Productivity loss at work due to health problems represents a serious concern in today’s workforce. The Commonwealth Fund estimates approximately $260 billion in lost productivity for health reasons per year in the United States alone (1). As the workforce continues to age and chronic illness becomes more prevalent (2–4), concerns about the ability of workers to be fully productive are on the rise (5). There has been some debate about how to best measure productivity loss, but the general consensus is that productivity loss due to health problems includes absence from work while employed, reduced productivity when at work (presenteeism), and premature withdrawal from employment (6, 7).
Despite some consensus on what constitutes health-related work productivity loss, there is little information about how and when this problem develops over a working lifetime, the reasons for temporal variation in patterns of productivity loss, and how these patterns may differ in risk for subsequent loss of employment. Many studies on productivity loss have examined factors associated with cross-sectional differences or short-term changes in productivity loss (≤5 years) (8–13), economic costs associated with productivity loss (14–17), and associations with subsequent employment loss and/or early retirement (8, 18, 19). Some have explored more long-term (≥10 years) developmental patterns of productivity loss across one’s working career, but these studies have incorporated only a few observations over time or include only a limited range of jobs (such as municipal managers), and outcomes are often limited to subsequent at-work productivity or well-being (18, 20).
If distinct long-term temporal patterns of productivity can be identified, and these patterns differ in risk of subsequent work disability, this may create an opportunity for new interventions to prevent disability and improve productivity, well before permanent work limitations or work loss has occurred. Interventions before work disability actually occurs are more likely to have a positive impact than attempts to reverse long-term work absence that follows a prolonged period of declining work ability (21). However, the lack of predictive information on which workers are at risk is a barrier to effective interventions.
In the current study, we examined developmental trajectories of the probability of experiencing health-related productivity loss over a 20-year period from ages 25–44 years. The first goal of this study was to evaluate longitudinal data on self-reported productivity loss to determine whether distinct temporal patterns exist. More specifically, we aimed to identify multiple different trajectories for the probability of productivity loss over time to see if, in fact, multiple trajectories exist and, if so, to describe these patterns in terms of whether they show (i) a generally high or low probability of productivity loss over time, (ii) an increasing or decreasing probability of productivity loss, and (iii) at what age an increase or decrease in probability would occur. A second aim of the study was to examine (i) differences in personal and demographic characteristics among the trajectories, such as higher self-esteem and education, and (ii) whether differing patterns of experiencing productivity loss between the ages of 25–44 years are associated with different employment outcomes in midlife, including the number of weeks worked in a year and the number of hours worked per week. If different employment outcomes are found based on the trajectories for productivity loss, this may have implications for identifying individuals at risk of poor employment outcomes in midlife. A strength of this study is the availability of information spanning the majority of respondents’ prime working years, providing a unique opportunity to identify long-term developmental patterns of productivity loss. This study may help to detect high risk patterns for potential work disability later in life.
Methods
Participants
This study utilized data from the National Longitudinal Survey of Youth 1979 (NLSY79), which is sponsored by the US Bureau of Labor Statistics (BLS) (22). A total of 12 686 participants aged 14–22 years were first surveyed in 1979. Follow-up interviews with participants were conducted annually from 1980–1994, and then every other year starting in 1996. The data is publically available for all years up to 2010 when the participants were aged 45–53 years. In 2010, 7565 participated for a retention rate of 59.6% of the original sample. The NLSY79 gathers information about a variety of topics including demographic characteristics, employment characteristics, income and assets, education, and work limitations.
For the current study, we were interested in examining trajectories of productivity loss over a 20-year period during the prime working years, specifically ages 25–44 years. In the current study, we restructured the NLSY79 data so that participants would enter our sample at age 25 and be similar ages at each of the 12 waves. For the first wave, we utilized data from the appropriate interview year at which participants should have been 25, based on their age in 1979. Data were collected annually until 1994, so the first five waves in our sample represent the ages at which participants should have been 25–29 years. The data were collected every other year starting in 1996, and so data for waves 6–12 were used for 2-year age groups. For the outcomes associated with the trajectories of productivity loss, we used data from the first interview year at which participants were ≥45 years or older. Table 1 displays the year of data used for each wave in our sample based on the participant’s age in 1979.
