Returning to work after a work-related injury is a dynamic multi-phase process that includes a sequence of states and transitions of different duration (1). Measuring return to work (RTW) at a single point in time may ignore the complexity of the RTW process (2, 3). Several studies have conceptualized RTW as a complex, multi-state outcome. For instance, Fan et al focused on differences in RTW types defined as partial, full, unspecified or non-RTW by the end of one year (4); Biering et al measured the fraction back at work using weekly data within one year following RTW (5); and Bültmann et al identified RTW trajectories based on RTW status and experienced recurrences at one and six months post-injury (6).
Traditional approaches to investigate predictors of RTW after work injury have typically relied on Cox proportional hazard models or other time-to-event analyses. While Cox proportional hazard models may be appealing in their simplicity, their assumptions are often violated which necessitates using other approaches. Moreover, the time-to-event analyses often used in these studies do not cover the transitions between RTW states, which are common among workers with musculoskeletal disorders (MSD). Pedersen et al analyzed transitions between four laborforce participation states defined as work, sickness absence (SA), unemployment and disability pension using sequence analyses (7). In 2016, Pedersen et al further evaluated the difference in RTW trajectories between sick leave due to mental health reasons and due to other reasons (8). In 2017, Lindholdt et al used sequence analysis to assess labor market participation for patients sick-listed with low-back pain in Denmark (9). As of writing, however, the value of sequence analysis to identify RTW trajectories added to traditional regression analyses for workers with a work-related MSD has not been explored.
To date, little is known about the RTW trajectory among injured workers with work-related MSD, such as sprains and strains, fractures, dorsopathies, and dislocations, which are associated with reduced quality of life and other social and economic costs (10). Worldwide MSD are a major cause of disability and, in high-income countries, a major cause of SA and work disability (11, 12). In Canada, MSD are the most prevalent chronic disorder and account for the highest costs for productivity losses due to disability (13). In British Columbia (BC), more than 65% of all lost-time claims in 2013 and almost 80% of all SA days between 2009 and 2013 were due to work-related MSD (14). The impact of work-related MSD on injured workers, employers and workers’ compensation systems is significant, and a better understanding of the RTW process is needed to develop effective interventions that reduce the duration of work disability.
We know that MSD episodes fluctuate over time and are characterized by recurrences or exacerbations (15, 16). Consequently, RTW after SA due to MSD is frequently marked by multiple spells of absenteeism and cannot be viewed as one RTW event (17). The purpose of this study was to (i) examine RTW after a work-related MSD using traditional time-to-event analysis, (ii) identify RTW trajectories among injured workers with work-related MSD, (iii) examine the associations between different MSD and RTW trajectories, and (iv) compare the results obtained from the various methods. The study used novel administrative health data from BC, Canada, that contained validated day-to-day RTW status measurements on all injured workers with a work-related MSD and at least one day of SA.
Methods
Jurisdictional context
In BC, Canada, workers who experience a recognized work-related injury or disease are provided with disability benefits, medical aid and rehabilitation services by WorkSafeBC, the provincial workers’ compensation board. WorkSafeBC, funded through employer-paid insurance premiums, provides short-term disability wage replacement to injured workers with the goal of getting workers back to work in a timely manner. Injured workers may remain on SA as long as they are recovering. Workers with permanent work-related impairments are provided with additional vocational rehabilitation and long-term disability benefits. Short-term disability payments, representing 90% of workers’ pre-injury wage, are provided until the worker returns to work in full capacity for most workers. Workers who reach a medical recovery plateau are eligible for vocational re-training and/or long-term disability and would be considered non-RTW (NRTW). Workers deemed fit to return to work but who have no job to return to are also considered NRTW. A small proportion of injured workers are deemed NRTW as they did not comply with medical or rehabilitation treatment and lost eligibility for benefits.
This study focuses on injured workers during the period in which they are provided short-term disability benefits, which for consistency with other literature and the European context we refer to as work-related SA. About 94% of the BC laborforce is covered by this work disability insurance arrangement.
Study design and study sample
Accepted work-related MSD lost-time claims filed between 1 January 2010 and 31 December 2012 were identified from administrative health data collected and kept by WorkSafeBC. The claim database contains information on injury and claim registration dates, as well as demographic, wage, occupation and employer variables. The claims data was linked to RTW calendar data that records detailed RTW status at a daily level. The follow-up period was restricted to a maximum of one year, measured as 52 weeks from the first recorded time-loss day. The Behavioral Research Ethics Board at the University of British Columbia approved the study (Certificate no. H15-00779).
