It is generally believed that biomechanical exposures at work (eg, lifting and trunk flexion) are important risk factors for the occurrence of low-back pain (LBP) (1, 2). However, the effects of these factors on LBP are insufficiently understood, and their impact on LBP has even been questioned (3, 4). Thus, the issue has been subject to intensive discussions, with research groups differing in their explanation of why the evidence on risk factors of LBP is ambiguous (1, 4–7). It has been suggested that one of the reasons for this ambiguity is that studies have differed in their strategies for collecting and modeling data on exposures and outcomes, including differences in the range of exposures represented in the study population. According to statistical theory in regression modeling, variance of the independent variable will have a significant influence on the ability of the regression to explain variance in the dependent variable. Therefore, the inclusion (or not) of groups with outlying exposures can be expected to affect the ability (power) of a study to identify an association between exposure and outcome. In addition to a statistical benefit of including groups with outlying exposures, such groups might also provide important information on the etiology of outcomes (eg, LBP), in particular if they show a deviating prevalence or incidence from that predicted on the basis of less exposed groups. This would, for instance, occur if the exposure–outcome relationship showed a steep increase in prevalence or incidence above a certain exposure threshold. While outlying groups with high physical loads may, thus, be important to include in an epidemiologic study for several reasons, they may be difficult to recruit (8).
Group-based exposure assessment approaches have been used since two decades in occupational epidemiology, particularly in studies of chemical exposures (9), but also in studies of biomechanical risk factors and LBP (10, 11). In a group-based approach, workers are classified into groups according to some common attribute, often their job or work tasks, and exposure is measured only among a selection of workers within each group. Subsequently, exposure estimates obtained from the measured workers (typically mean values) are assigned to all workers belonging to that group. These exposure estimates are used together with individual outcome data from all subjects in estimating exposure–outcome relationships. The group-based exposure assessment strategy is based on the assumption that workers within the same group have comparable exposures, ie, that groups are homogeneous in terms of exposure. At the same time, exposure variability between groups should be comparatively large, so that the exposure contrast between groups is substantial (12–14). If these properties are met, a group-based exposure assessment might lead to more stable exposure estimates compared to individual exposures measured over a limited time period, which typically suffer from considerable random measurement error (15). A regression of outcomes on group-based exposure estimates will therefore be less biased than a regression based on individualized data, in particular if groups are sufficiently large (16–19). Thus, in theory, exposure–outcome associations are stronger and more correct when based on a group-based approach than when using individual exposures (20). This advantage of the group-based strategy comes, however, at the price of an increased uncertainty of the regression coefficient (17, 21).
While, thus, groups with an outlying biomechanical exposure can be expected to have effects on the results of exposure–outcome analysis using regression statistics, the nature of this influence is not well understood. Therefore, in the current study, we aimed to assess the influence of the presence of an outlying group in analyses of exposure–outcome associations. We examined this issue using simulations based on a parent dataset from a prospective cohort study on trunk exposures and LBP. We particularly addressed the extent to which the characteristics of the outlying group (number of subjects, exposure, and outcome prevalence) would influence the strength and significance of exposure–outcome associations [in terms of the odds ratio (OR) and its confidence interval (CI), and study power]. While we acknowledge that several other factors beyond biomechanical exposures, including psychosocial working conditions and personal attributes, have also been shown to influence the difference in LBP prevalence between occupational groups (22), we have designed our study to examine the effect of groups with an outlying biomechanical exposure in univariate associations between exposure and LBP.
Methods
Population
Data for the present study were obtained from the Study on Musculoskeletal Disorders, Absenteeism, and Health (SMASH), which has been described in detail in previous publications (10, 23). In summary, a group of Dutch workers participated in physical exposure assessments at the workplace, followed by a three-year follow-up period during which self-reported LBP was assessed. The Netherlands Organization for Applied Scientific Research (TNO) ethics committee provided ethical approval for this study. Any identifiable subjects have provided their signed consent to publication and participants gave informed consent before taking part in the study.
