The role of personal characteristics in an employee’s experience of job strain has been the focus of growing research interest in recent years (eg, 1, 2, 3). Two crucial questions are, firstly, which personal characteristics function as risk factors for experiences that are known to affect employee well-being and, secondly, what is the relation between these personal characteristics and characteristics of the job across time. Although some associations have been found between personal characteristics and job characteristics (eg, 3, 4, 5), their predictive associations are still unclear due to the limited longitudinal research with a full panel design. Knowledge on these predictive associations is needed in order to fully understand how job strain develops from the viewpoint of the individual.
The aim of the present 8-year, 5-wave follow-up study among Finnish employees was to explore the predictive associations between employees’ personality characteristics and their experiences of their job. The study was based on the widely used effort–reward imbalance (ERI) model, developed originally by Siegrist (6). We chose the ERI model for our study because it provides a rich theoretical framework for understanding both the job-related reasons behind employees’ experiences of strain as well as the role of employees’ personality characteristics [ie, overcommitment (OC)] as a risk factor in these experiences. Thus, the present study increases understanding of the ERI model, the reciprocal nature of its key components, and the underlying predictive mechanisms between situational and personal features (ie, OC) in job strain in the long run.
The effort–reward imbalance model
The ERI model has its roots in the notion of social reciprocity, which explains how the costs and gains of social exchanges determine people’s behavior with others (6). Costs and gains at work are depicted through the job-related characteristics of effort and reward. Effort represents an employee’s perception of the strain caused by the various demands and responsibilities of the job imposed by the employer, such as interruptions, overtime, and obligations. Effort at work is ideally reciprocated by socially defined rewards which are not restricted only to wages but also include performance-related esteem, career opportunities, and job security.
When an employee perceives an imbalance between effort and reward at work, (s)he is assumed to have a greater risk of poorer health (6, 7). Such an imbalance may be experienced, for example, in a situation where an employee must repeatedly work overtime to complete his or her overloaded projects, but does not receive appropriate remuneration for this. The failed reciprocity in terms of high effort and low reward elicits strong negative emotions and this, in combination with sustained autonomic activation results in adverse long-term consequences for physical and mental health (8). High ERI has been found to associate with increasing risks of cardiovascular diseases (for a review, see 7, 9), mortality (eg, 10), poor general mental health (11), and higher psychological distress (12). High ERI has also been found to associate with work-related outcomes such as emotional exhaustion at work (13, 14) and turnover intentions (15).
While ERI reflects extrinsic demands, employees’ personal motivation is taken into account in the ERI model (6) by the concept of OC. With its assessment of an employee’s OC, the ERI model takes into account a personality feature that reflects the cognitive, emotional and motivational characteristics of an employee in relation to his or her work. An overcommitted employee is very ambitious, feels the need to be approved and esteemed by others, and often has difficulty withdrawing from work during leisure time (7). Like effort and reward, also pronounced OC – due to the nonsymmetric exchange – is assumed to be associated with an employee’s reduced health and well-being (6, 7). Highly overcommited employees underestimate challenging situations and overestimate their own capacity, and consequently they tend to invest (too) much effort in their job. In addition, although their excessive efforts are often not met with adequate rewards, overcommitted employees tend to maintain their level of involvement (16). A high OC has been found to be associated with insomnia (17), poor self-rated health (18), and emotional exhaustion at work (19). The risk for poor health is expected to be highest when high OC is combined with high ERI (6, 7).
The present study
The aim of the present study was to examine the cross-lagged effects between the key concepts of the ERI model across eight years. Our focus was on the role of person-related OC as a possible predictive factor of ERI experiences. In general, the concept of OC has received much less research attention compared to the other indicators of the ERI model (10, 16). We consider it is important to investigate the possible predictive role of OC on ERI indicators because it brings new light on the dynamic role of the person- and job-related components of the ERI model. This kind of causality analysis provides more information about the role of OC in an employee’s job strain experiences. If high OC increases ERI experiences in the long run, it would mean that OC is not only a risk factor for lowered health (as it is shown to be so far) but also a risk factor for increasing experiences of imbalance between effort and reward at work.