Study eligibility required claims to be accepted for disability compensation related to a MSD and to involve at least one full day off work. The cohort was restricted to the first MSD work disability claim per worker in the study cohort leading to one RTW trajectory per worker in the study. We excluded claimants without a claim related to a classifiable MSD [defined using International Classification of Diseases, Ninth Revision, Clinical Modification (ICD)-9-CM codes and National Work Injury Statistics Program (NWISP) WorkSafeBC Nature of Injury codes], age <15 or >65 years, less than one day off work, multiple jobholders, incomplete claims, claim related to previous non-MSD claims (ie, consolidated claims), claims from self-insured industry sectors, or claims related to fatal injuries. Claims with missing data on firm size, industry, wage or gender information were also excluded.
A total of 108 398 unique claims were identified between 1 January 2010 and 31 December 2012. The eligibility restrictions were conducted in four stages: (i) restrict the cohort to the first claim per worker in the years 2010–2012 (excluding 12 442 claims); (ii) exclude claims based on the previously stated exclusion criteria (N=14 068); (iii) exclude claims based on missing data (N=462); and (iv) restrict the cohort to workers with at least one day off work to ensure a RTW trajectory (excluding N=384) (see Figure 1 for more detailed information). This led to a final cohort of 81 062 workers.
Outcomes
The outcome variable in this study was employment status during the 52 weeks following the first time-loss day. In the time-to-event analyses, the outcome was sustained RTW, which was defined as the period (in calendar days) between the first time-loss day and has RTW as end event of the claim. In the sequence analysis, the outcome was extended to include four different RTW states, of which the RTW trajectories were developed.
Definition of RTW states
Each claim had a potential of four RTW states after injury that were measured during the one year of follow-up. These were SA, modified RTW (MRTW), RTW, and NRTW. SA signifies that the injured worker was not working in any capacity due to temporary disability and that the worker was still receiving wage loss benefits at that time. MRTW means a worker was back at work, but was either working fewer hours than pre-injury or had been assigned alternative or modified duties, or both. RTW means that a worker was back at work and conducting full pre-disorder work duties. NRTW indicates that a worker was not working, but was deemed fit enough for full duties or that a worker was unable to return to work due to a recovery plateau, permanent functional impairment or vocational rehabilitation referral. In this case, the worker was no longer entitled to receive short-term wage-loss benefits.
Definition of RTW trajectory
RTW trajectories consisted of a sequence of RTW states that were derived from the RTW calendar data. The calendar data captured the start and end date for each state. Each trajectory started with an injury and was characterized by a sequence of RTW states. For this study, all trajectories began at the date of the first RTW state following the injury date. We converted the daily RTW calendar data into time periods of four weeks starting from the start date of the first RTW state. This created a uniform sequence of RTW states spanning the one-year follow-up period where each time period was assigned the RTW state noted at the end of that period. The RTW trajectories, then for each worker, comprised a maximum of 13 states. For instance, workers who had returned to work by the end of the 4th period and experienced MRTW for the three previous periods would have the following trajectory: MRTW-MRTW-MRTW-RTW. For trajectories ending in RTW or SA the end state was considered to be sustained throughout the follow-up period as no other transition was observed. For trajectories ending in NRTW, the end state was censored until the end of the follow-up period as there was no subsequent record of the injured worker.
Musculoskeletal disorders (MSD)
MSD were categorized into nine major categories using the Barell matrix (18) (for musculoskeletal injuries; sprains/strains, fractures, dislocations) and ICD-9-CM codes (for musculoskeletal diseases; dorsopathies and rheumatism, excluding the back). Sprains/strains and fractures were divided into three body regions; (i) head/neck/spine/back/torso; (ii) upper extremities; and (iii) lower extremities. Disorders not mapping to one of these nine categories were excluded from the cohort, because they were too small to be an independent category (N=2005).
Covariates
All analyses were adjusted for the following variables, obtained from the claim database: age, gender, wage, history of prior claims, occupation, industry sector and size of the workers’ firm. All continuous variables were categorized; age at the time of the disorder (15–24, 25–34, 35–44, 45–54, 55–64 years); annual wage at the time of the disorder [<$20 000, $20 000–39 999, -$40 000–59 999, >$59 999 (Canadian dollars, CAD)]; and firm size, measured as fulltime-equivalent (FTE) workers employed by the firm (<20, 20–99, 100–499, 500–999, >999 FTE). Prior claims were defined as at least one accepted claim in the preceding ten years to the MSD claim. Occupation was classified by ten categories according to Statistics Canada’s Standard Occupational Classification (19). Industry sector was classified by seven categories according to the WorkSafeBC industry classification structure (20).