Workers were recruited from 34 companies in the Netherlands representing several industrial and service branches, including metal, computer software, chemical, pharmaceutical, food and wood construction industries, as well as insurance companies, childcare centers, hospitals, distribution companies and road worker companies. Therefore, the study population included workers performing various tasks with a wide range of physical and mental workloads. At baseline, 1989 out of 2048 invited workers agreed to participate and questionnaire data on personal factors and work characteristics were obtained from 1802 (91%) of these workers. For these workers, video recordings were collected at the workplace on four randomly chosen occasions during a single work day. One video recording lasted for 5–15 minutes, with longer recordings for workers performing tasks that were expected to have larger exposure variability. On inspection of the videos, each single worker was allocated to an occupational group performing the observed tasks, as based on the International Standard Classification of Occupations (24). These occupational groups were then compiled into 19 groups expected to have comparable physical exposure, as judged by ergonomics experts (table 1). Thus, for this classification, information was available on neither the actual exposure of individual workers nor psychosocial and other workplace factorsnor LBP status. Within each of the 19 groups, video recordings were analyzed post-hoc in more detail from a random sample of roughly one fourth of the workers, using a structured continuous observation protocol (see below). After excluding workers who dropped out after the baseline measurements, the parent data set for the current study included 1131 workers from the 19 groups (table 1). Video-based observation data were available from 371 workers in total.
Table 1
Characteristics of the parent data set. In the upper part, the total number of workers and the number of workers observed are shown for each occupational group, together with low-back pain (LBP) prevalence with 95% confidence intervals (95% CI). Also group mean exposures [with standard deviations (SD) between workers] to the number of lifts at work per week and the proportion of time (%) spent with the trunk flexed >30° are shown. In the lower part of the table, pooled descriptive statistics (gender, length, weight, age, working hours per week, years of employment at the current job, and number of workers with LBP at baseline) are shown for all workers and those workers observed.