In our longitudinal study there were altogether five measurement points with two-year time lags. We considered two years an appropriate time lag to show the lagged relationships between the relatively stable personality feature of OC and the less stable job-related characteristics of effort and reward (see 20). It is suggested that a time period of at least two years is needed for lagged effects of chronic workplace stressors to emerge (21, 22). Our study met the criterion of a full panel design as both the contextual, job-related characteristics of effort, reward, and ERI and the personality feature of OC were measured at each measurement time. Thus, the stabilities of the constructs as well as the predictive associations between job- and person-related features could be tested in a valid manner.
We hypothesized that employees’ high OC predicts their later perceptions of high effort (H1a), low reward (H1b), and high ERI (H1c) (normal causation model). We based these assumptions on the role of OC as a personal characteristic in the ERI model. On the one hand, as a personal characteristic, OC is expected to remain more stable than the contextually dependent effort, reward, and ERI. Thus, it is reasonable to assume that the person-related OC predicts more modifiable ERI indicators in the long run. It is reasonable to expect the higher stability of OC as it has been found to be associated with high neuroticism (23, 24), a personality trait which shows relatively high stability in adulthood (25, 26). On the other hand, overcommitted employees are driven by their strong need for control and approval (7), and therefore they are constantly willing to put extra effort into their work. At the same time, they may expect to be rewarded from their invested effort in line with the basic idea of reciprocity in social exchange theories, in which ERI model has its roots (6). However, they may feel dissatisfied with the rewards they receive because of their pronounced commitment to their work. A nonsymmetric exchange occurs. Taking into account both of the above expectations (high OC predicts both high effort and low reward), OC is also expected to predict a high mismatch between efforts and rewards (ie, ERI).
To confirm the validity of H1, we also tested reversed associations between OC and effort, reward, and ERI (reversed causation model). In other words, high effort at work (eg, time demands, responsibilities, overtime) might lead to high OC (H2a), which would be seen, for example, in an employee’s difficulties in detaching from work during nonwork time, which is the main component of OC (7). This expected association is in line with the stressor-detachment model (27), which shows that demands at work predict difficulties in psychological detachment from work (see 27, for a review). Furthermore, low rewards (eg, low esteem and high insecurity) might evoke commitment to work (H2b), because in such situation an employee might try to show that he or she is a good worker. In accordance with this kind of reasoning it has been shown, for example, that job insecurity can be a motivator among employees to work harder (eg, 28). As a result of both of these assumptions, a mismatch between high effort and low reward (ie, high ERI) might lead to an increase in an employee’s OC (H2c), that is, one tries to put additional effort into one’s work in order to cope with the demands and gain better and more satisfying rewards.
Finally, the condition where both of the above assumptions (H1a–c and H2a–c) are simultaneously present was also tested (reciprocal model). In the reciprocal model, OC associates with increasing perceptions of effort, decreasing perceptions of rewards, and increasing ERI and, conversely, high effort, low rewards, and high ERI associate with increasing OC (H3).
Method
Participants and procedure
The present study is a part of a larger research project focusing on working conditions and well-being of young professionals working in technical fields (for more details, see 29). The longitudinal study was conducted with five measurement points, in 2006 (T1), 2008 (T2), 2010 (T3), 2012 (T4), and 2014 (T5).
The baseline sample (T1) was selected from the membership registers of two national trade unions in Finland (Pro and Union of Professional Engineers). The baseline sample consisted of 1904 members <36 years of age whose professional title indicated a management or leadership position. Questionnaires were sent to their home addresses and 933 questionnaires were returned. Of the respondents, 186 were not in management or in employment (eg, they were on maternity leave, studying, or had been unemployed for >3 months) and these respondents were therefore excluded from the final sample (N=747). The response rate was 43% in 2006. Of the respondents, 85% (N=637) were men. Their average age was 31 years (SD 3.2, range 24–36). The majority of participants were engineers (68%) and other participants were technicians (6%) or had other technical qualifications (26%). Eight percent worked in upper management (managing director or management), 49% in middle (eg, head of department, project director), and 43% in lower management (supervisor).
At T2, questionnaires were sent to 621 of the participants who had participated at T1. At T1, 126 participants had indicated that they did not wish to participate in the research in the future and were therefore excluded from the follow-up study at T2. At T2, 422 employed participants completed the ERI scale.
At T3, questionnaires were sent to 595 participants, since at T2 a further 26 participants had declined to take part further. At T3, 368 employed participants completed the ERI scale.
At T4, the questionnaire was sent to 575 participants, since a further 20 participants had indicated at T3 that they no longer wished to be contacted. At T4, 325 employed participants completed the ERI scale.