Statistical analysis
Descriptive statistics (frequency counts and proportions) were applied to describe the total study cohort and compare the baseline characteristics between trajectory clusters.
Cox regression was used to examine the differences in time to sustained RTW between the MSD by calculating cumulative incidence proportions (CIP) and hazard ratios (HR) at the end of the year after the first time-loss day. The analyses were conducted unadjusted and adjusted for the following covariates: age, gender, wage, firm size, history of prior claims, occupation, and industry sector.
The RTW trajectories were constructed using the Stata program for sequence analysis, SQ-Ado (21). The program identified and enumerated each trajectory. For analysis purposes the trajectories were clustered. Initially optimal matching (OM) and cluster analysis was considered using methodology established by McVicar and Anyadike-Danes to cluster time-series sequences of monthly labor market activities (22). However OM approaches did not lead to coherent clusters as the terminal RTW end state was underweighted using this methodology. As an alternative, we derived the following decision rules and then applied these rules programmatically to group the trajectories. Decision rules were determined taking three characteristics of the trajectories into account; (i) the end state of a trajectory; (ii) the time to the end state; and (iii) the type of states preceding the end state. Nine clusters were identified. In the statistical analysis, we examined the association of the six most common trajectory clusters with early sustained RTW as the reference cluster.
Poisson regression with robust standard errors was used to generate relative risk ratios (RR) with 95% confidence intervals (CI) between MSD type and a referent trajectory and the other trajectories. This is consistent with the methodology outlined in Zou et al (23) that enables the comparison of relative risks across a number of discrete outcome categories. All analyses were conducted unadjusted and adjusted for the following covariates: age, gender, wage, firm size, history of prior claims, occupation, and industry sector. All analyses were performed in Stata 13 (Stata Corp LP, College Station, TX, USA).
The Cox regression HR and Poisson regression RR may be qualitatively compared given the construction of the respective statistical model, higher HR imply faster RTW, while higher RR imply a greater likelihood of being in a given RTW trajectory.
Results
Sample characteristics
A total of 81 062 workers was included in the final cohort. The sample comprised of 50 829 (63%) men and 30 233 (37%) women; the mean age of the workers was 41 years and their mean annual wage prior to injury was approximately CAD$40 000. Sixty-five percent of the workers had a history of prior claims. More than a third of all occupations were in trades. The most common industry sector was service (42%) and the median firm size was 144 FTE workers. Sprains and strains (N=63 729) were the most common disorder type, fractures (N=7974) and musculoskeletal diseases (N=7966) such as rheumatism and dorsopathies were the second and third most common in the cohort, while dislocations (N=1393) were the least common. Descriptive statistics of the sample characteristics in total, and by cluster, are shown in the appendix (www.sjweh.fi/show_abstract.php?abstract_id=3701).
Time until RTW
A total of 88% of the workers with back sprains and strains reported having returned to work one year after the first time-loss day (table 1). Workers with all other injuries (except lower-extremity sprains and strains) reported lower HR to RTW within one year. The lowest HR to RTW was for workers with torso fractures (HR 0.49, 95% CI 0.46–0.52), only 70% of these workers returned to work after one year (table 1).
Table 1
Likelihood of return to work among individuals on sickness leave due to a musculoskeletal disorder during 1-year follow-up using Cox survival analysis. [CIP=cumulative incidence proportion, percentages of individuals having returned to work within one year after injury; HR=hazard ratio; CI=confidence interval.]
RTW trajectories and clusters
A total of 2132 unique trajectories, using four states (SA, MRTW, RTW, NRTW), were identified in the final sample with the 20 most common trajectories covering 86% of the claims (see Figure 2). Nine different clusters were identified based on the sequence analysis and decision rules, of which the six most common were used for analyses (see table 2). For examples of specific trajectories that were classified in each cluster, see Figure 3.
Table 2
Description of return to work (RTW) trajectory clusters. [MRTW=modified RTW; SA=sickness absence; NRTW=non-RTW]
Figure 3
Example of return-to-work (RTW) trajectories by cluster. [T=timeframe (denoting a 4-week SA spell); EE=end event.]
