Workplace exposure assessment is necessary for effective assessment and prevention of health conditions that may be affected by occupational factors including physical (biomechanical) exposures. A job exposure matrix (JEM) is an efficient tool to estimate workers’ exposure to occupational risk factors by using job titles, industry information, and job-level exposure data. There has been considerable international interest in constructing JEM to estimate physical exposures for the study of work-related musculoskeletal disorders (MSD), leading to recent JEM created in Denmark (1), Norway (2), Finland (3, 4), the United States (5, 6), and France (7).
Several studies have validated physical exposure JEM and their association with various MSD, including low-back pain (3), hip and knee osteoarthritis (8, 9), carpal tunnel syndrome (CTS) (10), and subacromial impingement syndrome (11). More recently, we (12) validated a JEM (O*NET JEM) based on the American Occupational Information Network data (O*NET, www.onetonline.org) by comparing exposure–disease associations for incident CTS in a well-studied cohort of US workers (13). Exposure–disease associations obtained using physical exposures estimated from a JEM were similar to associations obtained from observations of individual workers (12). In a cross-national comparison of exposure estimates, we compared a French JEM based on self-reported physical exposures from a large cohort study (CONSTANCES – Cohorte des consultants des Centres d’examens de santé) (14, 15) with the American O*NET JEM based on exposure information provided by expert job analysts and from surveys of workers in different jobs. We found that exposure estimates from these two general population JEM were strongly related, suggesting that results obtained from different general population JEM were likely to be comparable. In some circumstances, it might be reasonable to combine exposures from different JEM to provide better estimates of some exposures (16).
The objectives of this study were to evaluate the ability of the CONSTANCES and O*NET JEM to detect known exposure–disease relationships in a large US prospective cohort study and to explore whether combining exposure variables from multiple sources of exposure information improved the prediction of health outcomes (figure 1). As an extension of our previous study (12), we first evaluated the predictive validity of the CONSTANCES and O*NET JEM by testing their ability to reproduce known exposure–disease associations obtained from individual-level measures (Aim 1). To further examine the relationships between the three sources of exposure data, we then compared associations and agreement of exposure estimates between the CONSTANCES JEM, the O*NET JEM, and individual-level measures obtained from a large US prospective cohort study (Aim 2). Finally, we compared multivariable models combining exposure data from both JEM and observations to determine whether a combined exposure variable model predicted CTS better than a model containing exposure data from a single source (Aim 3). To our knowledge, this is the first study to explore the combination of exposure variables from two general population JEM and individual-level measures in the prediction of MSD. A general population JEM that produces similar exposure–disease associations to individual-level measures, and which can serve as a strong complement to existing JEM, will be an effective tool to study the effects of workplace physical exposures on a variety of health conditions.
Figure 1
Three study aims: (i) evaluate exposure–disease associations between exposure estimates from three sources and incident CTS, (ii) compare association and agreement of exposure estimated and values both at individual and job-title levels, and (iii) compare mulitavariate model performance between combined models (O*NET + CONSTANCES, O*NET + Observed, CONSTANCES + Observed) and single source models.
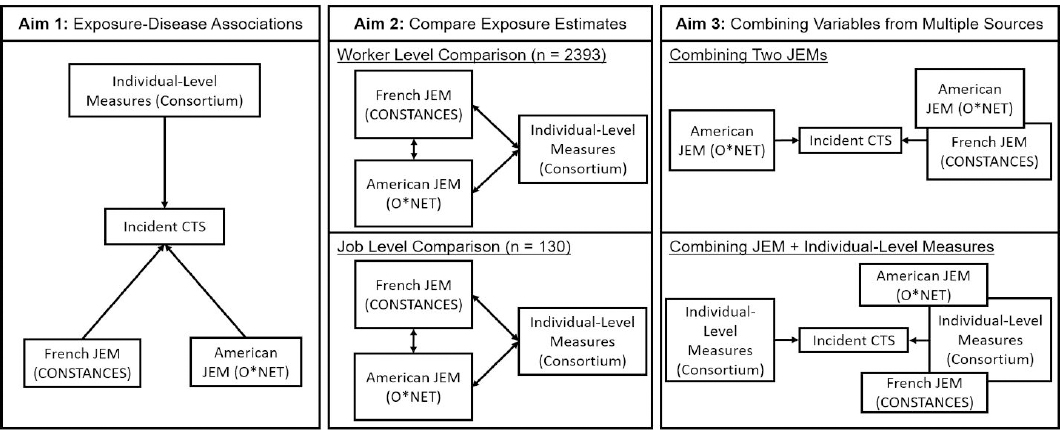
Methods
Individual-level measures
Cohort study methodology. Pooled exposure data were obtained from six prospective cohort studies conducted as part of the NIOSH upper-extremity MSD consortium. This cohort has been thoroughly described in previous studies (12, 13, 17–19). In brief, 4321 workers were recruited across six study sites and followed between 2001 and 2010. All study participants were full-time employees, >18 years of age, recruited from jobs that involved hand-intensive activities, and employed in manufacturing, production, service, and construction industries. Ethics approval was provided by the respective institutional review boards for each study and written informed consent was obtained from all participants.