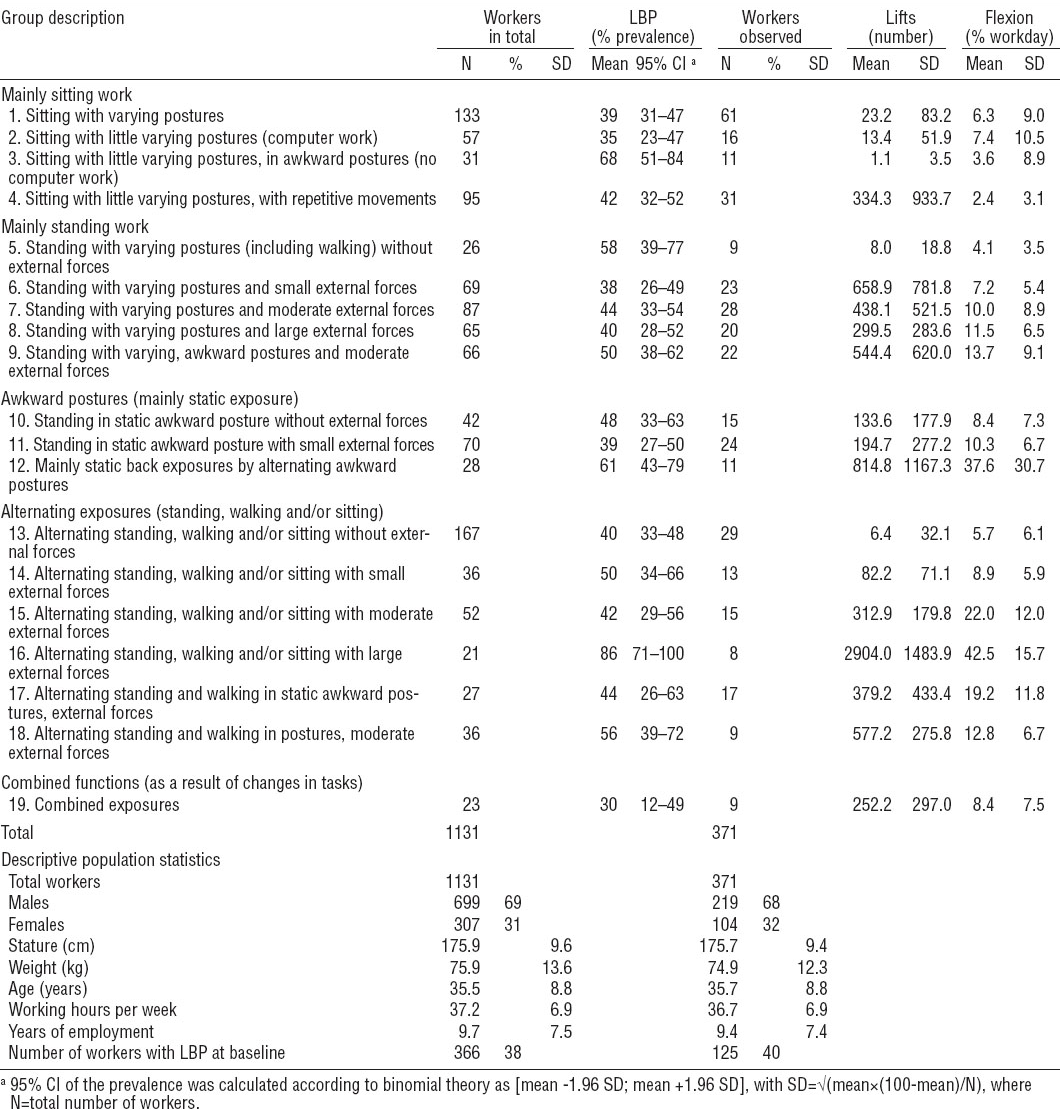
Exposure and outcome in the parent data set
During the structured observation protocol, two biomechanical exposures were assessed, which had previously been shown to be significantly associated with LBP in the same population (10); ie, the number of lifts during a 40-hour work week, and the percentage of working time with the trunk flexed >30°. Mean exposures of those workers observed in each of the 19 groups were assigned to all workers classified into that particular group.
After the baseline measurements, self-reported LBP was assessed for all 1131 workers annually during three years of follow-up, using a Dutch version of the Nordic Questionnaire (25). A case of LBP was registered when a worker reported regular or prolonged LBP during at least one of these three years, regardless of baseline status. Description of all groups as well as their physical exposure and prevalence are depicted in table 1. In this parent data-set of 1131 workers, exposure–outcome associations were pursued by logistic regression analyses, using the two exposure variables as continuous independent variables (in which the number of lifts were divided by 100 and percentages of time in trunk-flexed postures was divided by 10), and LBP as the dichotomous dependent variable. Confirming previous findings (10), both the number of lifts [per 100 lifts; OR 1.06 (95% CI 1.03–1.09), P<0.01] and the time working with the trunk flexed [per 10%; OR 1.31 (95% CI 1.12–1.52), P<0.01] were significantly associated with LBP.
Simulations
A first simulation was performed in which the effect of the presence of each of the exposure groups in the parent dataset was evaluated. Using a jack-knife procedure (26), each of the 19 groups was removed from the cohort, one after the other, and logistic regressions were conducted on the 18 remaining groups as described above. This procedure clearly showed that the presence of group 16 was crucial for the association between exposure and LBP (figure 1). When this outlying group was left out, the OR point estimate clearly decreased (although it was still >1) and the exposure–outcome association became non-significant (P>0.05). This particular group of workers performed tasks defined as “alternating standing, walking and/or sitting with large external forces”, and consisted mainly of road workers.