Table 1
Representation of the survey year used to provide data for the respective wave and age range based on the respondent’s age in 1979. a
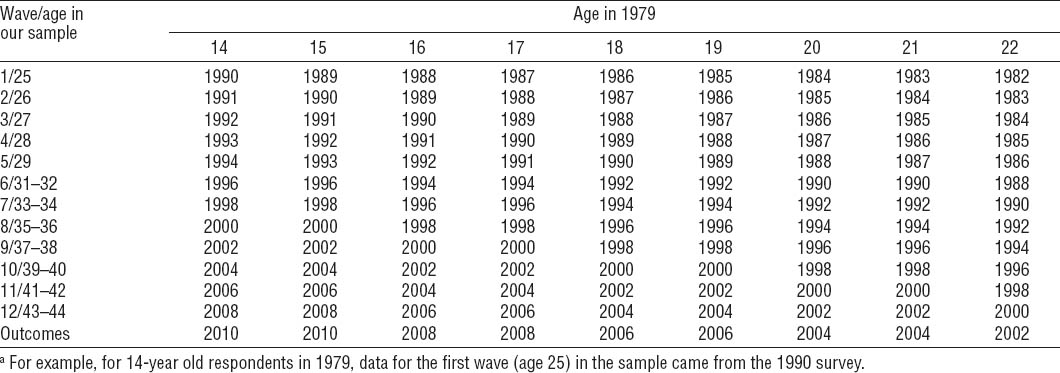
Within the 12 waves of data used for modeling the trajectories of productivity loss, we only included participants who had valid data for ≥6 waves and who participated in the interviews for wave 1 and for wave 12. This yielded a sample of 5699 unique cases for our trajectory model. Of the 12 686 participants in 1979, 4066 who did not have valid data in ≥6 waves were excluded from our study. An additional 2921 participants were excluded who did not participate in both waves 1 and 12. Additional information on the sample is included in the supplementary material (www.sjweh.fi/data_repository.php).
Measures
Productivity loss
The variables used to measure productivity loss trajectories were a composite of three items asked in each interview year: (i) “Would your health keep you from working on a job for pay now?”, (ii) “Are you/ would you be limited in the kind of work you (could) do on a job for pay because of your health?”, and (iii) “Are you/would you be limited in the amount of work you (could) do because of your health?” Prior to 2000, all participants, who reported that they were not working in the previous week regardless of whether they were employed or not, were asked the first question. From 2000 on, this question was only targeted at participants who reported that they were employed but not working in the previous week. All respondents, who were working the previous week or answered “no” to the first question, were asked the remaining questions. All respondents were asked at least one of the questions about productivity loss in each wave, and we did not observe any changes in the pattern starting after 2000. For all three questions, respondents were not asked what specific health condition was responsible for their productivity loss. For each wave, a single item was created which coded participants 1 if they reported yes to any of the three questions and 0 if they reported no and were working ≥10 weeks during the previous calendar year of the interview. In the trajectory variables, we omitted participants who reported that pregnancy was the reason for their productivity loss in a given wave. Across waves, the number of participants omitted due to pregnancy ranged from 1–115, with an average of 49 participants omitted per wave. Additional information on measure of productivity loss is included in the supplementary material (www.sjweh.fi/data_repository.php).
Baseline covariates
The baseline covariates were used to estimate the productivity loss trajectories. The trajectories were adjusted for gender and race/ethnicity. Gender was coded 1 for female and 0 for male. Race/ethnicity was coded 1 for white/non-Hispanic and 0 for non-white/Hispanic.