Both hand/arm exposures and the health outcome (CTS) were assigned at the worker level. All study participants completed baseline questionnaires and underwent physical examinations, which included median and ulnar nerve electrodiagnostic tests. We defined incident CTS as (i): symptoms of tingling, numbness, burning or pain in the thumb, index finger or long finger, and (ii) abnormal electrodiagnostic tests consistent with median neuropathy at the wrist (12). Work exposure assessments were performed for each individual, consisting of interviews to identify primary work tasks, video recordings of workers performing typical work tasks, and worker- and analyst-rated estimation of hand forces required to perform each task. Physical exposure variables were relevant to MSD risk, including force, repetition, hand/wrist posture, and hand/arm vibration.
Individual-level measure variables. Peak hand force required for a task was assessed using the Borg category ratio 0–10 (CR-10) rating scale (20) and was obtained from both worker estimates [peak hand force (worker rated)] and from trained analysts [peak hand force (analyst rated)]. Video recordings of work tasks provided estimates of duty cycle, for all exertions in a task (duty cycle for all exertions) or for exertions requiring significant force (duty cycle of forceful exertions). Forceful exertions were defined as pinch force ≥9 N, or power grip force ≥45 N, or a Borg CR-10 rating of ≥2; estimates of force was based on measurement of the force required for the task, the weights of parts or tools, or from force matching. Repetitiveness of tasks were estimated using hand activity level (HAL) ratings, which were calculated using Latko et al’s (21) 0–10 verbal anchor scale determined by analysts who assigned ratings by observation in the field and from video analysis of worker tasks. Other temporal exertion patterns for repetition was determined by detailed time studies of video recordings for all exertions (repetition per minute for all exertions) and for significant exertions (repetition per minute for forceful exertions). The American Conference of Governmental Industrial Hygienists (ACGIH) threshold limit value (TLV) for HAL was calculated to provide a single composite index value from a combination of job physical exposure factors (force and repetition) (22). Both worker- and observer-estimated TLV were calculated using the equation score of TLV = peak force/(10-HAL), using worker- or analyst-rated peak force, and the analyst-rated HAL. Finally, using video recordings of work tasks, we calculated the percentage of time in wrist extension (≥50 degrees) or flexion (≥30 degrees). Workers who performed multiple tasks in their job, or their supervisors, provided estimates of the proportion of time spent in each task. We calculated a time-weighted-average (TWA) exposure to create a single exposure variable measure that accounted for the proportion of daily work time in each observed task.
CONSTANCES job exposure matrix. A general population physical exposure JEM was constructed from self-reported data obtained from CONSTANCES, a large cohort study of French salaried workers (14, 15). Details of the creation of this JEM have been described in Evanoff et al (7). Briefly, in CONSTANCES, the duration of performing specific activities of a given frequency or intensity were self-reported for the current job using a 4- or 5-point ordinal scale; physical intensity was assessed with Borg’s rating of perceived exertion (RPE) scale. The CONSTANCES JEM focused on 27 physical risk factors relevant to MSD and used data from the first 81 425 CONSTANCES participants. Reported job titles were assigned a 4-digit PCS (profession et catégorie sociale - profession and social category) job code using the SiCore automated coding system (23). When required, job codes were grouped with similar codes to ensure all PCS job codes had a minimum of ten valid responses for each of its 27 physical risk factors. The resulting JEM was comprised of 27 physical risk factors assigned to 407 different 4-digit PCS codes among 35 526 eligible CONSTANCES participants after excluding participants who (i) were not currently working, (ii) did not report a job title, (iii) were not assigned a PCS job code through automatic coding, or (iv) had missing exposure data.
