Obesity and sleep problems are now epidemic among working populations. Worldwide, the prevalence of obesity has more than doubled since the 1980s (1, 2). In the US, it is estimated that one third of adults are obese (3). In European countries, including Finland, the prevalence has reached 10–30% (4, 5). The obesity epidemic has been paralleled by a trend of sleep curtailment (2, 6). Approximately 10–30% of adults report frequent sleep problems, and the prevalence is increasing in several countries (7–10).
These trends are of particular concern because both obesity and sleep problems are risk factors for premature mortality (1, 11–13), and researchers are now beginning to pay attention to the close association between the two. On the individual level, associations between obesity and sleep are bidirectional. The poor quality of sleep may play a role in the development of obesity (2, 14), but obesity is also a risk factor for sleep apnea, an important cause of disturbed sleep (1, 6, 15). The prevalence of sleep problems among obese individuals varies between 16–42% (16, 17). Many of these associations are not large by magnitude, and, as such, they might not be important clinically at the individual level. However, even a small increase in risk can still have important consequences at the population level. Therefore, findings of a bidirectional association of obesity and sleep problems are of public health significance.
These two epidemics may have shared determinants (common causes), and at least obesity has also been shown to spread and cluster in social networks (18). However, few studies have examined clustering of co-morbidities for obesity, such as sleep problems, or considered the shared environmental causes of obesity and sleep problems. For example, socioeconomic adversity may underlie both outcomes (3, 19, 20). It is also possible that unhealthy working conditions – such as work stress or shift work – are responsible for both sleep problems as well as obesity via unhealthy eating habits or low physical activity (8, 21–24). In this case, obesity and sleep problems might cluster in certain workplaces. Stated differently, the workplace could be a target for interventions to prevent the co-occurrence of these two health problems. To date, it is not clear whether the workplace itself contributes to this co-occurrence. The specific question of interest is whether workplaces that have high rates of overweight employees are also the same ones that report high rates of sleep problems; that is, do these health problems cluster in the workplace?
To this end, we analyzed data from over 3000 Finnish public sector workplaces and their 40 000 employees who responded to a survey addressing work-related factors and health issues. As employees may be influenced by their social environment at work, we used multivariate, multilevel analysis, that is, models with multiple outcome variables, in this case obesity and sleep problems. This approach enabled us to determine (i) the correlation between obesity and sleep problems; (i) the extent to which the correlations depend on the individual and the workplace level; and (iii) the joint effects of workplace- (eg, distribution of employees) and individual-level factors (eg, socioeconomic status, work stress) on obesity and sleep problems (25–27).
Methods
Participants and design
Data were obtained from the Finnish Public Sector Study, an ongoing cohort study of a large sample of public sector employees involving all personnel in 10 municipalities and 21 hospitals in Finland (21). The Ethics Committee of the Finnish Institute of Occupational Health and the Hospital District of Helsinki and Uusimaa approved the study. The eligible population for this study comprised the 48 592 employees who responded to the survey in 2000–2002 (response rate 68%). We excluded 6908 participants with missing information on obesity, sleep problems, workplace social capital, or other covariates. We identified each participant’s work unit at the lowest level of organizational hierarchy, such as a kindergarten or a hospital ward, from employers’ records. We included only individuals from work units with ≥3 participants, resulting in a final sample of 39 873 employees nested in 3040 work units. Of the participants, 81% were women, 18% manual workers, and the mean age was 44.3 (range 18−63) years.
Outcome measures
Obesity
We used self-reported height and weight to calculate body mass index (BMI) with a BMI of ≥30 kg/m2 indicating obesity (1).
Sleep problems
We assessed sleep problems using the 4-item Jenkins Sleep Problem Scale (28). Participants reported the frequency of difficulties initiating sleep, waking up several times per night, awakening too early in the morning, and non-refreshing sleep over the past 4 weeks on a scale of 1−6 (1=never to 6=every night). Those who reported any of the 4 sleep problems on 5–7 nights a week were coded as having frequent/severe sleep problems (29, 30).
Explanatory variables
Individual characteristics
We obtained sex, age, and socioeconomic status (SES) from employers’ records. SES was assessed using the occupational-title classification of Statistics Finland: (i) high SES (upper-grade non-manual workers, eg, physicians, teachers), (ii) intermediate SES (lower-grade non-manual workers, eg, technicians, registered nurses), and (iii) low SES (manual workers, eg, cleaners, maintenance workers) (31). Standard questionnaires were used to assess heavy drinking (>210 g/week versus less) (32), smoking status (current versus ex-/never), and low physical activity [<2 metabolic equivalent task (MET)-hours per day versus more] (33). Self-rated health was used to measure health (very good or good versus average or less).