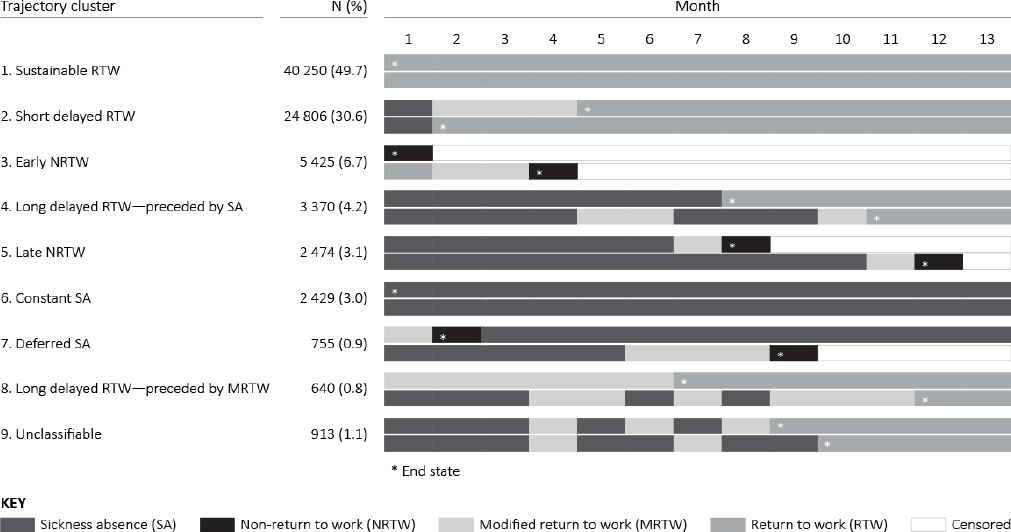
Half of the workers (49.7%) returned to work by the first month (early sustained RTW) and 30.6% of the sample had a short delay before RTW (short-delayed RTW). The other clusters were early NRTW (6.7%), long-delayed RTW preceded by SA (4.2%), late NRTW (3.1%), constant SA (3.0%), deferred SA (0.9%), long delayed RTW preceded by MRTW (0.8%), and a cluster with unclassifiable trajectories (1.1%).
Associations between MSD and RTW trajectories
The RR from the Poisson regression can be interpreted as the increase or decrease in the risk of being in a trajectory cluster relative to early sustained RTW. Table 3 shows the adjusted results of the Poisson regressions models. Adjusting for age, wage, gender, previous claim, industry, occupation, and firm size only slightly attenuated the RR of NRTW, long delayed RT, or constant SA compared to early RTW.
Table 3
Adjusted relative risk ratios (RR) for musculoskeletal disorders (MSD) with return-to-work (RTW) trajectory clusters, Poisson regression with robust error variance [CI=confidence interval; NRTW=non-RTW; SA=sickness absence].
Musculoskeletal disorder | Short-delayed RTW a(cluster 2) | Early NRTW (cluster 3) a | Long-delayed RTW, preceded by SA (cluster 4) a | Late NRTW (cluster 5) a | Constant SA (cluster 6) a | |||||
---|---|---|---|---|---|---|---|---|---|---|
|
|
|
|
|
||||||
RR | 95% CI | RR | 95% CI | RR | 95% CI | RR | 95% CI | RR | 95% CI | |
Back sprains and strains | 1.0 | Ref | 1.0 | Ref | 1.0 | Ref | 1.0 | Ref | 1.0 | Ref |
Upper extremity sprains and strains | 1.1 | 1.0–1.1 | 1.0 | 0.9–1.1 | 2.5 | 2.3–2.8 | 1.3 | 1.2–1.5 | 3.4 | 3.1–3.8 |
Lower extremity sprains and strains | 0.9 | 0.8–0.9 | 0.8 | 0.8–0.9 | 2.1 | 1.9–2.4 | 1.0 | 0.9–1.1 | 1.8 | 1.5–1.9 |
Upper extremity fractures | 1.9 | 1.9–2.0 | 1.7 | 1.5–1.9 | 5.7 | 5.1–6.5 | 4.0 | 3.6–4.9 | 4.9 | 4.1–5.7 |
Lower extremity fractures | 2.1 | 2.0–2.2 | 2.0 | 1.8–2.3 | 9.9 | 8.9–11.1 | 7.6 | 6.7–8.8 | 9.0 | 7.8–10.4 |
Torso fractures | 1.7 | 1.6–1.8 | 1.9 | 1.6–2.2 | 4.9 | 4.1–5.9 | 5.9 | 4.9–6.9 | 7.7 | 6.6–8.9 |
Dislocation | 1.6 | 1.5–1.7 | 1.4 | 1.2–1.7 | 6.2 | 5.3–72 | 2.8 | 2.2–3.7 | 7.6 | 5.2–7.8 |
Dorsopathies | 1.0 | 0.9–1.0 | 1.0 | 0.9–1.2 | 2.3 | 2.0–2.7 | 1.9 | 1.6–2.1 | 3.0 | 2.6–3.6 |
Rheumatism (excluding the back) | 1.3 | 1.2–1.3 | 1.1 | 1.0–1.3 | 2.6 | 2.2–3.0 | 2.2 | 1.9–2.6 | 2.7 | 2.3–3.3 |
Workers suffering from back (head, neck, spine and torso) strains and sprains were more likely to be in early sustained RTW by end of the first month than to have delayed RTW, be absent or NRTW, compared to other disorder types. Exceptions included: (i) workers with sprains and strains of the lower extremities who were less likely to have a short delayed RTW (adjusted RR 0.9, 95% CI 0.8–0.9) or an early NRTW (adjusted RR 0.8, 95% CI 0.8–0.9) trajectory; (ii) workers with upper extremity strains in short delayed RTW cluster (adjusted RR 1.1, 95% CI 1.0–1.1) and early NRTW- (adjusted RR 1.0, 95% CI 0.9–1.1); and (iii) workers with dorsopathies in short delayed RTW cluster (adjusted RR 1.0, 95% CI 0.9–1.0) and early NRTW cluster (adjusted RR 1.1, 95% CI 0.9–1.2).