O*NET job exposure matrix. A JEM was created using physical job demand data obtained from O*NET (version 21.2), a publicly available American database of more than 800 occupations. Estimates of job demands, pertaining to the frequency and intensity, were provided by expert job analysts and from self-reported exposures by individual workers across different jobs. Job demands in O*NET were scored on a 5- or 8-point ordinal scale with exposure-specific descriptive anchors (16). Occupations from O*NET were assigned a standard occupational classification (SOC) job code.
Assigning JEM exposure estimates to individual workers
From the 4321 original pooled consortium study cohort, we excluded prevalent cases of CTS at baseline and removed subjects with no follow-up measurements, workers who did not have detailed data necessary for individual assessment, and workers who had missing covariate data, leaving 2393 workers for analysis of CTS incidence. This analyzed cohort was identical to a previous study on exposure–disease associations that used a smaller set of O*NET exposure variables (12). SOC codes were assigned to each job for each worker using information about the worker’s current job (ie, job title, company name, job start date and work-related tasks) collected at baseline (12). The job title selection feature provided by O*NET assisted in assigning SOC codes to match primary tasks reported by the worker and from employer information. Two raters, blinded by case status, assigned job codes independently, with differences resolved by consensus. For assigning exposure values using the CONSTANCES JEM, we created a crosswalk to match French PCS codes with American SOC codes based on similarity of work physical exposures (16). French PCS codes were first matched to three-digit ISCO-88 (International Standard Classification of Occupations) codes using the Codage Assisté des Professions et Secteurs d’activité (CAPS) and an existing French auto-coding tool (24). ISCO-88 codes were then matched to ISCO-08 codes using an existing crosswalk from the International Labour Organization (25). Finally, ISCO-08 codes were matched to American SOC codes using an existing crosswalk from the US Bureau of Labor Statistics (www.bls.gov/soc). The mean CONSTANCES ordinal score for each exposure variable was then assigned to each participant’s SOC code. A similar process was performed when assigning exposure values using the O*NET JEM. The mean O*NET ordinal score for each exposure variable was assigned to each consortium participant. For every exposure variable, each of 2393 participants was assigned an exposure value from the individual-level measures, an exposure estimate from the CONSTANCES JEM, and an exposure estimate from the O*NET JEM. We focused on exposures relevant to incident CTS: 11 exposure variables from the CONSTANCES JEM, 8 variables from the O*NET JEM, and 11 variables from the consortium study (supplementary material: www.sjweh.fi/show_abstract.php?abstract_id=3855 tables S1 and S2). Variables such as “stand” and “work outdoors” were excluded from analysis as they were not expected to be related to CTS. Dale et al (12), reported exposure–disease associations for CTS using a subset of physical exposures from O*NET (12); in this study we examined a larger set of O*NET variables.
Statistical analysis
Aim 1: Physical exposures and incident CTS. We computed Cox proportional hazard models to evaluate relationships between baseline physical work exposure and incident CTS. We determined hazard ratios (HR) and 95% confidence intervals (CI) adjusted for age, gender, body mass index (BMI), and study site. Each model included a single physical work exposure from the CONSTANCES JEM, O*NET JEM, or individual-level measurement. Since exposure data from all sources were expressed on different scales, we examined a dichotomous exposure model where values were split at the median value (high versus low), in addition to continuous exposure models (per 1-unit increase). We applied robust sandwich estimators (26) to account for intra-cluster dependence within each model.
Aim 2: Comparison of exposure estimates between JEM and individual-level measures and between JEM at the worker- and job-level. We carried out two comparison analyses: Spearman’s rank correlation coefficient and Cohen’s kappa. We matched a priori similar exposure variables in order to compare exposure estimates between JEM and observation. Matched exposure variables assessed similar ergonomic risk factors (ie, force, repetition, posture, duration). For the O*NET JEM, we matched ≥1 of 8 O*NET variables to 11 consortium variables, resulting in 41 matched pairs (supplementary table S1). For the CONSTANCES JEM, we matched ≥1 of 9 CONSTANCES variables to 11 consortium variables, resulting in 54 matched pairs (supplementary table S2). Between CONSTANCES and O*NET JEM, we matched 8 O*NET variables with 9 CONSTANCES variables, resulting in 28 matched pairs (16). Job title data represented 130 unique American SOC codes matched to 77 unique French PCS codes.
We assigned exposure values from both JEM and from individual-level measurement to each of 2393 workers, and calculated Spearman correlations and Cohen’s kappa at the worker level between: (i) O*NET JEM and consortium exposures, (ii) CONSTANCES JEM and consortium exposures, and (iii) CONSTANCES and O*NET JEM. We also performed the same comparisons at the level of the job within the 130 job codes contained in the consortium data. When calculating Cohen’s kappa, we dichotomized physical exposure estimates from the CONSTANCES JEM, O*NET JEM, and consortium individual-level measure exposure data at their respective median physical exposure levels. We interpreted our Cohen’s kappa calculation as the level of agreement in categorizing high and low exposure groups between exposure methods for each paired exposure variable.
