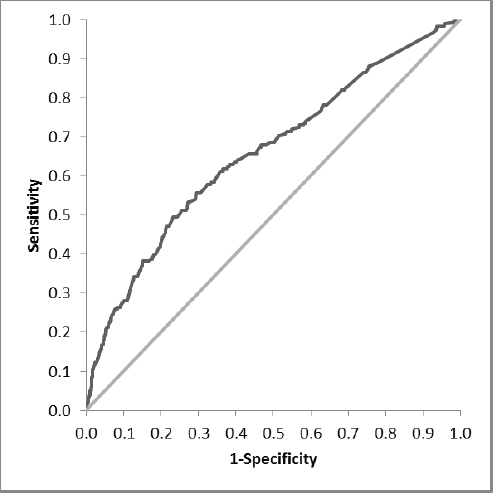
Specificities were higher and sensitivities lower in non-manual workers as compared with manual workers for all cut-off probabilities (table 3). Specificities ranged between 1.0–99.9%, and sensitivities between 5.9–100% in non-manual workers.
Table 3
Cut-off probabilities for the 6-predictor model.
Discussion
In this study, we developed a prediction model to identify non-sick listed workers at risk of LBP sick leave with variables measured in occupational health checks. A 6-predictor model including pain/stiffness in the back, physician-diagnosed musculoskeletal disorders/injuries, postural physical demands, feeling healthy, organization of work, and vitality as predictors showed adequate calibration, but low explained variance and poor discrimination between construction workers with and without LBP sick leave during 1-year follow up.
Predictors of LBP sick leave
Pain/stiffness in the back was the strongest predictor of LBP sick leave, in agreement with previous studies (15–18). Furthermore, musculoskeletal complaints have previously been reported to predict LBP sick leave (15, 19), although these were not physician-diagnosed. In agreement with previous studies, postural physical work demands was a strong predictor in the 6-predictor model (17, 19). However, dynamic physical work demands were excluded from the 6-predictor model, while frequent bending and lifting/carrying of loads are risk factors of LBP according to the guidelines for OP (27). Dynamic physical demands were also significant predictors of LBP sick leave in a study among nurses’ aides (15) and among blue- and white-collar workers (20). However other studies confirmed our present finding that dynamic physical work demands did not predict LBP sick leave (17, 18).
LBP sick leave prediction model
The performance of the prediction model was poor in terms of explained variance and discrimination. Previously, Salathé et al (13) reported that prediction models including gender, age, sickness absence at baseline, fear avoidance behavior, life satisfaction, job satisfaction, and doing sports explained 25–28% of the variance of LBP sick leave risk during 1-year follow-up. Melloh et al (12) reported that a prediction model with job control, depression and functional limitations explained 47% of the risk of LBP sick leave after 6 weeks, but none of the predictor variables predicted LBP sick leave during longer follow-up. Both studies included workers who experienced LBP at baseline. It is conceivable that predicting LBP sick leave is more difficult among workers without LBP complaints. Furthermore, the fact that the prediction model presented by Melloh et al (12) failed to predict LBP sick leave occurring >6 weeks after baseline underlines the difficulty of predicting sick leave during longer periods of follow-up. It can also be debated whether LBP is too heterogeneous and therefore more difficult to predict. However no clear consensus is reached on subgrouping non-specific LBP patients to decrease the heterogeneity (28).
Strengths and limitations of the study
The prospective design with different data sources (health check questionnaire for baseline predictor variables and occupational health register for LBP sick leave) are strengths of the present study. LBP sick leave was OP-diagnosed and registered instead of worker-reported, which minimized self-report bias.
The study population consisted of older workers who have been employed in the construction industry for a long time. In other words, they were healthy enough to stay in the construction industry (healthy worker bias). Furthermore, healthy workers are more likely to participate in occupational health checks than workers with health complaints (healthy volunteer bias) (29). Both biases may have under-estimated the associations between risk factors and LBP sick leave. The low incidence of LBP sick leave in the study population could also be explained by these types of bias. This restricted the number of variables to be included in the full prediction model and could well explain the low predictive performance in terms of explained variance (21).
The low explained variance also indicates that important predictors could have been missed. This study only used data collected by the occupational health check questionnaire. Consequently, not all known risk factors of LBP were included in the prediction model. Furthermore, the answer options of the items of the factors were often limited to “yes” and “no”, which provides less information and may have reduced the predictive performance of the factors. Additionally, it can be debated whether physical demands can be measured with self-report questionnaires as its validity is often found uncertain (30). However, physical demands measured by a questionnaire (15) and video recordings (20) were both predictive of LBP sick leave.
Practical implications and future research
The prediction model presented in this study is based on occupational health check data and could therefore be readily implemented on a large scale as a screening tool for LBP sick leave if the performance would be sufficient. Although the manual and non-manual workers differed on baseline characteristics, the performance of the model was similar in both populations. The AUC is slightly higher among the non-manual than manual workers, but considering the overlapping confidence intervals, this difference was not statistically significant. The similar performance would indicate that the prediction model performs equally in different populations.
The prediction model based on occupational health check variables poorly discriminated between workers with and without LBP sick leave during follow-up. However, the model’s risk predictions were adequate in the sense that the risks predicted by the model did not deviate significantly from observed LBP sick leave frequencies. The model could be used to estimate a worker’s risk of LBP sick leave. For example, if a construction company considers an ergonomic training aimed at preventing LBP, but is unable or unwilling to train all staff, then the workers at lowest risk of LBP sick leave could be excluded from the training. In the present study, there were no false negatives when the cut-off risk was set at 0.2% (ie, a quarter of the population incidence). In other words, none of the construction workers with a predicted risk of LBP sick leave lower than 0.2% had LBP sick leave during 1-year follow-up.
The occupational health check variables did not provide sufficient information to identify workers at risk of LBP sick leave. It should be noted that occupational health checks are conducted to address health and working conditions, not specifically for predicting (LBP) sick leave. Additional predictors of LBP sick leave are needed to improve the discriminative ability of the prediction model. The present study investigated a prediction model for risk of LBP sick leave among healthy workers. Since it is difficult to predict LBP sick leave among healthy workers, it might be better to focus on prediction models for sick leave durations and outcomes among workers who have reported sick with LBP sick leave because previous studies have shown higher performance measures for such models (6–11).