Work-related variables
We obtained the types of job contract from employers’ records (permanent versus temporary). We used survey responses to measure workplace social capital, job strain, and shift work. Workplace social capital was defined as the shared values, attitudes and norms of trust and reciprocity as well as practices of collective action in their work unit. We used a psychometrically tested 8-item measure (34), shown to be associated with depression and mortality (35, 36), to assess workplace social capital. Job strain was calculated from items addressing job control and job demands (the means of job control scores subtracted from the means of job demand scores) (37, 38). Social capital and job strain scores were divided into tertiles. Information about the work schedule was dichotomized to assess shift work (shift work with or without night shifts) in contrast to day work. Beyond these individual factors, we objectively assessed work-unit-specific variables from the employers’ annual records of work-unit composition for the survey year. From these data, we calculated the proportion of manual workers and temporary workers in each work unit. Both were divided into low versus high using the median value (5% and 25%, respectively).
Statistical analysis
We followed the multivariate, multilevel approach introduced by Goldstein (25), in which multiple binary outcomes are jointly regressed over a set of explanatory variables. In the multivariate, multilevel statistical framework, individual measurements of obesity and sleep problems were treated as repeated measures from the same individual and the lowest level of hierarchy. The within-individual measurements (obesity and sleep problems) were level 1 units; 39 873 participants were level 2 units; and the 3040 work units were level 3 units. No level 1 variation was specified because level 1 existed solely to define the multivariate structure. The level 2 variances and covariance were the between-individual variances, and the corresponding measures at level 3 were those between work units. In the models, each individual had two outcomes (obesity and sleep problem); dummy variables indicated which outcome was present (for example, obesity=0 and sleep problems=1). Explanatory variables were defined by multiplying these dummy variables by individual and work-related factors (25). These interactions enabled us to examine whether the explanatory variables were differently associated with obesity and sleep problems.
We fitted multivariate 3-level logistic regression models for sleep problems and obesity, modeled simultaneously as a function of fixed individual- and work-unit-level factors and random effects (individual, work unit) (39). All statistical analyses were performed using the GLIMMIX procedure in SAS© 9.2 statistical software (SAS Institute Inc, Cary, NC, USA). We started the multivariate, multilevel modeling with a null model (model 1) to examine the between-individual and between-work-unit variances and covariance in obesity and sleep problems. Covariance is a measure of how much these outcomes change together in the same direction. If the outcomes show similar behavior, their covariance is >0, and, if the variables are independent, their covariance is 0. We then fitted 3 sequential models to study how much of the variation and covariation in obesity and sleep problems was accounted for by the individual characteristics (model 2) and work-related variables (model 3). The results showing the effects of individual and work-related factors on the outcomes are from the full model including all covariates (model 4), and they are expressed as odds ratios (OR) and their 95% confidence intervals (95% CI).
Results
Participant characteristics are shown in table 1. The prevalence of obesity and self-reported sleep problems was 11% and 23%, respectively. Of the obese participants, 30% reported sleep problems. Thus, the prevalence of the co-occurrence of obesity and sleep problems was 3% at the individual level. Obese participants were working in 1945 work units (64% of all work units). In 91% of them, employees also experienced sleep problems. The prevalence of the co-occurrence of obesity and sleep problems was 58% at the work-unit level.