Aim 3: Comparison of models consisting of CONSTANCES, O*NET, and individual-level measures in predicting incident CTS. Our third aim was to compare models based on their performance, including measure of goodness-of-fit. In order to compare models, we used binomial logistic regression with research site as a random intercept; there are few readily implemented tests for goodness-of-fit for Cox proportional hazard models (27). Each model included a set of physical work exposures from the CONSTANCES JEM (11 variables), O*NET JEM (8 variables), and consortium individual-level measures (11 variables). For all binomial logistic regression models, we selected a fixed follow-up time of two years for all participants; this two-year period ensured that data from all six study sites were retained in our analysis, leaving 2173 eligible participants for analysis. We excluded all participants who had missing exposure data, leaving 1073 participants.
For the multivariable model analysis, we performed backward selection by Akaike information criterion (AIC) (28), to retain a subset of variables, adjusted for age, gender, BMI, and research site, for models containing CONSTANCES, O*NET, or consortium exposures. For each model, we calculated the c-statistic as a measure of goodness-of-fit of models with multiple variables from each exposure method.
To explore the prediction of incident CTS by combining variables across JEM or adding JEM data to individual-level measures, we compared an O*NET-only model to O*NET + CONSTANCES, and a consortium exposure-only model to consortium + O*NET and consortium + CONSTANCES. For these combined models, we carried out backward selection by AIC, retaining a subset of JEM variables into a model with our a priori selected covariates (age, gender, BMI, research site) and O*NET or consortium variables identified in the O*NET or consortium variable-only analysis. We computed AIC estimates (29), and the c-statistic, between the O*NET model and CONSTANCES + O*NET model, between the consortium model and consortium + O*NET model, and between the consortium model and consortium + CONSTANCES model. We compared c-statistics by calculating the 95% CI with bootstrapping resulting in a one-sided bootstrapped P-value.
All analyses were carried out with R software (R Foundation for Statistical Computing, Vienna, Austria).
Results
Aim 1: Physical exposures and incident CTS
HR for consortium individual-level measures ranged from 1.00–1.42 for continuous models. Of 11 consortium exposure variables, 9 were statistically meaningful, with the highest HR value observed with ACGIH TLV (analyst rated). In analyzing the dichotomous models, 5 exposure variables were statistically meaningful.
All 8 O*NET variables were statistically meaningful in their relationship to incident CTS. HR ranged from 1.31 (95% CI 1.01–1.70) (wrist finger speed) to 2.01 (95% CI 1.55–2.59) (spend time using your hands) in the continuous models. We observed HR ranging from 0.99–1.64 in the dichotomous models where 4 variables were statistically significant.
HR from continuous models were in the range of 1.08–2.05 for CONSTANCES exposure variables (table 1). Of 11 variables, only 2 were statistically meaningful. “Finger pinch” demonstrated the highest HR of 2.05 (95% CI 1.38–3.06), followed by “rotate forearm” (HR 1.44, 95% CI 1.10–1.89). Dichotomous models showed HR between 0.81–1.46, only “repetition” was statistically significant.
Table 1
Hazard ratios (HR) and 95% CI calculated from Cox proportional hazard models to evaluate relationship between baseline physical exposure and incident CTS. Adjusted for age, gender, body mass index (BMI), and research site. Applied with robust sandwich estimates (Lin & Wei, 1989). N=2393. [JEM=job exposure matrix]
In summary, we observed significant exposure–disease associations using O*NET JEM exposure variables to predict CTS in a US worker population; these associations were broadly similar to variables assessed by individual-level measures. We also observed some, but fewer, significant exposure–disease associations using a CONSTANCES JEM to predict CTS in a US worker population.
Aim 2: Comparison of exposure estimates between JEM and individual-level measures and between JEM at the worker- and job-level
Aim 2 results are reported in supplementary figures S1–4. We provide an example that is indicative of overall trends and briefly describe the supplementary results. In our example, correlation coefficients between worker-assigned CONSTANCES JEM and consortium individual-level measure exposure variables ranged from -0.01 to 0.36 (figure 2a). Among the 54 matched pairs, 9 pairs demonstrated low positive correlations while the remaining 45 pairs were negligibly correlated. Unmatched pairs resulted in negligible-to-low correlations. Between CONSTANCES and consortium variables, Cohen’s kappa values ranged between -0.07 and 0.37 (figure 2b). Of the 54 matched pairs between CONSTANCES and individual-level measures, 17 pairs demonstrated fair agreement. Unmatched exposure variable pairs showed low-to-fair agreement.