Figure 1
Jack-knife analysis of the influence of each of the 19 occupational groups on the odds ratios (OR) of the association between low-back pain and the two exposure variables number of lifts (upper panel) and time with the trunk flexed >30° (lower panel). The groups were ranked by magnitude of exposure and are labeled according to their description in table 1. The thick solid line indicates the OR point estimate, with the grey area illustrating its 95% confidence interval (95% CI). Dashed horizontal lines mark the OR with 95% CI for the exposure–outcomes associations in the entire parent data-set.
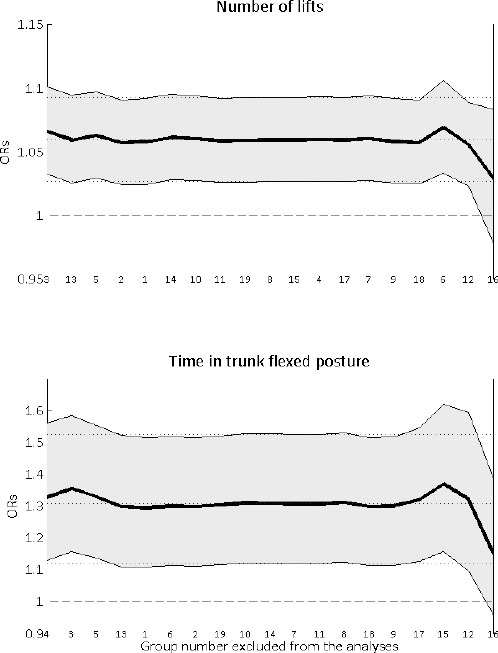
After identifying the group of road workers as being particularly influential on the eventual exposure–outcome association, a second simulation was performed, in order to assess the effects of changes in the size, exposure and LBP prevalence of this outlying group on the exposure–outcome relationship. The parameters for this simulation were selected on the basis of an inspection of the forms and parameters of the distributions of estimated exposure and outcome in the outlying group in the parent dataset, so as to represent a likely range of true exposure and outcome values for this group. Thus, using the estimated mean values and dispersions shown in table 1, we chose three likely true group mean exposure (e) values for lifts and trunk flexion (ie, e=2000, 3000, 4000 lifts per week and e=30, 40, 50 percent time with the trunk flexed). Also we chose four likely true prevalences (p) of LBP (ie, p=70, 80, 90, 100%). Using these values, simulation of results for individual workers proceeded as described in detail below. The simulated number of subjects (n) in the outlying group was selected to reflect a feasible range in a field study of the size of the original SMASH study (ie, n=4, 8, 16, 32, 64, 128). For each of the 6(n)×3(e)×4(p)=72 combinations of parameters, virtual studies were constructed for each of the two exposures using the following procedure:
A virtual outlying group including n subjects was formed. One fourth of these n subjects received a personal exposure by randomly selecting a value from a rectangular distribution with mean value e and range 2e; ie, a distribution with equal probabilities of obtaining any value between 0 and 2e. The choice of n/4 subjects actually being observed corresponded to the exposure sampling plan in the parent SMASH study. The mean exposure value across these virtual subjects with personal exposure data was assigned to all n subjects, in agreement with the group-based strategy used in the parent study.
Each of the n workers received a LBP outcome (“yes” or “no”), obtained from a binomial distribution with probability p of obtaining the answer “yes”. Outcomes were assigned to workers independent of their individual exposures; the group-based approach does not include any a priori assumptions on the nature of exposure–outcome associations within groups, and truly individual exposures are only available for a fraction of the whole population.
The virtual outlying group, with its simulated size, exposure and outcome, was entered into a logistic regression as that described above, together with all other 18 original occupational groups, all of which were retained from the parent data set without any modifications. OR with 95% CI for this regression was noted.
This procedure was repeated 3000 times for each combination of n, e, and p. Statistical properties of each combination were then assessed in terms of the mean OR across the 3000 virtual studies, the mean lower limit of the OR 95% CI, and the proportion of the 3000 studies with an OR significantly (P<0.05) differing from 1 (ie, the power of the study design to detect a significant exposure–outcome relationship at a P<0.05 level). All calculations were performed using custom scripts in Matlab (MATLAB 7.7.0, The MathWorks Inc, Natick, MA, USA). Logistic regression analyses were implemented using the Matlab statistics toolbox.