At T5, the questionnaire was sent to 562 participants, which took into account the further 13 participants who had indicated at T3 that they no longer wished to be contacted. At T5, 273 employed participants completed the ERI scale.
Measures
The key variables of the ERI model were measured by the 23-item ERI scale (6) at each time point. The ERI scale is a psychometrically well-justified measure (7, 11) and its theoretically based factor structure has found to be valid in different countries including Finland (15, 20, 30). Effort is assessed in the ERI scale with five items (eg, “I have constant time pressure due to a heavy work load”). The participants gave their answers on a response scale from 1 (“does not apply to my job”) to 5 (“does apply to my job and I am very distressed”). The mean scores were calculated for each measurement point: the higher the score, the more stressful was the effort reported.
Reward included 11 items describing esteem (5 items, eg, “I receive the respect I deserve from my superiors”), career opportunities (4 items, eg, “Considering all my efforts and achievements, my salary/income is adequate”), and job security (2 items, eg, “My job security is poor”). The response scale for reward items was the same as for the items describing effort but before data analyses were carried out the scoring was reversed at each measurement point so that the higher the mean score, the greater the esteem, career opportunities and job security each reflected.
ERI was measured by the ERI ratio at each measurement point. Standard procedures for computing the ERI were followed (see 7, 31). A score close to 0 indicates a favorable situation, in which the rewards received outweigh the effort invested at work; a score >1 indicates an unfavorable situation, where effort outweighs expected or received rewards. In the present study, the ERI ratio was used as a continuous variable at each measurement point.
OC was measured with six items (eg, “As soon as I get up in the morning I start thinking about work problems”) with a response scale from 1, “strongly disagree”, to 4, “strongly agree”. The sum scores for OC were calculated for each measurement time: the higher the score, the more OC was reported.
Table 1 shows the ranges, means, SD, and Cronbach’s α for the variables of the ERI model at each measurement time. The mean scores of the study variables indicated that participants perceived their rewards relatively high, and effort and OC moderate in five measurement points. The intercorrelations between all the study variables are available from the corresponding author on request.
Table 1
The descriptive information of the study variables at T1, T2, T3, T4, and T5. [SD=standard deviation.]
Control variables
Weekly working hours and job changes between the study waves were taken into account in our analyses as control variables. Average working hours were the following: at T1 38.5 (SD 2.9), at T2 38.0 (SD 3.3), at T3 38.2 (SD 4.0), at T4 38.0 (SD 5.7), and at T5 39.3 (SD 3.9). Of the investigated participants at T2, 29% (N=121) had changed their job during the past two years (between T1 and T2). The corresponding percentages were 17% (N=64) at T3, 21% (N=69) at T4, and 16% (N=44) at T5.
Attrition analysis
The baseline attrition analysis showed that the participants at T1 (N=747) did not differ in gender from non-respondents (N=1157) (for more details, see 26). The data on the non-respondents’ age was only available for the members of Trade Union Pro; these respondents (N=331) did not differ from non-respondents (N=379) in age by the independent samples t-tests.
Next, the participants at T1 were compared in terms of background variables (gender, age) and the variables of the ERI model to those participants who had responded at T1 but not at T2. The chi2 tests indicated that there were no differences in gender distribution. Similarly, no differences were found in age, effort, reward or OC as indicated by the independent samples t-tests. Attrition analyses were also performed to compare those participants who responded at T1, but did not participate in later time points (at T2, T3, T4, or T5) to the ones who participated in later time points. These analyses also showed that there were no differences in gender, age, effort, reward and OC between respondents and non-respondents.
Data analysis
The present study examined the predictive associations between effort, reward, ERI, and OC using structural equation modelling (SEM). The SEM analyses were performed with the Mplus 7.2 programme (32) using the robust maximum likelihood (MLR) estimation method which is full information maximum likelihood method (FIML) and gives robust standard errors and scale corrected chi2 value. The MLR method is recommended in a case when the observed variables are distributed non-normally. FIML does not exclude participants with incomplete data in given variables but instead uses all available data in order to estimate the model. Thus, missing values are not excluded or imputed. Rather, parameter estimates and standard errors are estimated directly from the observed data (33, 34) and the significance of each estimate in the model is based on the observed number of cases. FIML is a preferable method in the analysis of the present multi-wave longitudinal data with missing values (list wise N=165). By using FIML, our SEM analyses are based on 747 (at T1), 422 (at T2), 368 (at T3), 325 (at T4), and 273 (at T5) participants when estimating cross-sectional intercorrelations of the study variables. When estimating longitudinal stabilities and predictive associations of the study variables, our SEM analyses are based on 422 (T1–T2), 297 (T2–T3), 257 (T3–T4) and 222 (T4–T5) participants.