Figure 2
Heat maps of (a) Spearman’s correlations and (b) Cohen’s kappa agreement values at the worker level between CONSTANCES and individual-level measures. Underlined values denote matched exposure variable pairs. (N=2393 workers.)
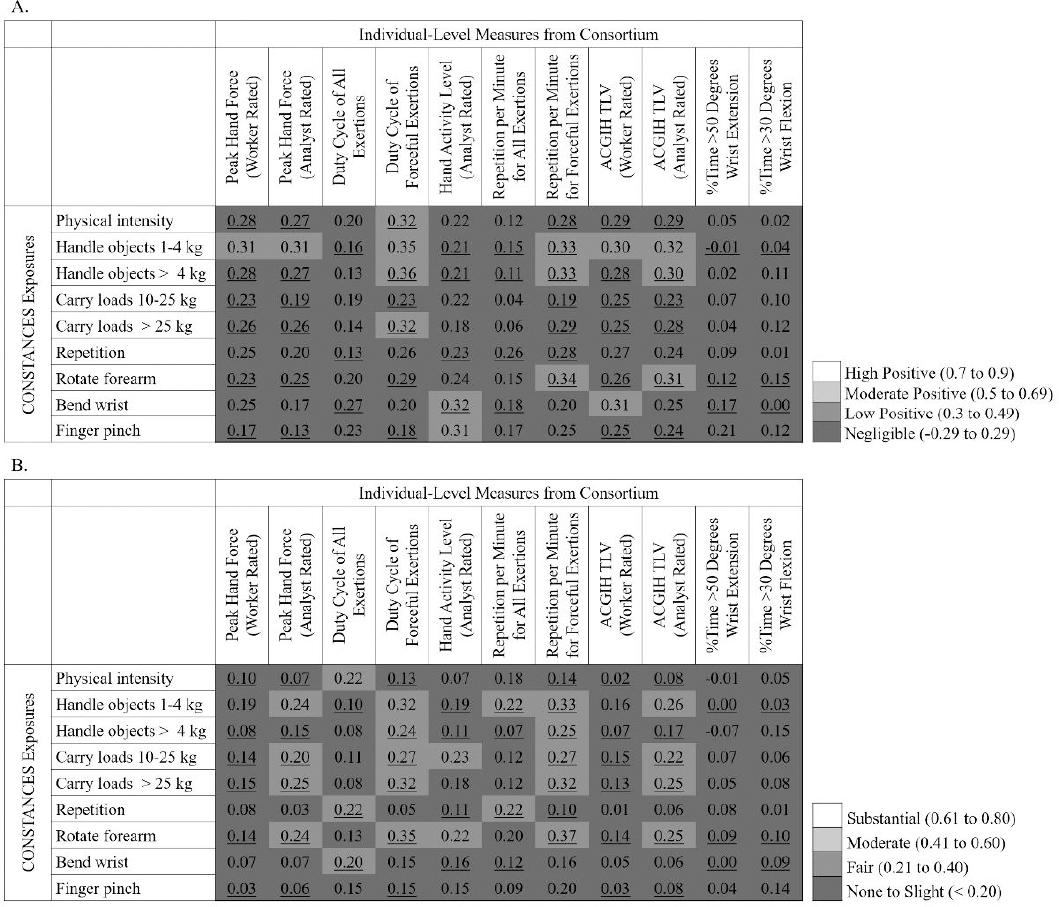
We also compared O*NET and CONSTANCES JEM exposure variables with consortium individual-level measures at the job level for 130 SOC job codes. Between CONSTANCES and consortium estimates, correlations ranged between 0.06 (negligible) and 0.59 (moderate). Of 54 matched pairs, 27 were moderately correlated (figure 3a). Between CONSTANCES JEM and consortium individual-level measures, 23 matched pairs demonstrated moderate agreement (figure 3b). Cohen’s kappa values ranged between -0.02 and 0.51.
Figure 3
Heat maps of (a) Spearman’s correlations and (b) Cohen’s kappa agreement values at the job level between CONSTANCES and individual-level measures. Underlined values denote matched exposure variable pairs. (N=130 SOC codes.)