Results
As described in the methods section, the jack-knife procedure clearly pointed out the group of road workers (group 16 in table 1) as being particularly influential. When it was omitted, the OR decreased and, while still >1, it was no longer significant at a P<0.05 level (figure 1). This group had the largest mean exposure of all groups, and also the largest prevalence of LBP (table 1; figure 2). Between-group exposure variability, expressed by the root mean squared deviation (RMSD) between groups was 688.4 (lifts) and 15.6 (% time in trunk flexion) when the road worker group was included in the population, while it decreased to 348.7 and 13.2, respectively, when it was excluded. Therefore, in the second simulation, the effects of the characteristics of this specific group were explored.
Figure 2
Scatterplot of low-back pain prevalence at follow-up against the mean exposure of each of the 19 groups at baseline [ie, number of lifts (upper panel) and time with the trunk flexed >30° (lower panel)]. The point size for any particular group is proportional to the number of subjects within that group.
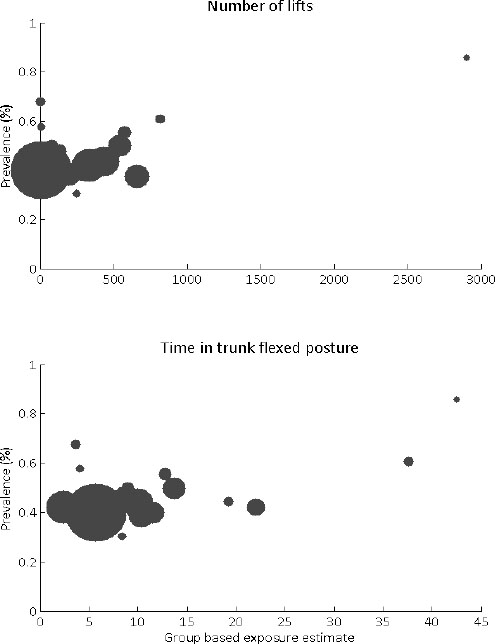
The point estimate of the OR (table 2) decreased towards 1 when either group size, exposure, or LBP prevalence decreased for the outlying group. Increasing values led to an increase in the lower boundary of the OR confidence interval, which in most cases was >1, while it was <1 when the outlying group was not included (N=0; figure 1 and table 2). Thus, statistical power was generally high in studies in which this group was present (table 3). Depending on the magnitude of exposure and prevalence, a minimum of 6–25 workers need to be included in this group to reach a power of 0.80. Thus, as long as the outlying group is included in the study population, the study will often be able to identify a significant exposure–outcome association, even if that outlying group is not very large. The OR, its confidence interval, and power were more sensitive to changes in group size and LBP prevalence than to changes in exposure magnitude. This is shown in table 2, where the LBP prevalence and especially the group size of the outlying group had a clear impact on the OR (both the point estimate and lower boundary of the confidence interval). Changes in the exposure of the outlying group (within the limits tested in the present simulation) only caused minimal changes in OR. Table 3 shows a similar effect for power, in being clearly affected by the size and LBP prevalence of the outlying group, but much less so by its exposure.
Table 2
Point estimate of the odds ratio (OR) and the lower boundary (LB) of its 95% confidence interval (95% CI) for the association between exposure and low-back pain (LBP) as affected by size, exposure, and LBP prevalence of the outlying group. Highlighted cells show LB 95% CI values >1.00. For reference, the OR (and 95% CI) in the parent data set was 1.06 (1.03–1.09) for lifts and 1.31 (1.12–1.52) for flexion.