Trajectory characteristics
Several variables were used to characterize the trajectories of productivity loss. These variables were not included in the trajectory model but rather used to assess differences between the trajectories. The Pearlin Mastery Scale was assessed in 1992 using 7-items to measure self-concept and the extent to which people feel in control of their lives (23). Participants were asked how strongly they agree or disagree with each statement on a 4-point response scale ranging from 1=“strongly disagree” to 4=“strongly agree.” The items were averaged to create a total score with higher scores indicating higher mastery (α=0.79). The Rosenberg Self-Esteem Scale was assessed in 1980, 1987, and 2006 using 10 items measuring the self-evaluation (approval or disapproval) thatpeople make of themselves (α=0.83–0.87) (24). Participants were asked how strongly they agree or disagree with each statement on a 4-point response scale ranging from 1=“strongly disagree” to 4=“strongly agree.” We used the average of the three assessments for analyses. Higher scores indicated higher self-esteem. Socioeconomic index (SEI) was assessed at every wave with a valid occupation listed. For data from interviews between 1982–2000, the SEI was coded using Stevens & Cho’s (25) method for converting 1980 census occupational codes to SEI (26). For data from interviews between 2002–2008, the crosswalk provided by Frederick & Hauser (27) was used for converting 2000 census occupational codes to SEI (28). We used the average SEI across waves for analyses. Educational attainment was assessed at the last wave in our sample as the highest year of schooling completed (eg, 4th year of college). At the wave at which participants were first ≥45 years, we used measures of the number of weeks worked in the calendar year (1=≥10 weeks, 0=<10 weeks), the number of hours worked per week, and employment status. The hours worked per week measure included self-reported information about the actual number of hours worked for the entire previous calendar year and was calculated as the total number of hours worked per year divided by the number of weeks worked per year. As such, even if a person who reported having productivity loss that prevented them from working in the week prior to the survey may still have a value >0 for hours worked per week. We also assessed general mental and physical health using the SF-12 (29). The SF-12 involves two summary scores, for mental and physical health each, computed using norm-based scoring methods. Higher scores indicated greater mental and physical health. Participants completed the SF-12 in the first wave they participated in after turning age 40. Ideally this measure would have been asked at ages 40 or 41, but in some cases the age 40 module was completed later. All participants in our sample completed the module by age 45.
Statistical analysis
The analyses were conducted in two stages. First, the trajectory model was estimated using the user-written program “traj” for STATA (Stata Corp LP, College Station, TX, USA) (30). This program is used for implementing latent class growth analysis (LCGA), a type of growth mixture modeling (31). Compared to traditional growth curve analysis, which estimates a single trajectory, LCGA has the advantage of being able to identify distinct subgroups of individuals who follow similar developmental trajectories in a larger sample. We used the logit option to model the trajectories that plotted the probability of productivity loss across the age range in our sample. The productivity loss variables for each wave were used for estimating the trajectories. In addition, the baseline covariates were included in the model and adjusted for differences in these factors across trajectory groups.
Finding the best-fitting trajectory model is an iterative process. A series of trajectory models were estimated, testing for different numbers and sizes of the individual trajectories (eg, linear, quadratic, cubic, quartic). Bayesian information criterion (BIC) was used to determine the best-fitting model. The model with the lowest BIC was selected as the final model. In addition, we examined the number of respondents belonging to each trajectory and rejected models containing trajectories with <100 participants. Participants were assigned to the trajectory to which they had the highest probability of belonging, based on the maximum likelihood probability rule (32, 33). A new variable was created which categorized participants into the trajectory groups.
In the second stage of analysis, we examined differences in the trajectory characteristics across the productivity loss trajectory groups, using the grouping variable that was created based on the trajectory model. Analyses of variance (ANOVA) were used for the number of weeks worked and the number of hours worked per week in the outcome wave. ANOVA was also used to test for differences in general mental and physical health in the age-40-years module and mastery, self-esteem, education, and SEI. Chi-square tests (χ2) were used to test for differences in the proportion of respondents who were employed in the outcome wave. All analyses were conducted using STATA 13.1.