Table 1
Characteristics of 39 873 participants and 3040 work units, Finland, 2000–2002. [SES=socioeconomic status; BMI=body mass index]
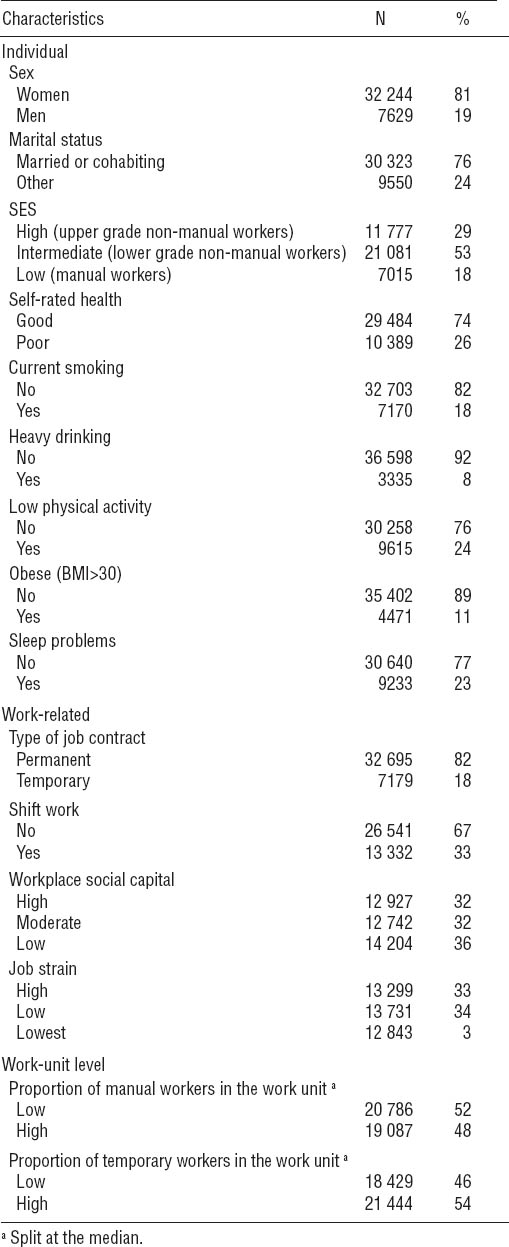
Table 2 presents the results of the fixed part of the multivariate, multilevel models conditional on random effects associated with work units. In a model including all covariates simultaneously, increasing age was positively related to obesity and sleep problems. Male and married employees were less likely to report sleep problems, whereas no differences were found for obesity. Employees in low and intermediate SES groups had an increased risk of obesity but a lower risk of sleep problems than their counterparts in the highest SES group. Poor health was the factor most strongly associated with both obesity and sleep problems increasing their odds to 2.1−2.9 fold. Heavy drinking and low physical activity were associated with an increased risk of both outcomes Of work-related factors, only job strain was associated with both outcomes. High job strain was related to a lower risk of obesity (OR 0.90, 95% CI 0.82–0.98) but was associated with increased odds of sleep problems (OR 1.78, 95% CI 1.67–1.90). Those employees who worked shifts during the time of the survey reported fewer sleep problems than day workers. Employees with low workplace social capital had a 24% higher likelihood of experiencing sleep problems. Type of job contract was not related to either outcome. At the work-unit level, a high proportion of manual workers but not temporary workers in the work unit slightly increased the odds of obesity but not of sleep problems.
Table 2
Odds ratios (OR) from fixed part of a multivariate 3-level logistic model for individual- and work-unit-level risk factors.a [95% CI=95% confidence interval; SES= socioeconomic status]
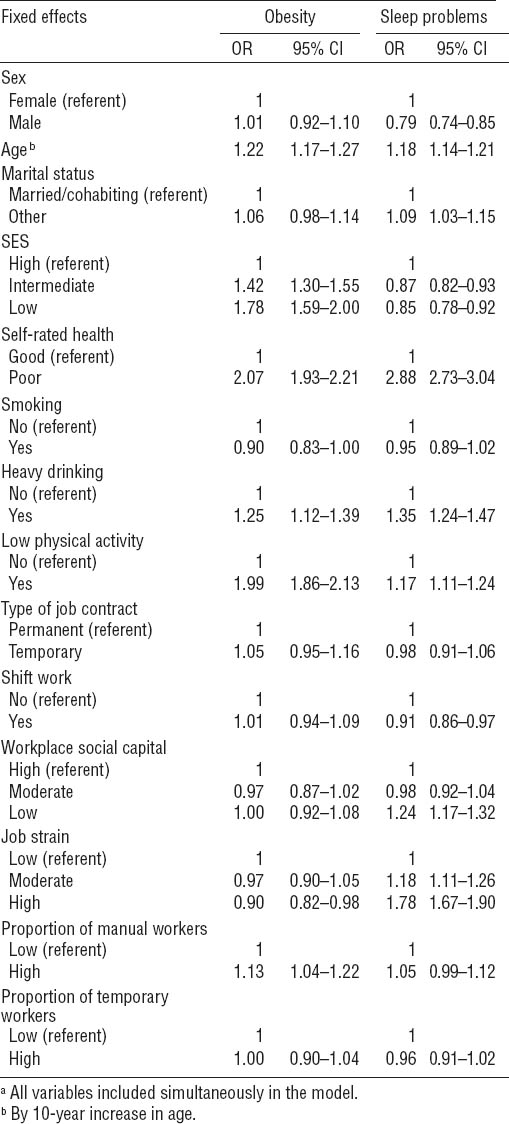
Table 3 demonstrates how obesity and sleep problems varied across individuals and work units and shows their correlation at both levels. We found a positive correlation between obesity and sleep problems at the individual level [correlation coefficient 0.047; covariance 0.048, standard error (SE) 0.005]. The association was attenuated by 83% when individual characteristics were added to the model (model 2), and, in this model, their covariance was no longer different from 0 which describes their independence at the individual level. When work-related factors were also added to the model (model 3), the correlation decreased by only 6%.