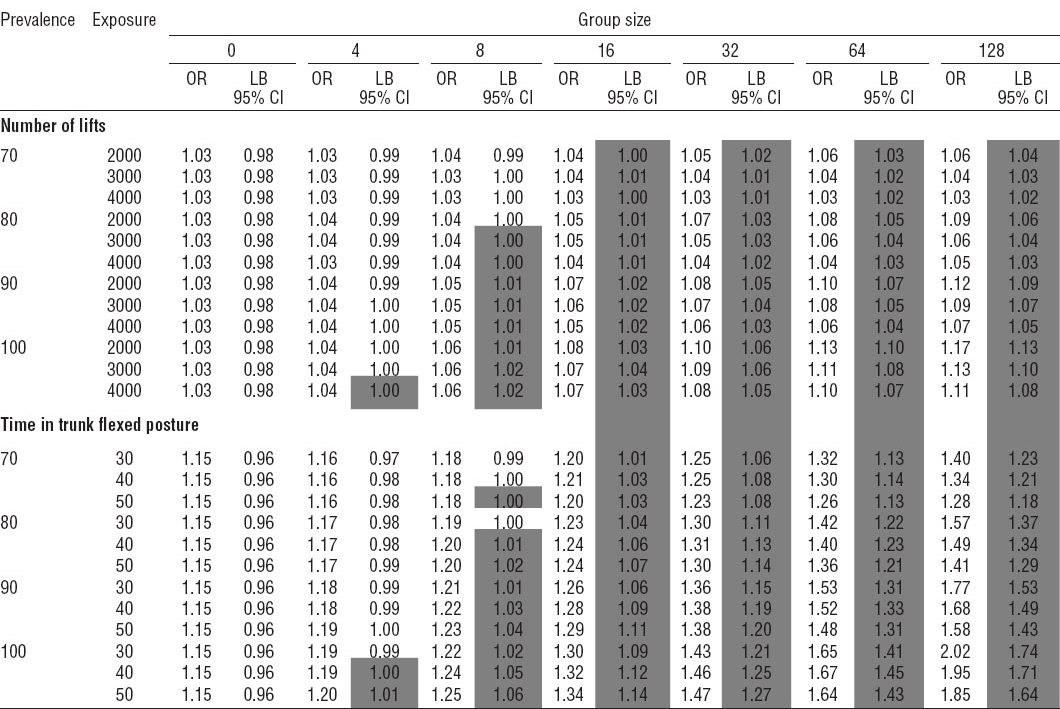
Discussion
Interpretation of results
This study showed that the presence of a single group of workers with outlying high exposures and high LBP prevalence can profoundly influence the exposure–outcome association and its significance in a group-based epidemiologic study, even if the far majority of a cohort does not belong to this group. Thus, our results suggest that it is of decisive importance to design studies that include groups with a broad range of exposures and outcome prevalences, in order to secure sufficient statistical power in detecting odds ratios that are not biased.
Our findings may appear trivial, since simple textbook power calculations (27) imply that a satisfying power in an epidemiologic exposure–outcome study relies on exposure variability being sufficiently large. However, our results illustrate that already as few as 21 outlying workers of the total 1131 workers (ie, 1.9%) can cause risk associations to change considerably. This remarkable effect of a small but influential outlying group could be one factor explaining the conflicting evidence that has been found for the effect of physical load on LBP in different studies (3, 4). Our findings point out that, in epidemiological studies on exposure–outcome associations, authors should carefully report results to the extent that the resulting exposure–outcome associations can be adequately interpreted by others. In addition, sensitivity analyses, such as the ones presented in this paper, would be a recommendable complement to basic results in research within this area. Moreover, a priori pilot measurements could guide researchers in how to effectively categorize subjects into groups so as to obtain a balanced cohort. An appropriate design including even groups with outlying exposures might, however, be difficult to accomplish due to practical obstacles, such as limitations in the number of companies available for the study, or the exposures of available workers within companies. Also, factors other than biomechanical exposures (eg, the psychosocial work environment and personal attributes, including socioeconomic status) play a role in the etiology of LBP, which further complicates the design of a balanced study.