The risk for being constantly absent post injury or experiencing a long delay of RTW and being predominantly absent before returning to work was – compared to back strains – considerably increased for all disorders. Workers injured by fractures or dislocation had a consistently high risk for these two clusters (adjusted RR 4.9–9.9). For both the NRTW clusters and the short delayed RTW cluster, the fractures and dislocation groups were also most at risk, although these risks were typically lower than for long delayed RTW preceded by SA and constant SA.
Discussion
Main findings
This study examined RTW outcomes using RTW trajectories that were derived from day-to-day calendar measurements of RTW states. First, Cox regression analyses showed that workers with back sprains and strains were most likely to return to work within one year after MSD, compared to all other MSD. Second, using sequence analysis, we visualized the complex trajectory of RTW after a work-related MSD. We identified 9 trajectory clusters: early sustained RTW, short-delayed RTW, early NRTW, long-delayed RTW preceded by SA, late NRTW, constant SA, deferred SA, long-delayed RTW preceded by MRTW, and unclassifiable. Compared to most other disorder types, workers suffering from back (head, neck, spine and torso) strains and sprains were more likely to have early sustained RTW by the end of the first month after injury than to have delayed RTW or be absent or NRTW.
The application of sequence analysis and the identification of RTW trajectories yielded several key insights not found using conventional Cox regression analysis. While Cox proportional hazard models may be appealing in their simplicity, their assumptions are often violated (as in this study), which necessitates using other models as complex as sequence analysis. The identification of RTW sequences showed that RTW could not be conceptualized as a single transition (ie, a period of sick leave until RTW), but that that it was it was a series of states and transitions with three distinct outcomes at the end of the study period (NRTW, RTW or SA). Our clustering of the sequences prioritized the end event and duration of the trajectories and enabled us to examine RTW outcomes ignored by the conventional application of Cox regression. Our results show that the MSD most associated with constant SA or early and late NRTW differed than those most associated with early or late RTW. For example, dislocations were only modestly associated with NRTW but had one of the largest associations with constant SA while upper-extremity fractures had a similar risk of long-delayed RTW as other fractures, but a lower risk of constant SA.