Results
Trajectory model for productivity loss
A five-group trajectory model for productivity loss was selected based on BIC values (figure 1). A good model fit is indicated by a posterior probability of 0.80 per trajectory group among those assigned to a given group (34). The mean probability was 0.80, 0.82, 0.94, 0.85, and 0.93 for those assigned to groups 1–5, respectively. All trajectories followed a quadratic shape. Trajectory 1 (ie, the low-risk group), accounting for 11.5% of the sample, was characterized by a relatively low and stable probability of productivity loss over time, although there was a slight increase up to around age 32, followed by a slight decrease thereafter. Trajectory 2 (ie, the late-onset increasing-risk group), accounting for 5.5% of the sample, was characterized by an initially low probability of productivity loss, with increasing probability beginning after age 35. Trajectory 3 (ie, the no-risk group), accounting for the largest part of the sample at 75.3%, was characterized by a stable zero probability of productivity loss over time. Trajectory 4 (ie, early-onset increasing-risk group), accounting for 3.7% of the sample, was characterized by an initially low probability of productivity loss followed by a quickly increasing probability beginning around age 30 years. Trajectory 5 (ie, the high-risk group), accounted for only 4.0% of the sample and was characterized by a relatively high probability of productivity loss over time, with a slight increase in the probability from 25 to 30 and relatively stable thereafter.
Baseline covariates
Our trajectory model was adjusted for gender and race/ ethnicity. Table 2 presents the proportion of each trajectory that was female and white/non-Hispanic. The low-risk group had the highest percentage of women (70.6%), while the no-risk group had the lowest percentage (43.4%). The no-risk group had the highest percentage of white/non-Hispanics (55.5%) in contrast to the high-risk group which had the lowest (46.0%). The early-/late-onset increasing-risk groups had similar percentages of women (46.5% and 47.3%, respectively) and white/non-Hispanic (48.4% and 49.2%, respectively).
Differences in the productivity loss trajectories
After identifying the productivity loss trajectories, differences in these groups were explored (see table 3). Regarding the percentage of years with productivity loss among the five trajectories, the high-risk group, exhibited the highest percentage (80.1%), followed by the early-onset increasing-risk group (47.6%). The late-onset increasing-risk and the low-risk groups had relatively low percentages of years with productivity loss at 27.3% and 17.7%, respectively. The no-risk group had the lowest percentage of years with productivity loss (<1%).
Table 3
Differences in the productivity loss trajectories. [SD=standard deviation; SEI=socioeconomic index.]
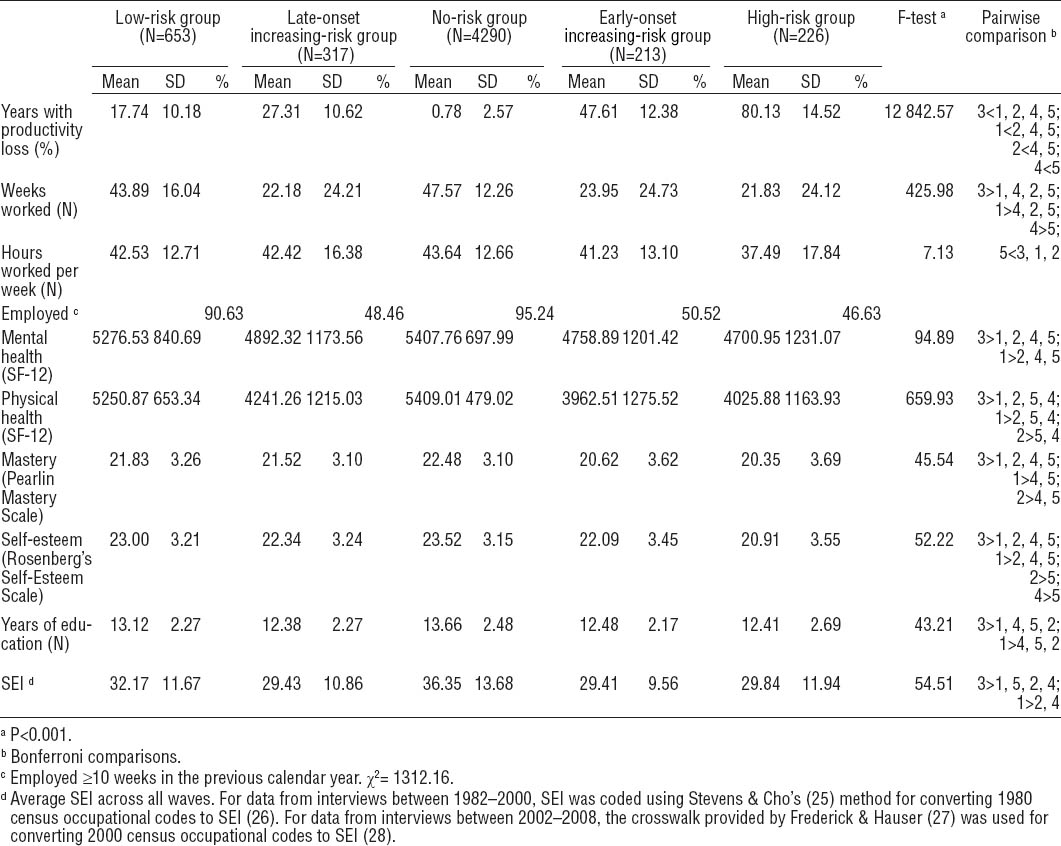
For the number of weeks worked in the year before the final survey, the no-risk group worked a high of 47.6 weeks, compared to 43.9 weeks for the low-risk group, 22.2 weeks for the late-onset increasing-risk group, 21.8 weeks for the high-risk group, and 24.0 weeks for the early-onset increasing-risk group. The high-risk group not only worked fewer weeks, it also worked fewer hours per week (37.5 hours) than the other groups. The no-risk group worked the most hours per week at 43.6 hours. Regarding general employment status in the last survey year, almost the entire no-risk group (95.2%) in the outcome wave was employed for ≥10 weeks during the previous calendar year compared to only 46.6% of the high-risk group, 50.5% of the early-onset increasing-risk group, and 48.5% of the late-onset increasing-risk group.