We investigated cross-lagged relationships first between effort, reward, and OC, and then between the ERI ratio and OC. Separate SEM analyses were needed because effort and reward variables are included in the ERI ratio. Because of the high number of items in the ERI scale (115 items in all, at five time points) in relation to sample size, we were not able to estimate the SEM models by using latent factors based on the original observed items. Instead, we used the mean scores of effort, the ERI ratio and OC at each measurement point. Reward was investigated using a latent variable, where the mean scores of its three subscales, that is, esteem, career opportunities, and job security, were set to load on the latent reward factor at each measurement time. Using mean scores in SEM models is well justified in the case of scales with high structural validity. The structural validity of the ERI scale has received strong support in previous studies (7, 11, 20, 30). Most importantly, the factor structure of the ERI scale was found to remain stable in the current longitudinal study sample; ie, no significant changes in factor loadings of the scale occurred across the first time period (20). It is therefore very unlikely that the possible stabilities and cross-lagged effects of the ERI indicators and OC were a result of any instability in the structure of the ERI scale. Furthermore, the reliability of each of the sum scores was high, which further supports the use of the mean scores in the analyses (see table 2).
Table 2
Goodness-of-fit statistics for structural equation models (SEM) including effort, reward, and overcommitment (OC) at T1, T2, T3, T4, and T5 (job changes between the study waves are controlled for in all SEM models). [RMSEA=root mean square error of approximation; TLI=Tucker-Lewis Index; CFI=Comparative Fit Index; ns=non-significant]
Tested SEM models | χ2 | df | RMSEA | TLI | CFI | Model comparisons:Δ χ2 (df) |
---|---|---|---|---|---|---|
1a. Stability model, freely estimateda | 731.806 | 322 | 0.041 | 0.89 | 0.91 | |
1b. Stability model, constrainedb | 740.821 | 330 | 0.041 | 0.89 | 0.91 | 1b versus 1a: Δ χ2(8), 9.02, ns. |
1c. Stability model, constrainedc | 772.144 | 342 | 0.041 | 0.89 | 0.90 | 1c versus 1b: Δ χ2(12), 30.75, P<0.01 |
1d. Stability model, constrainedd | 789.144 | 338 | 0.042 | 0.88 | 0.90 | 1d versus 1b: Δ χ2(8), 44.34, P<0.001 |
2. Normal causation model | 708.529 | 322 | 0.040 | 0.90 | 0.91 | 2 versus 1b: Δ χ2(8), 32.29, P<0.001 |
OC at T1 → Reward and Effort at T2 | ||||||
OC at T2 → Reward and Effort at T3 | ||||||
OC at T3 → Reward and Effort at T4 | ||||||
OC at T4 → Reward and Effort at T5 | ||||||
3. Reversed causation model | 719.398 | 322 | 0.041 | 0.89 | 0.91 | 3 versus 1b: Δ χ2(8), 21.18, P<0.01 |
Reward and Effort at T1 → OC at T2 | ||||||
Reward and Effort at T2 → OC at T3 | ||||||
Reward and Effort at T3 → OC at T4 | ||||||
Reward and Effort at T4 → OC at T5 | ||||||
4. Reciprocal model, conditions of models 3 and 4 are simultaneously valid | 694.437 | 314 | 0.040 | 0.89 | 0.92 | 4 versus 1b: Δ χ2(16), 45.82, P<0.001 4 versus 2:Δ χ2 (8), 14.44, ns. |
a Factor loadings of the reward factor and cross-sectional intercorrelations and stability coefficients of effort, reward, and OC estimated freely across time.
b Factor loadings of the reward factor estimated equal across time and cross-sectional intercorrelations and stability coefficients of effort, reward, and OC estimated freely across time.