Table 3
Variation and covariation in sleep problems and obesity at the individual and work-unit level from the random part of multivariate 3-level logistic model. [SE=standard error]
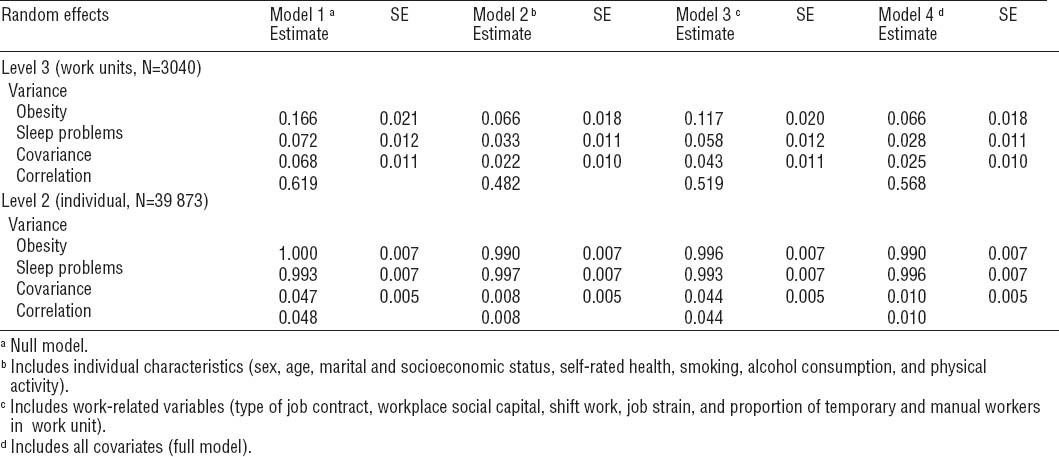
Table 3 further shows that we observed a more pronounced correlation between obesity and sleep problems at the work-unit level (correlation coefficient 0.619; covariance 0.068, SE 0.011). When individual characteristics were included in the model, the between-work unit variance in obesity decreased by 60% (variance 0.166 versus 0.066). The corresponding reduction in the clustering of sleep problems attributable to individual characteristics at the work-unit level was 54% (variance 0.072 versus 0.033). Workplace-related factors accounted for 30% and 19% of the associations, respectively. The correlation between obesity and sleep problems at the work-unit level remained in all model specifications; after controlling for all individual and work-related variables, the correlation coefficient was 0.568 (covariance 0.025, SE 0.010).
Discussion
We used a multivariate, multilevel approach to investigate the variation and covariation in obesity and sleep problems in a large sample of public sector employees and work units in Finland. We found a correlation between obesity and sleep problems both at the work-unit and individual level, suggesting not only that obese employees are more likely to suffer from sleep problems, but also obesity and sleep problems tend to cluster in the same workplace as indicated by increased proportions of employees with a BMI of ≥30 kg/m2, sleep problems, or both. Although we could not identify any specific patterns regarding the workplace factors that could be attributable to the co-occurrence, these findings suggest that such factors exist.
Some limitations should be borne in mind when interpreting our results. First, the assessment of both obesity and sleep problems were based on self-report and, thus, their co-assessments may have been influenced by a joint underlying factor, such as reporting style or other source of common method variance. Unmeasured conditions that have been associated with both obesity and sleep problems, such as chronic pain syndromes limiting physical activity as well as interfering with sleep, could bias the estimates of the correlation between obesity and sleep problems. However, this is unlikely to explain our findings, because some explanatory variables were differently associated with these outcomes and, more importantly, the individual-level correlation of sleep problems and obesity was less robust than that at workplace level. Still, we cannot totally exclude the possibility of residual confounding. Second, the cross-sectional nature of the study does not allow any causal interpretation of the results. Third, the prevalence of obesity was low compared to the average population-level estimates (5), potentially reflecting the healthy-worker effect or underreporting of weight in surveys compared to direct measurements (4). However, the prevalence of obesity is lower in Finland than in several other countries, for example in the USA or the UK (3, 4); other countries with higher prevalences of obesity may observe different covariances. Fourth, the fact that the correlation between obesity and sleep problems was more robust at the work-unit than the individual level suggests that our covariates covered contributing factors to a larger extent at the individual than at the workplace level. Fifth, the study population consisted predominantly of female public sector employees, which may compromise the generalizability of the results to male-dominant working populations. However, the study population is representative of the Finnish public sector employees in general; female-dominated occupations such as nurses and teachers comprise a large proportion of public sector employees in Finland. Finally, the data used are ten years old. The results might be different today because the prevalence of obesity and sleep problems has further increased.