For both physical and mental health, the best outcomes were observed in the no-risk followed by the low-risk group; the worst outcomes were observed for the early-/late-onset increasing-risk groups and the high-risk group. For mastery and self-esteem, the no-risk group consistently had the highest levels, the low-risk and late-onset increasing-risk groups had similar levels in the middle, while the early-onset increasing-risk and the high-risk groups consistently had the lowest levels. For self-esteem, however, the high-risk group was significantly lower than the early-onset increasing-risk group. Both education and SEI were the highest for the no-risk group, followed by the low-risk group, whereas the remaining three groups were all lower on average.
Discussion
In the current study, we examined trajectories of productivity loss over a 20-year period using a secondary analysis of the NLSY79. The first goal of this study was to evaluate longitudinal data on self-reported productivity loss to determine whether distinct temporal patterns exist. To our knowledge, this study is the first to use a person-centered analytic technique, specifically latent class growth analysis, to investigate productivity loss longitudinally. Consequently, we were able to gain a more in depth understanding of the many developmental paths of productivity loss across the prime working years (ages 25–44) and explore differences among these trajectories.
We identified five unique trajectories of productivity loss among participants. For the large majority of participants (three-quarters of the sample), the probability of productivity loss over time was approximately zero. An additional one-tenth of the sample had a relatively low probability of productivity loss over time, although it was notably higher than in the no-risk group. The remaining participants (approximately 15% of the sample), were split across three groups. About 4% of the sample experienced a sharp increase in their probability of productivity loss early, around age 30, whereas 6% of the sample did not experience the sharp increase until closer to age 40. The final group represented approximately 4% of the sample and had a relatively high probability of productivity loss over time with a slight initial increase from age 25 to 30 years.
Although no previous research has examined multiple trajectories of productivity loss, one study examined trajectories of work ability over a 10-year period and found that, for the majority of the sample, work ability remained excellent (18). A smaller portion of their sample had declining and consistently poor work ability, as did the high probability of productivity loss groups in our study. In another study examining sickness absence over a 7-year period, the authors also found the majority of the sample to be in the low-risk group, with a smaller portion being in a high-risk group, with a similar trend as observed here (35). Our findings regarding the relatively small percentage of participants who were in groups experiencing a high or increasing probability of productivity loss are in line with previous research, which showed that, by age 45, approximately 15% of adults had experienced a work disability for ≥4 years, while only 10% had experienced a work disability for 4 consecutive years (36).