The cross-lagged SEM models were estimated in four stages. Firstly, we estimated a stability model (with control variables) in which each construct (effort, reward, OC) was set to predict itself across measurement times and in which the intercorrelations between the variables were estimated within each measurement point. The stability model served as our baseline model, to which our further cross-lagged models were compared. Secondly, the normal causality model was estimated, where OC at T1, T2, T3, and T4 was set to predict the effort and reward (and ERI in a separate SEM model) that was measured two years later. Thirdly, the reversed causality model was estimated, where effort and reward (and ERI in a separate SEM) at T1, T2, T3, and T4 were set to predict OC two years later. Finally, a reciprocal model was estimated in which the conditions of the normal causality model and the reversed model were simultaneously valid. The goodness of fit of the cross-lagged models were compared to the stability model using the Satorra-Bentler scaled chi2 difference test (35). If the chi2 difference test showed a significant improvement for the cross-lagged model as compared to the stability model, the cross-lagged model was supported.
The goodness of fit of all the tested models was judged by the following goodness-of fit indices: (1) χ2 test, (2) root mean square error of approximation (RMSEA), (3) the Tucker-Lewis Index (TLI), and Comparative Fit Index (CFI). A non-significant χ2 test indicates a good fit. RMSEA with values of ≤0.05 indicate a good fit, values of 0.06–0.08 a reasonable fit, and values ≥0.10 a poor fit. TLI and CFI values range between 0–1; values of ≥0.90 are considered to indicate an acceptable fit of the model (36, 37).
Results
Cross-lagged relationships between OC and effort and reward
We first investigated cross-lagged associations between OC, and reward and effort. We started our SEM analyses by estimating a series of stability models with control variables. Weekly working hours (almost all participants worked full time) did not associate significantly with effort, reward, and OC at any time points and it was therefore dropped from the stability model. However, job changes between the study waves showed some significant associations with effort, reward, and OC and it was therefore included as a control variable into the stability model at each time point. Furthermore, as reward was constructed as a latent variable at each measurement point, we tested both a freely estimated stability model (model 1a; factor loadings in reward factors were allowed to vary across measurements) and a constrained stability model (model 1b: factor loadings were set to be equal across measurements). This approach was adopted to test whether the measurement properties of the reward scale remained the same across five measurement points, and to ensure that possible changes in the mean differences were not due to instability in its factor structure.
As seen in table 2, the Satorra-Bentler scaled chi2 difference test resulted in a non-significant loss-of-fit for model 1b as compared to 1a. Therefore, the further stability model comparisons were done against model 1b. We next tested a stability model where all cross-sectional associations between effort, reward, and OC were set to be equal across time (model 1c). The Satorra-Bentler scaled chi2 difference test produced a significant loss-of-fit for this model as compared to model 1b, and it was consequently rejected from our further analyses. Finally, we tested a stability model where the stability coefficients of each construct (effort, reward, OC) were set to be equal across time (model 1d). This model also provided a significant loss-of-fit compared to model 1b. As a result of these model comparisons, we chose model 1b as our baseline model in further cross-lagged analyses.
When comparing the cross-lagged models (models 2, 3, and 4) to the stability model 1b, the cross-lagged models showed a significant improvement in model fit (table 2). The normal causation model (model 2) and the reciprocal model (model 4) improved the model fit at the level of P<0.001, whereas in the case of the reversed causation model the improvement remained at the level of P<0.01. Consequently, the best fitting models (the normal causation and reciprocal models) were further compared to each other in subsequent analysis. The Satorra-Bentler scaled chi2 difference test indicated that the reciprocal model (model 4) did not improve the fit of the model as compared to the normal causality model (model 2). Furthermore, we checked the reversed cross-lagged paths in models 3 and 4 and none of them were significant. Thus, the normal causality model was concluded to be the best fitting.
The significant associations of the normal causation model are shown graphically in figure 1. As seen in the figure, OC showed higher stability over time than did effort or reward. Altogether four statistically significant cross-lagged effects were found. First, OC at T1 and T3 predicted rewards two years later: The more OC the participants reported, the less they reported receiving rewards in their work later on. Second, the more OC was reported at T2 and T3, the more effort was reported two years later. A closer examination of the control variables showed that those participants who had changed their job between T1 and T2 reported lower OC scores at T2 than those who had not changed their job. Job changes during the past two years were also associated with higher reward at T2, T3, and T4. Staying in same job, in turn, was found to be associated with lower effort at T2 and T3.