Regardless of these limitations, however, this study also has a number of strengths, including the use of a multivariate, multilevel approach to identify the individual and work-unit-level determinants of obesity and sleep problems and their variance and covariance across individuals and work units. Using a 3-level model, we were able to partition the random variations onto work units and individuals, thereby increasing confidence in the inferences drawn from the data. Furthermore, the large number of individuals and work units helped us to detect between-work-unit variability.
As seen in previous studies modeling obesity and sleep problems as single outcomes (3–6, 19–22), simultaneous modeling of these two health problems revealed that they shared several determinants, such as age, SES, and job strain although the associations were in opposite directions, and some associations did not fully repeat the previously observed patterns. For example, in contrast to a previous study (20), employees in lower SES groups reported fewer sleep problems than their counterparts in high SES groups. The explanation for this finding is unclear, although it is possible that higher occupational status is associated with longer working hours which, in turn, are related to sleep disturbances (40). Of work-related variables, only job strain was related to both outcomes. High job strain was associated with an increased likelihood of sleep problems, but unexpectedly those with high job strain had lower odds of obesity. A potential explanation is that the effect of work stress on weight gain may vary leading some employees to gain and others to lose weight (41, 42).
We applied a multivariate, multilevel model because this method allowed us to examine the co-occurrence of obesity and sleep problems at both the individual and the workplace level. One of the advantages of this model is that we can draw conclusions about the extent to which the correlations depend on each level (25, 27). We found a positive intra-individual correlation between obesity and sleep problems illustrating that a proportion of the variation in obesity and sleep problems was between individuals. However, the intra-individual correlation was heavily attenuated following control for individual characteristics and only slightly when work-related factors were taken into account. These findings reflect the importance of individual factors rather than workplace issues as correlates of the co-occurrence of obesity and sleep problems among employees. At the same time, a more pronounced correlation was observed at the work-unit level demonstrating that a larger extent of the variation in co-occurrence was between work units. Indeed, we found that obesity and sleep problems co-occur at the work-unit rather than the individual level affecting 58% of the work units but only 3% of the participants. From the workplace health promotion perspective, this result illustrates the greater scope for interventions targeting both obesity and sleep problems at the work-unit rather than the individual level. Our results are in line with those reported by Subramanian et al (26) who studied covariation in individual-level determinants of health and happiness across communities in 36 US states (26). In their study, the correlation between individual-level health and happiness was positive, but it was stronger at the community level, indicating that healthy communities are likely to be happy communities.
Importantly, the disparities between workplaces persisted after accounting for differences in an array of individual and work-related factors. This suggests that clustering of obesity and sleep problems occurs within workplaces as well as among individuals. In other words, obese employees and those with sleep problems are not always the same individuals although they tend to work in the same workplaces. However, because we could not identify any pattern regarding workplace factors, we cannot propose any specific factor that should be targeted. Further studies are needed to examine potential work-related factors that could play a role in the co-occurrence of obesity and sleep problems, such as long working and commuting hours. From the employer perspective, it would be important to identify whether economic issues such as the push for productivity in some workplaces compromise issues on the health-promotion agenda. Finally, from the epidemiological perspective, the next step is to investigate whether the clustering is specific to these particular health outcomes and public sector workplaces. These results can be important for healthcare and the employer in terms of costs reduction, productivity, and maintenance of employee health. Future studies should address the question of what should be done in the workplace to reduce obesity and sleep problem prevalence.
In conclusion, we found that workplaces with a high prevalence of employees with sleep problems were also likely to be workplaces with obese employees, independent of the correlation of obesity and sleep problems between individuals. This suggests that differences between individuals did not explain the clustering of these two health problems in the workplace. Since the worksite is emphasized as an important venue in which to address multiple individual risk factors (43), future studies should investigate whether, in addition to targeting individuals at risk, interventions to reduce obesity and sleep problems might benefit from identifying “risky” workplaces (ie, those with co-occurring obesity and sleep problems).