A second goal of the present study was to examine differences among the trajectory groups in relation to personal and demographic characteristics, as well as differences in employment outcomes in midlife. When looking at the percentage of years from age 25–45 in which participants experienced productivity loss, the high-risk group had, by far, the highest rate in 80% of the years. This was almost double the percentage for the next highest, the early-onset increasing-risk group, who experienced productivity loss in an average of half of the years. The late-onset increasing-risk group experienced productivity loss in approximately a quarter of the years and the low-risk group only experienced productivity loss in <20% of the years. Not surprisingly, the no-risk group experienced productivity loss in less than 1% of the years.
For the personal and demographic characteristics, we found similar distributions by gender and race/ ethnicity within the trajectories, but the low- and highrisk groups had a greater percentage of females, and the no-risk group had a greater percentage of white/nonHispanic respondents than any of the other trajectory groups. Not surprisingly, the no-risk group presented the highest levels of education, mastery, and self-esteem, along with the highest average SEI. The low-risk trajectory, in which respondents had relatively few waves with productivity loss, had higher average levels of all the characteristics explored than any other trajectory group and was most similar to the no-risk group. The other three trajectories (the high-risk group, early-onset increasing-risk, and late-onset increasing-risk groups) were fairly similar with regards to mastery, self-esteem, education, and SEI with a few exceptions. The late-onset increasing-risk group had higher mastery than both the high-risk and early-onset increasing-risk groups and the high-risk group had lower self-esteem than all other trajectory groups. Our results are in line with previous research suggesting that productivity loss and work disability are lower among those with higher education (11, 14), greater personal/psychological resources (37–39), and higher SEI (40).
For the outcomes in midlife, we found that the highrisk, early-onset increasing-risk, and late-onset increasing-risk trajectories had the lowest number of weeks worked, compared to the no- and low-risk trajectories. In fact, the no- and low-risk trajectories worked approximately double the number of weeks as the other three trajectories. In addition, the high-risk group worked the fewest hours per week relative to the other groups. Similarly, a large majority of both the no- and low-risk trajectories were employed ≥10 weeks during the previous calendar year at the outcome wave, compared to only about half of the high-risk, early-onset increasing-risk, and late-onset increasing-risk trajectories. In line with previous research showing a relationship between work disability and health (12, 41, 42), the no- and lowrisk group had the highest levels of mental and physical health at age 40, whereas the high-risk and early-onset increasing-risk groups had the lowest. Additionally, for physical health, the late-onset increasing-risk group reported better physical health than both the high-risk and early-onset increasing-risk group.
Implications
The findings of our study have several implications for identifying individuals at risk for work disability over time. Our results suggest that workers experiencing just one or two periods of productivity loss before the age of 45 might not be indicative of an early withdrawal from the labor force. However, workers who experience recurrent productivity loss early on in their careers may be at risk of being unable to work up to the age of 50 and beyond, and these workers may be an important group on which to focus in order to prevent permanent employment withdrawal.
We found quite a few factors that differed across the trajectory groups that may help to explain some of the productivity loss patterns and the risk factors for recurrent productivity loss. Based on our findings, those in the trajectories associated with the lowest employment participation at the outcome wave had lower education, socioeconomic status, and levels of personal resources compared to the groups with the highest employment participation. It is likely that workers with lower education and socioeconomic status find themselves in more physically demanding jobs where work is more likely to be limited by health conditions (43). In these types of jobs, accommodations to facilitate continued work participation may be important for workers experiencing productivity loss early in their careers. For personal resources, these findings may reflect that individuals with higher levels of self-esteem and mastery have better health and so they are less likely to experience productivity loss. However, an alternative is that individuals with higher levels of mastery self-select into work that fits well with their personal needs and abilities, and so individuals with health conditions that may cause productivity loss in certain lines of work may choose other careers to avoid experiencing productivity loss. Also, when faced with some type of productivity loss, these individuals may be better able to deal with their health limitations without needing to withdraw from the labor force.
Another implication of our findings deals with the timing of experiencing productivity loss. Our trajectory model revealed consistently low- and no-risk groups as well as a consistently high-risk group, but we also found two increasing-risk groups. The early-onset increasing-risk group began experiencing an increase in the probability of productivity loss by age 30, whereas the late-onset increasing-risk group did not experience an increase until closer to age 40 years. At the outcome wave, the late-onset group had greater physical health than the early-onset group. Although we do not have information on the specific health conditions causing the productivity loss at each wave, our findings suggest that general health may be worse in midlife for those who experience a condition or conditions leading to productivity loss earlier in adulthood compared to those who experience the condition(s) later in adulthood. It is important for future research to address whether the health conditions in these two trajectories are, in fact, different and what implications this may have for a person’s ability to deal with the health conditions.