Figure 1
Normal causation model of the predictive associations between overcommitment (OC), effort (E), and reward (R). (Note: All hypothesized normal causation predictive associations were estimated and included in the model but only statistically significant associations are depicted in the figure. Job changes (JC) between the study waves are included in the model as a control variable for OC, E, and R at each follow-up time point but only statistically significant associations are depicted in the figure).
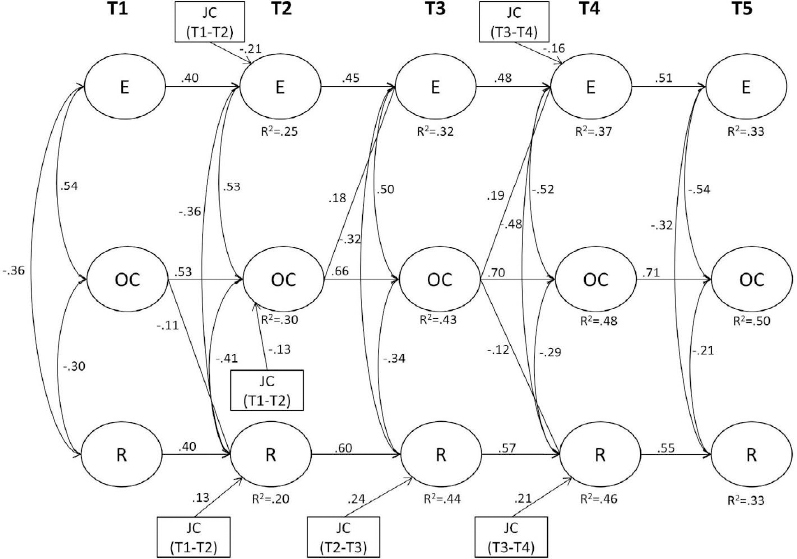
Cross-lagged relationships between ERI and OC
Table 3 shows the tested models for the ERI ratio and OC. Again, job changes between the study waves were included into all models as a control variable at each measurement point. Weekly working hours were dropped from the models due to the non-significant associations with the ERI and OC. The freely estimated stability model (model 1a) was chosen as our baseline model because the Satorra-Bentler chi2 difference test produced a significant loss-of-fit for the constrained stability models 1b (cross-sectional correlations between ERI and OC set to be equal across time) and 1c (stability coefficients of ERI and OC set to be equal across time).
Table 3
Goodness-of-fit statistics for structural equation models (SEM) including effort–reward imbalance (ERI) and overcommitment (OC) at T1, T2, T3, T4, and T5 (job changes between the study waves are controlled for in all SEM models) [RMSEA=root mean square error of approximation; TLI=Tucker-Lewis Index; CFI=Comparative Fit Index; ns=non-significant]
Tested SEM models | χ2 | df | RMSEA | TLI | CFI | Model Comparisons: Δ χ2 (df) |
---|---|---|---|---|---|---|
1a. Stability model, freely estimateda | 177.878 | 56 | 0.054 | 0.86 | 0.90 | |
1b. Stability model, constrainedb | 187.983 | 60 | 0.053 | 0.86 | 0.89 | 1b versus 1a: Δ χ2 (4), 10.82, P<0.01 |
1c. Stability model, constrainedc | 241.674 | 62 | 0.062 | 0.81 | 0.85 | 1c versus 1a: Δ χ2 (6), 43.31, P<0.001 |
2. Normal causation model | 148.140 | 52 | 0.050 | 0.88 | 0.92 | 2 versus 1a: Δ χ2 (4), 48.46, P<0.001 |
OC at T1 → ERI at T2 | ||||||
OC at T2 → ERI at T3 | ||||||
OC at T3 → ERI at T4 | ||||||
OC at T4 → ERI at T5 | ||||||
3. Reversed causation model | 179.354 | 52 | 0.057 | 0.84 | 0.89 | 3 versus 1a: Δ χ2 (4), 3.760, ns |
ERI at T1 → OC at T2 | ||||||
ERI at T2 → OC at T3 | . | |||||
ERI at T3 → OC at T4 | ||||||
ERI at T4 → OC at T5 | ||||||
4. Reciprocal causation model Conditions of models 2 and 3 are simultaneously valid | 149.480 | 48 | 0.050 | 0.86 | 0.91 | 4 versus 1a: Δ χ2 (8), 62.23, P<0.001 4 versus 2: Δ χ2 (4), 3.46, ns. |
As shown in table 3, the Satorra-Bentler scaled chi2 difference tests showed that the cross-lagged models 2 (causation model) and 4 (reciprocal causation) were statistically better models than the stability model 1a. The model 3 (reversed causation model) was rejected because Satorra-Bentler scaled difference test did not produce a significant improvement for its fit as compared to the stability model 1a. We further compared models 4 and 2 and found that the reciprocal model 4 did not improve the model fit as compared to the normal causation model 2. Thus, the normal causation model 2 was our final model.