Associations between type of MSD and RTW outcomes diverge from some findings of previous research. Cheadle et al suggested that back/neck strains predicts a longer duration of disability (24), while Hubertsson et al presented a shorter sick leave for low-back pain (25). Our results suggest that the back strain group returned to sustainable work sooner than all other disorder groups. Possibly, this was caused by the duration of low-back pain in this group. Acute low-back pain is suggested to have a rapid improvement (26, 27) and this diagnosis may have been, due to its high prevalence, predominant in the back strain group. In contrast, workers with fractures or dislocations were more likely to experience a more complex RTW trajectory. This may have been a consequence of longer recovery processes these disorders seem to experience and therefore require a longer time off work. These disorders may be more serious in that they were more likely to end in a permanent functional impairment or a recovery plateau. In a study on severe fractures of lower extremities, MacKenzie et al presented a strong relationship between severe impairment and the timing of RTW (28).
Advances in measuring RTW using sequence analysis
Our finding indicate that sequence analysis provides additional analytical value and insight in supplement to standard regression analyses (like Poisson regression, as applied here) to investigate associations between specific groups and their RTW trajectories. Three other studies (assessing labor market outcomes among patients with low-back pain or neck and shoulder pain) have used sequence analysis in combination with traditional regression analyses. These studies also show the value of sequence analysis in assessing RTW outcomes (9, 29, 30). Prior to the recent publication of these studies, multistate models were commonly used to investigate multiple states of the RTW process (31). However, multistate models analyze the transition between RTW states, where sequence analyses provides the opportunity to create RTW trajectories optimizing the use of day-to-day calendar data. It describes the process over time, instead of analyzing time to events or effect at a given point in time. Our results could be used as an argument for investigating RTW trajectories following injury on short- and long-term RTW and NRTW outcomes.
Challenges in measuring RTW using sequence analysis
We recommend using sequence analysis as an adjunct analysis in combination with other regression techniques as it is not possible to perform statistical testing or adjust for covariates and confounders when only using this technique (9, 32).
Secondly, using day-to-day calendar data with a population-based cohort results in very detailed RTW trajectories, and we were required to simplify the RTW trajectory data to create comparable timeframes across individual trajectories. This led to ignoring RTW transitions that took place within each four-week period. This simplification was needed to reduce the dimensionality of the number of potential trajectories and to apply a sequence analysis technique that required evenly spaced time periods. Notwithstanding, this is the first study to examine up to 13 distinct RTW states and transitions over a one-year period using the combination of sequence analysis and multivariate regression.
Thirdly, the trajectories were clustered based on decision rules that emphasized three characteristics of the trajectories and that privileged the end RTW state. Other decision rules may have led to a different set of clusters, and the impact of these decisions are important to investigate in future research.
Strengths and limitations of the study
The detailed population-based data used in this study representing all time-loss claims for MSD in the province of BC, Canada, coupled with the detailed and refined RTW outcome is a main strength of this study. Further, the detailed diagnostic coding of MSD enabled the examination of how different MSD affect RTW cluster membership. The administrative data also contained a comprehensive set of confounders permitting the estimation of fully adjusted models.
A limitation of using administrative data is that it may be subject to misclassification or miscoding and lead to information bias. However, cleaning of the data was intensive and incomplete claims were excluded from analysis. Moreover, the results may not be generalizable to jurisdictions that have different assessment, treatment and benefit criteria. Finally, there may have been unmeasured psychosocial, clinical and economic confounders that leading to some residual confounding across injury types (33).
Implications for research and practice
This study has added to the knowledge base applying sequence analysis to defining RTW trajectories and has identified specific MSD that may be related to longer or more complex RTW trajectories.
The RTW calendar data coupled with the population-based administrative data create the potential to explore other research questions related to understanding the RTW trajectory. This study showed the potential of using sequence analysis in addition to traditional regression analyses. Sequence analysis is an exploratory method that allows researchers to investigate RTW as a multi-state outcome and visualize RTW trajectories. However, future research is necessary to improve the clustering and the use of more detailed timeframes is needed in order identify other types of RTW trajectories.
Policy-makers and occupational health professionals can use this knowledge to identify groups of injured workers who may be at risk of poorer RTW outcomes and in prioritizing interventions for these groups.
Concluding remarks
This study identified RTW trajectories for workers with a work-related MSD using sequence analysis and found that workers with back strains were more likely to have an early sustained RTW trajectory, while workers with fractures or dislocations were more likely to have SA and NRTW trajectories. These differences suggest that it is important to differentiate between specific diagnoses when investigating RTW trajectories in research and practice. This study provides a more in-depth look at RTW as a process by using population-based data and RTW trajectories. We also showed the potential of using sequence analysis in combination with regression analyses to investigate RTW after MSD as a trajectory.