Our results also contain an etiological message. As it appeared, including the group with an outlying, high exposure led to a change in the point estimate of the OR (expressed per unit of exposure). Only with this outlying group included, the OR differed significantly from 1. This might suggest that the investigated exposure–outcome relationships were non-linear, with only high exposures leading to a substantial increase in the prevalence of LBP; a possible explanation for this phenomenon being that exposures below a certain “safe” limit may increase or decrease without any noteworthy change in LBP risk. While this finding can have important implications for LBP prevention policies, it is important to note that while not significant, our data do suggest a small effect of low exposures on LBP as the OR point estimate was still >1 when leaving out the outlying group (figure 1). These results were based on simulations from an epidemiological study, ie, the SMASH study, where one occupational group showed a clearly deviating exposure (and outcome) from all other groups, and intermediate exposure groups were not included. Thus, our results cannot be extrapolated to epidemiological studies in general without caution. On the other hand, we emphasize that the SMASH population was intended to be a fairly representative selection of the Dutch work force, and that our case may thus represent a realistic exposure scenario, even if it does not exhibit a statistically optimal situation.
Non-linear associations between physical exposure and LBP have, indeed, been proposed before (11), and several non-linear models have been proposed to describe relationships between exposure and LBP, such as u-shaped associations (28) or spline regression models (20). Non-linear associations have also been implemented indirectly by transforming exposures, for instance using quadratic functions (29), fourth order weighting of loads (30) and polynomial transformations (31). An alternative explanation for the apparently non-linear association between biomechanical exposures and LBP might, however, be that other factors of importance to LBP (such as the psychosocial work environment or personal traits) may occur to a larger extent in occupations with large biomechanical exposures. Moreover, these factors might modify the effect of biomechanical exposures to different extents depending on the intensity of those exposures. We could however not pursue these explanations in the present material.
After having realized the profound influence of the road workers group, we assessed the effect of the characteristics of this group in terms of its size, exposure, and LBP prevalence, for a likely selection of parameters, as judged from the parent data. Reduced exposure and LBP prevalence in this group (ie, a smaller contrast between the group and the other occupational groups in the material) led to a smaller OR point estimate (table 2), which was also less likely to be significant (table 3), especially if the size of the outlying group was also small. LBP prevalence and group size appeared to be more influential than exposures in creating these effects.
The effect of increasing the size of the outlying group was most pronounced if the group was small; at larger group sizes the OR tended to stabilize, even though it did not approach any asymptotic value even with the largest of the investigated group sizes (N=128). These findings stress the importance of constructing cohorts with a substantial number of subjects in groups deviating considerably from other groups both in exposure and expected outcome. While the effects of changes in group size, exposures and LBP prevalence on OR point estimates and their CI were still very obvious with a large outlying group (table 2), study power saturated at group sizes >32 (table 3). These findings are important to bear in mind when interpreting previous epidemiological findings. Not only can the presence or absence of a single outlying group affect the ability (power) of a study to identify a significant association between exposure and outcome, but the magnitude of this group may also substantially influence the point estimate and lower boundary of the resulting OR.
Methodological considerations
The classification of workers into exposure groups used in the present data set was made by the same trained observers who also collected the video recordings, on the basis of their extensive experience in assessing physical workloads in occupational settings. As it appeared, the classification succeeded only partly in the primary objective of a group-based exposure assessment approach, ie, identifying groups differing markedly in exposure while, at the same time, exhibiting only limited exposure variability between subjects within each group (table 1). Thus, another categorization of some workers might have resulted in more homogeneous groups. This, in turn, could have influenced our results, since the grouping scheme is known to have effects on the outcomes of a study (32, 33), for instance in terms of its effectiveness in reducing attenuation of an exposure–outcome associations (34). Finding another grouping scheme with a larger overall difference between groups appears, however, in our case to be less likely, considering that very few subjects in the material showed to have exposures even close to those found in the outlying group focused in the present paper. However, a larger exposure contrast between groups due to a smaller within-group exposure variability could have affected both OR and power compared to the present design (12, 13), and thus even the observed effects of changing the characteristics of the most outlying group. However, the available material did not allow any further analysis of alternative grouping schemes.