The normal causation model 2 is shown in figure 2. A high level of OC at T1, T2, and T3 was associated with experiencing a strong imbalance between efforts and rewards two years later. Job changes between the baseline and T2 were associated with lower ERI and OC scores at T2. Job change between T3 and T4 associated also with lower ERI score at T4.
Figure 2
Normal causation model of the predictive assosiations between overcommitment (OC) and effort–reward imbalance (ERI). (Note: All hypothesized normal causation predictive associations were estimated and included in the model but only statistically significant associations are depicted in the figure. Job changes (JC) between the study waves are included in the model as a control variable for OC and ERI at each follow-up time point but only statistically significant associations are depicted in the figure).
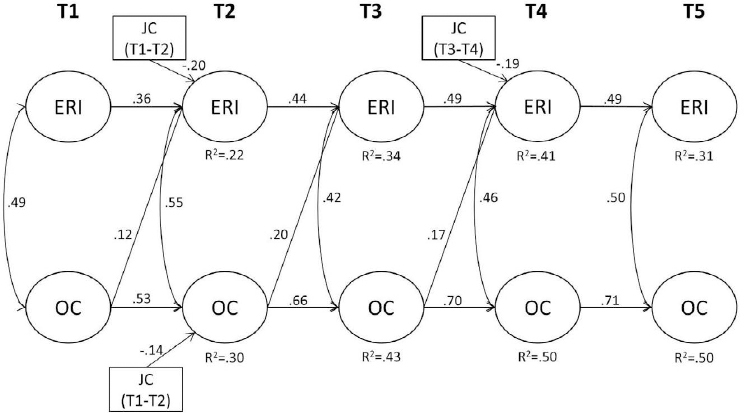
Discussion
The present longitudinal study tested the predictive associations of the core concepts included in the ERI model (6): effort, reward, ERI (contextual job-related characteristics), and OC (person-specific feature). More specifically, our focus was on the role of OC in the ERI model. Altogether three predictive associations between the concepts were tested and compared using cross-lagged analysis within the framework of SEM: (i) OC predicts later experiences of effort, reward, and ERI (H1: the hypothesized normal causation model), (ii) effort, reward, and ERI predict later OC (H2: the reversed causation model), (iii) both of the above conditions are simultaneously valid (H3: the reciprocal model).
Our results supported the hypothesized normal causation model (H1), indicating that the participants’ high OC predicted their later experiences of high effort (H1a), low reward (H1b), and high ERI (H1c). This finding is in accordance with the ERI model (6, 7), which shows that overcommitted employees tend to invest a lot of effort into their work – over the levels usually considered appropriate – and judge their extrinsic rewards to be low. As a result, OC increases the risk for perceiving an imbalance between the effort put into work and the reward gained from work, that is, ERI.
A notable finding was that participants’ OC predicted their experiences of imbalance across the three time periods (T1–T2, T2–T3, T3–T4), indicating the systematic role of OC as a risk factor for an increasing ERI. OC predicted also both high effort and low reward across two time periods: T2–T3, T3–T4, and T1–T2, T3–T4. The only time period where significant predictive cross-lagged associations were not found was the last follow-up period (T4–T5). This finding can be explained, at least partially, by the lower number of participants (ie, lower power) included in this time period. Thus, H1 received relatively strong support from our results.
As could be expected, OC as a personality feature showed higher rank-order stability across time points (two-year interval stability coefficients 0.53–0.71) compared to the stabilities of the context-dependent effort (0.40–0.51), reward (0.38–0.55), and ERI (0.36–0.49). The observed differences in the stabilities between OC and ERI indicators support their differentiated roles in the ERI model: as a more stable, personal characteristic, and more fluctuating, contextual characteristics. A further important finding was that the stability of OC increased over time, reaching its highest point at the last measurement period (T4–T5). Given the participants’ young age at the study baseline, this might indicate that the intrinsic personal characteristic of OC shows fewer changes with advancing age. This has also been observed in studies of personality traits and dispositions; that is, they become more stable with age (for a review, see 26; see also 38).