Strengths and limitations
There are several strengths in the current study which include the use of longitudinal data, with multiple observations over a 20-year period in a large, national US sample with high retention rates. To our knowledge, this publically available data has not been used previously to examine general work disability and productivity loss. Our study is also unique in its focus on categorizing multiple trajectories of productivity loss. While there has been previous longitudinal work on productivity loss, a group-based modeling approach is not seen in the literature. This innovative method allows the identification of trajectories that characterize smaller portions of the total sample but that represent significant developmental patterns.
Despite these strengths, there are a few important limitations to acknowledge. As with any secondary analysis, there were challenges associated with using data that have already been collected. First, the survey in the NLSY79 did not ask questions about what specific conditions were causing the productivity loss. As a result, it is not possible to identify whether the same condition was responsible for the productivity loss in multiple waves for an individual or if certain conditions were more prevalent across one trajectory compared to another.
Second, the questions for the different types of productivity loss were asked about current work limitation. It is possible that people may have been limited in work for 30 weeks in the year but were fine at the time of the interview and so in that respective wave, they would not be considered to have productivity loss. Similarly, people may have just been limited in the week in question but were fine for the rest of the year; however, they would still have been considered to have productivity loss. To see whether these questions seemed to be predictive of actual productivity loss, we examined the total number of weeks worked in a given year to see if it differed based on whether participants reported productivity loss in that year. As expected, we found that the participants reporting productivity loss in a given wave reported working less than half the number of weeks in the previous calendar year for the respective wave compared to those reporting no productivity loss. Thus, the items used in our study do seem to be a reasonable surrogate for productivity loss for the previous calendar year for a given wave.
Third, there is no way to disentangle the two constructs of expected productivity loss from actual productivity loss. If participants reported that they were not working in the week prior to the interview, they were asked if health would keep them from working, which relates more to expected productivity loss. In contrast, if participants were working or reported that health would not fully keep them working, they were asked whether they are or would be limited in their work by health, which combined actual productivity loss with expected productivity. This is one of the limitations of the data.
Fourth, we do not have information on the frequency or severity of productivity loss within years. This is a limitation of using data that has already been collected. In our study, people were considered to have productivity loss if they reported that, in the week prior to the interview, health would prevent them from working or that health was or would limit the amount or kind of work they could do. It is possible that some people reporting productivity loss had a minor limitation to their work while others had very significant productivity loss which prevented any type of work. The experiences of individuals may vary greatly based on the severity or the frequency of productivity loss. Despite this limitation, having access to 20 years of longitudinal data is rare and so we feel that the analyses are still informative. Future research can aim to examine variations among the trajectories identified in this study based on both the frequency and severity of productivity loss throughout adulthood.
Finally, as we were examining employment status at age 45, we were interested in including information about participants who receive disability insurance, social security, or workers’ compensation. However, in the NLSY79, information on receiving these benefits was asked in reference to the participant and/or the participant’s spouse. For this reason, we were unable to disentangle who was actually receiving the benefit. Future research may try to address whether patterns of productivity loss over time are associated with receiving disability benefits prior to retirement age.
Concluding remarks
Productivity loss represents a serious concern for employers and employees. As the prevalence of chronic health conditions continues to rise in our population, understanding the patterns of health-related productivity loss across one’s working life is a topic of interest. The current study identified five unique trajectories of productivity loss. The no- and low-risk trajectories showed a low probability of productivity loss over time and did not have a high prevalence of early work withdrawal in midlife. The high-risk and early-/late-onset increasing-risk trajectories showed initially high or increasing probabilities of productivity loss and were related to being out of work in midlife. In addition, these three trajectories showed lower levels of personal resources than the no- and low-risk trajectories, suggesting they may be in need of intervention to prevent early-work withdrawal.