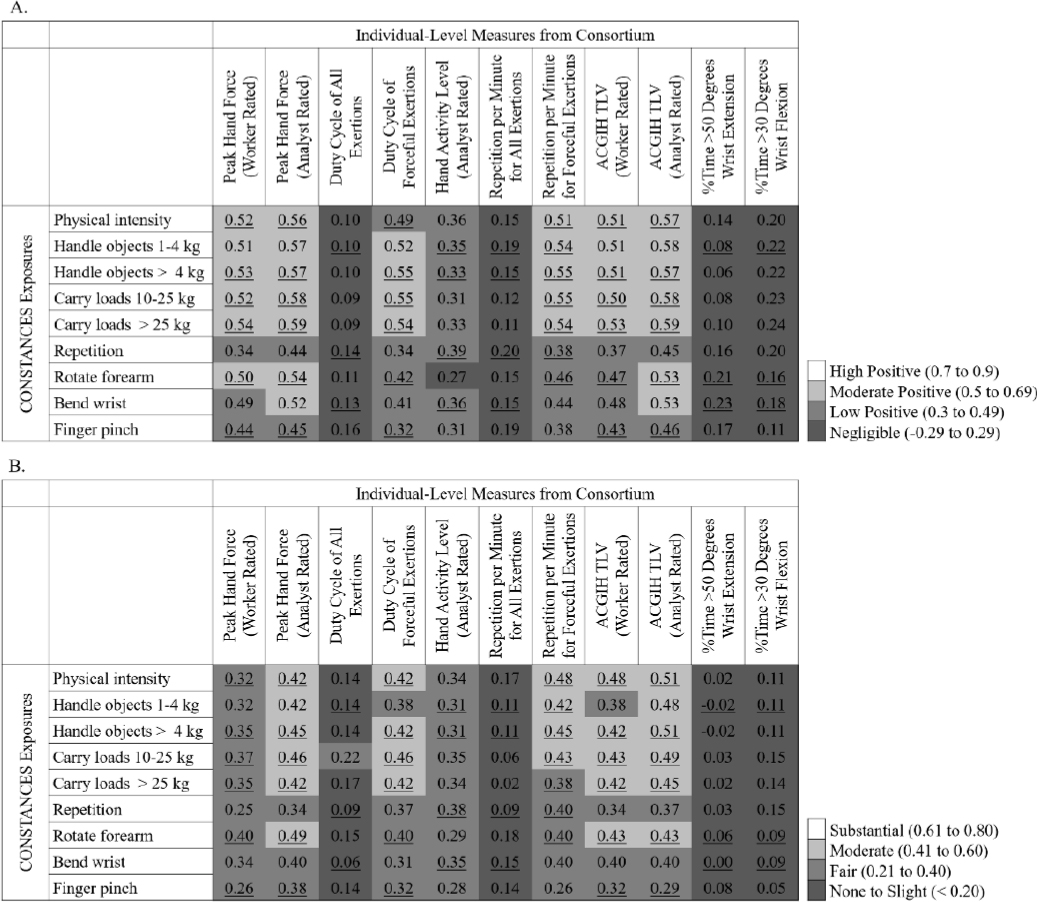
In our results, we found mostly low correlations and slight agreement between matched variables from the O*NET JEM and consortium individual-level measures when assigned to workers (supplementary figures S1a–b). Comparing CONSTANCES and O*NET JEM exposure estimates assigned to workers, the correlations between matched variables were minimal but agreement was fair to moderate (supplementary figure S2a–b). When comparing exposure estimates at the job level, correlation and agreement between CONSTANCES JEM, O*NET JEM, and individual-level measure variables were substantially higher (supplementary figures S3a–b and S4a–b).
Aim 3: Comparison of models consisting of CONSTANCES, O*NET, and individual-level measures in predicting incident CTS
The analyzed cohort from aims 1 and 2 was compared to aim 3’s restricted sample. We observed similar mean age and BMI between the two analyzed samples (supplementarey table S3), however, the restricted sample had a larger proportion of female workers (60.4% versus 52.2%) and a higher incident rate of CTS (4.8 cases per 100 person-years versus 3.9 cases per 100 person-years). The number of SOC job codes represented within the analyzed cohort decreased from 130 SOC codes in the aim 1 and 2 sample to 113 SOC codes in the aim 3 sample; the proportion of workers per job code in each sample showed little difference, with a maximum of 5% (supplementary table S4). Between the two samples, mean physical exposure levels for the 11 consortium variables were similar (supplementary table S5).