Strengths, limitations and avenues for future research
One notable strength of our study was its long follow-up time, across eight years. Our data also met the criteria of a full panel design (all the study constructs were measured at each measurement point), a dataset that is ideal for testing the cross-lagged effects between the study constructs under examination. As a result of these benefits, our data allowed us to establish the cross-lagged associations between OC and ERI indicators across four two-year time lags. As significant cross-lagged effects were found across more than one time period, we conclude that the finding of OC as the predictor of ERI indicators is valid.
Despite its strengths, our study also had certain limitations which should be remembered when drawing any final conclusions on the basis of the results. First of all, the long follow-up period resulted in increasing sample attrition over the measurements points because a small proportion of the participants at each of the first four measurement times (T1–T4) were unwilling to continue to participate in our study at the later stages. Also, some attrition occurred at each measurement point for unknown reasons. As noted above, the lower number of participants possibly weakened the statistical power of the cross-lagged effects at T4–T5. However, regarding the representativeness of the participants in the later measurements compared to the baseline measurement, no significant differences in background variables, OC, or ERI variables occurred. Thus, the participants in our longitudinal study represented well the participants in the baseline study with respect to the key study variables.
Secondly, our data were strongly dominated by males and engineering occupations. This may limit the generalizability of our findings to female-dominated and blue-collar occupations. The participants were also quite young at the baseline measurement, which limits the generalizability of the findings to older employees. Thus, in the future, the role of OC as the antecedent of ERI indicators could be investigated using a broader range of occupational samples.
Thirdly, our study tested the cross-lagged effects of OC and ERI indicators by using the well-validated ERI scale (7, see also 15, 20, 30). This indeed brought new insights to the dynamics of the theoretical ERI model and its measurement by showing the significance of OC as the antecedent of the ERI indicators. However, we remind that our study utilized the former 5-point response scale of the effort and reward sub-scales of the ERI questionnaire which covers also stress experiences caused by high effort and low reward. Currently, a 4-point response scale (strongly disagree – strongly agree) is recommended by Siegrist et al (39). At a time when our follow-up study started, the revised response format was not in use and it was not possible to change it in the middle of our follow-up data collection. Thus, it must be remembered that our findings cannot be directly generalized to the ERI research based on the revised response format.
Fourth, we investigated participants’ self-rated perceptions of their job-related efforts and rewards. Self-ratings do not necessarily provide a comprehensive picture of the dynamics of the ERI model in practice. Consequently, any future inquiry would greatly benefit from studying something more objective than self-reported perceptions of rewards and efforts in relation to OC. More objective indicators could include, for example, salary (reward) and weekly working hours (effort).
Finally, an important research question for future research stems directly from the current findings: Do ERI and its components actually mediate the associations between OC and health-related outcomes? Since previous research has already shown that both ERI and OC predict employees’ health problems and the current study shows that OC is an antecedent of ERI, it would be reasonable to expect that the effect of OC on health could be explained through the mediating role of ERI in this relationship. Longitudinal mediator analyses with OC (as a predictor), ERI (as a mediator), and health (as an outcome) are needed in future studies to empirically test this assumption.
Concluding remarks
As the first study investigating the predictive associations between the person- and job-related indicators of the ERI model, our findings make a significant contribution to the ERI research by showing that employees’ perceptions of failed reciprocity in their work can originate from their OC. In line with the basic idea of reciprocity in social exchange theories, the current findings indicate that high OC increases experiences of effort and decreases experiences of reward resulting in higher ERI. Thus, employees’ OC is a risk factor not only for problems with their health and well-being, as hypothesized in the ERI model (6, 9), but also for having adverse experiences in their working environment.
From an applied perspective, the present results will be of value for designing interventions aimed at promoting employee well-being in the work place. Our results suggest that, when implementing interventions, organizations should take into account the dynamic nature of employees’ job strain experiences, and particularly the role of individual differences in commitment to work. Practitioners need particularly to be aware that employees with high OC are at a greater risk for creating working conditions of poor social reciprocity for themselves, which in turn may lead to poorer health. A key target of such interventions could be that employees should find and reflect on their personal line of “good commitment” (promoting health and motivation) instead of “harmful overcommitment” (which exposes them to health problems). It is also important to include commitment as a part of the annual performance appraisal discussion between a supervisor and the subordinate.