Approximately one fourth of the total available population in each of the 19 groups was randomly selected to be observed as the basis for estimating group mean exposures. Descriptive statistics (table 1) confirmed that these observed workers did not differ to any notable extent from the total population of workers in the parent data set in terms of gender, age, working hours a week and percentage of workers with LBP at baseline. Thus, we consider selection bias among the observed workers to be unlikely.
In our study, video recordings were collected during four randomly chosen periods in a single work day. This procedure generates an estimate of each worker’s exposure, which is, by nature, uncertain. Distributing the four measurement occasions over several days would probably have resulted in a more precise exposure estimate, since exposure varies substantially between days for an individual worker (35, 36). More certain estimates of the exposures of individual workers in any particular group would, in turn, lead to a more precise mean exposure estimate even for the whole group (37). Thus, distributing exposure data collection over multiple days per worker could have had a slight influence on the reported effects of including an outlying exposure group. Also, the exposure variability (non-differential misclassification) between workers differed among the 19 groups (table 1), as well as the number of workers included in the group exposure estimate. Thus, the mean exposures in the 19 groups were determined with differing levels of precision. Regression coefficients will be biased in a group-based approach if group mean exposures are imprecise, as shown in theoretical studies (18, 19), but the size of this bias is difficult to assess on theoretical grounds in realistic study designs as the present one, where groups differ considerably in both size and exposure variability. Empirical simulation studies are an attractive alternative to analytical statistics in such cases.
The present study addressed only lifting and trunk flexion as risk factors for LBP even though several other physical and psychosocial exposures might be relevant, including metrics addressing the intensity and/or duration of lifting (1, 38, 39). Extrapolating our results to other exposure variables with different statistical structures is therefore not trivial. When studying LBP risk factors, multivariate analysis is often applied, taking into account confounders such as psychosocial factors and personal attributes. Properties of multivariate analysis were, however, not the focus of the present study. We chose lifting and trunk flexion as our main exposure variables since they have been shown to be important predictors of LBP in earlier investigations on the same cohort (10). Moreover, these two exposure variables were only weakly correlated in our parent data set (Pearson’s correlation coefficient: 0.34). Thus, while we cannot explicitly extend our results to other exposures, we do claim that the consistency of our results across two weakly correlated exposures suggests that the generic effects of our analysis may hold a fair external validity. We are further supported in this conviction by noting that our results were consistent across these two exposures even though they differ substantially in both absolute and relative sizes of within- and between-group variability (table 1). It thus appears warranted to assess the effect of these exposures on LBP independently of each other. Also, it seems reasonable to assume that our findings concerning the effects of the characteristics of an outlying exposure group on an exposure–outcome relationship have a fair external validity with respect to other variables describing trunk exposure.
Concluding remarks
The present study showed that the inclusion of a group of workers with an outlying, high exposure to lifting and trunk flexion, and a high LBP prevalence, profoundly influenced the shape and statistical properties of the association between exposure and LBP, even though the outlying group comprised <2% of the total study population. Using simulations, we found that the outlying group influenced the point estimate and lower boundary of the OR more if it deviated more in exposure from the other occupational groups in the population. An increase in the size of the outlying group and its LBP prevalence amplified these effects even more. Also study power was strongly influenced by the presence and properties of the outlying group. Our results may contribute to explaining inconsistencies in previous research, and they emphasize the decisive importance of including groups with “extreme” exposures in epidemiologic studies of associations between biomechanical exposures and musculoskeletal disorders. Moreover, our results emphasize that epidemiological studies should report results to the extent that possible effects of outlying groups, if present, can be appreciated, including sensitivity analyses of how exposure–outcome associations identified by the study might have been influenced by such groups.