We also compared exposure–disease associations between the aim 1/aim 2 full, and aim 3 restricted samples. We computed log-binomial regression odds ratios (OR) for each variable within the three sources of exposure data (supplementary table S6). Generally, in both full and restricted samples, we observed statistically meaningful relationships between exposure variables, within each of the three sources of data, and incident CTS.
Backward selection based on AIC resulted in three retained variables in each of CONSTANCES, O*NET, and consortium models (table 2). We also listed the exposure variables within each combined variable model and compared their performance against a single source model (table 2). For example, the O*NET + CONSTANCES model consisted of five CONSTANCES variables added to the three O*NET job demands. When compared to the O*NET variable-only model, the O*NET + CONSTANCES model had a significantly higher c-index (P=0.02) and a lower AIC (Δ=-13.0). We observed similar trends with the combined consortium + O*NET and consortium + CONSTANCES models where c-indices were significantly higher (P=0.01 and P=0.02, respectively) and the AIC values were lower (Δ=-16.6 and Δ=-10.30, respectively) from the single source model.
Table 2
Retained variables for each source of physical exposure data and multivariable model comparisons. Restricted to 2-year follow-up period. N=1073. Adjusted for age, gender, BMI, and research site (random intercept). [AIC= Akaike information criterion.]
Exposure variables a | Binomial logistic regression criterion | ||
---|---|---|---|
|
|||
C-Index | P-value b, c | AIC | |
Single source models | |||
Consortium (individual-level measures) | 0.716 | ||
Peak hand force (worker rated) | |||
Repetition per minute for all exertions | |||
%Time ≥30 ° Wrist Flexion | |||
CONSTANCES JEM | 0.755 | ||
Finger pinch | |||
Handle objects >4 kg | |||
Carry loads <10 kg | |||
O*NET JEM | 0.749 | ||
Dynamic strength | |||
Spend time making repetitive motions | |||
Spend time using your hands | |||
Combined variable models | |||
O*NET JEM | 0.749 | 614.676 | |
O*NET JEM + CONSTANCES JEM | 0.780 | 0.02 | 601.662 |
Repetition, rotate forearm, handle objects >4 kg, carry loads <10 kg, bend wrist | |||
Consortium (individual-level measures) | 0.716 | 624.505 | |
Consortium (individual-level measures) + O*NET JEM | 0.753 | 0.01 | 607.878 |
Dynamic strength, spend time making repetitive motions, spend time using your hands | |||
Consortium (individual-level measures) + CONSTANCES JEM | 0.759 | 0.02 | 614.202 |
Finger pinch, handle objects >4 kg, carry loads <10 kg |
a Variables for both JEMs and individual-level measures retained by backward selection based on AIC.
b One-sided bootstrapped P-value of statistical differences between c-statistics using 95% CI with 1000 bootstraps.
In summary, combining exposure variables from two sources of exposure information improved model performance compared to a single source of exposure information. Combining CONSTANCES JEM variables with O*NET JEM variables better predicted CTS than a model of only O*NET variables. Combining either CONSTANCES JEM or O*NET JEM exposure variables with individual-level measures also improved model performance.
Discussion
There is a growing interest in applying physical exposure JEM to improve prediction of MSD and other health conditions by incorporating exposure variables to cohorts where no other work exposure data exists, or complementing existing sources of exposure information by combining exposure methods. There is also interest in comparing results obtained from JEM developed in different countries. We observed meaningful exposure–disease associations with incident CTS in a US worker population using both the American O*NET and the French CONSTANCES JEM, with a greater number of associations found with the O*NET JEM. Combining exposures from two JEM or combining exposure from a JEM with individual-level measures improved the prediction of CTS in exploratory models.
We previously evaluated a subset of O*NET exposure variables and their relationship with incident CTS (12) and found that O*NET JEM exposure estimates predicted CTS with similar effect sizes as exposure values obtained from individual-level measurement. In this study, we extended this evaluation by including three additional O*NET variables and found similar significant exposure–disease associations using O*NET JEM exposure estimates, particularly with variables related to strength and job demands requiring hand motions. We also evaluated the French CONSTANCES JEM, which includes physical exposures not available through O*NET, including pinch grip, hand or wrist posture, and hand vibration. CONSTANCES exposure variables pertaining to repetition were statistically meaningful in both continuous and dichotomous survival analysis models, while “rotate forearm” and “use vibrating tools” were statistically meaningful predictors in log binomial analyses. Differences between O*NET and CONSTANCES questions and scales might help explain contrasts in the exposure–disease associations between seemingly similar exposure variables. Generally, O*NET variables address the magnitude or intensity of exposure whereas CONSTANCES variables pertain to duration of performing specific actions at a specific intensity or frequency.
Although we observed meaningful exposure–disease associations with incident CTS using CONSTANCES and O*NET JEM, we observed negligible-to-low correlations and low-to-fair agreement between individual-level measures and both JEM. These results, in part, likely reflected the different scales and methods used to obtain exposure data from CONSTANCES, O*NET, and the consortium study. We previously found moderate-to-high positive correlations and moderate-to-substantial agreement between CONSTANCES and O*NET exposure variables at the job level for 367 job codes (16); in that analysis, each job code was weighted equally. In the current study, we observed higher correlations and agreement between JEM exposure estimates when comparing agreement at the job level than when comparing agreement at the worker level; when assigning exposure estimates at the worker level, correlations and agreement are dependent on the distribution of workers in different jobs within the population. In order to compare with worker level observation, the current study also compared a smaller number of job codes (130 SOC codes and 77 PCS codes) than the previous CONSTANCES to O*NET comparison (367 job codes). The strength of agreement between JEM and other exposure estimates may be influenced by the distribution of the worker population and the number of jobs under study; both of these factors may affect the within-job versus between-job variation in JEM-based exposure estimates.
Differences in constructs between general population JEM provide an opportunity to combine complementary variables into a single multivariable model. Hybrid exposure assessment methods have been used to study prostate cancer risk (30) and shoulder disorders (1). Since the development of MSD is multifactorial (31), relying on a single source of exposure information may not provide the optimal breadth of physical exposure data, and hybrid exposure methods offer the opportunity to improve precision while maintaining the efficiency of a JEM. Our exploratory comparison of multivariable exposure models supports the use of combining data obtained from individual-level measures with JEM data, and combining data from different JEM. Combining exposure variables from a French population JEM with an American JEM to predict CTS in a US worker population improved model performance. Combining JEM variables with individual-level measures also seemed to improve the prediction of incident CTS. We observed decreases in AIC compared to the baseline model, indicating that combined variable models provided better approximations than single source models (29). These findings warrant further research in combining exposure data from different methods to better predict risks of work-related MSD.
Alongside our study strengths, there are several limitations. First, we used a sample size of 2393 workers for aims 1 and 2, allowing for comparisons using the widest range of available job codes and time-to-event analysis; in aim 3, we used a restricted sample of 1073 workers in order to compare predictive models. Despite slight differences in demographics, incidence rate of CTS, and fewer SOC codes represented within the restricted sample, the mean physical exposure levels between the two samples were similar. Second, the crosswalk process for matching French PCS codes with American SOC codes required a multistep process. Existing software and tools assisted with assignment of job codes; however, there is no certainty that crosswalked jobs have the same tasks with similar exposures, nor that job tasks performed in identical jobs in different countries are the same. Furthermore, the number of job codes reflected in this analysis was on a portion of all possible job codes within each country. These differences may have contributed to the fewer exposure–disease associations found using the CONSTANCES JEM.
Overall, our results suggest that O*NET JEM and to some degree CONSTANCES JEM can reproduce known exposure–disease associations obtained from individual-level measures. Combining exposure estimates between two JEM and between JEM and individual-level measures improved the prediction of CTS when compared to single source models. Exposure information from a JEM could potentially enrich existing individual-level datasets or complement an existing JEM that might lack particular exposures. These preliminary findings using cross-national JEM are encouraging, but clearly, more investigations are needed to explore exposure-disease associations in other samples and combinations of exposure data from different methods to better predict MSD risk.
Concluding remarks
Both O*NET and CONSTANCES JEM demonstrated meaningful exposure–disease associations with incident CTS. The O*NET general population JEM demonstrated generally similar results as individual-level measures when calculating exposure–disease associations for CTS in the same worker cohort while the CONSTANCES JEM demonstrated fewer associations for CTS. This suggests that these JEM are useful tools for estimating workplace physical exposures in population studies. In exploratory models, adding exposure data from JEM to individual-level measures improved the prediction of incident CTS in our study, as did combining data from JEM from two different countries. These data suggest potential for combining exposure methods to improve the estimation of workplace physical exposures for surveillance and epidemiology studies. The performance of a general population JEM is influenced on the distribution of jobs within the studied worker population; in most cases it is likely that a larger variability of jobs within a studied cohort will improve the ability of general population JEM to demonstrate exposure–disease associations.