Shift work is related to chronic health conditions associated with poor nutrition (1, 2). A recent meta-analysis investigating the diet of shift workers concluded that shift work does not alter energy intake (3). However, there is evidence to suggest that shift work may alter dietary composition (4–7). Although there has been an increasing interest in the diet of shift workers there has been limited investigation into changes associated with different shift types that may influence diet.
Due to circadian disruption and environmental influences, shift workers commonly experience sleep restriction (8). In turn, this may impact on diet. For example, sleep restriction has been associated with increased hunger (9), snack consumption (10), and alterations in macronutrient distribution (11). A limited number of studies have examined the sleep of their shift working participants in addition to investigating diet (7, 12–14). Only one of these studies has explored sleep duration as a predictor of diet. Results showed that shorter sleep durations were associated with higher carbohydrate intake, yet longer sleep durations were associated with a higher intake of fat (7). The link between sleep and the diet of shift workers should be explored further since improving sleep in shift workers may also improve diet.
In addition to sleep restriction, shift workers frequently report mood disturbances (1). A wide body of research has demonstrated that mood disturbances such as fatigue, depression, anxiety, and stress may influence dietary intake and choices (15–18). Despite this evidence, the relationship between mood and diet has not been widely examined in shift workers. Of the limited studies conducted in shift work populations, results show anxiety and hunger levels were higher following a night shift (14) and chronic fatigue in shift workers was associated with a higher intake of fat (7). These findings indicate that the link between mood and diet should be investigated further.
Taken together, findings suggest factors associated with shift work such as sleep restriction and mood disturbance could lead to alterations in diet. However, it is also important to consider how these variables are related to each other. Each shift type has a differential effect on circadian disruption and the amount and quality of sleep obtained (19). Furthermore, the level of circadian disruption experienced and duration of sleep obtained on different shifts may determine the extent that mood is disturbed. For example, night shift workers operate in complete opposition to their internal body clock and as a result report short sleep durations and poorer sleep quality (1, 8). Similarly, laboratory studies show that mood is affected by both circadian disruption and sleep restriction (20, 21). In addition to the relationships between sleep and mood there are a number of other work-related and demographic factors associated with dietary intake and composition in shift workers and the general population such as shift work history (22), body mass index (BMI) (23), and gender (24). To understand how shift work may influence diet, the relationships between all factors needs to be understood.
The current study will investigate a proposed pathway to explain how shift work alters diet (see figure 1). Research has established that shift type influences sleep, and that both shift type and sleep influence mood. Expanding on these known relationships, this study will investigate whether differences in shift type, sleep, and mood are associated with differences in macronutrient profile and energy consumed in a group of shift working nurses.
Material
Study design
The current study employed a repeated measures within and between subjects design. The study was conducted over a nine month period (2012–2013). Ethics approval was obtained from the University of South Australia Human Research Ethics Committee (Ethics Protocol: 0000027799) and from SA Health (Ethics Protocol: HREC/12/TQEHLMH/34).
Participants
In total 52 shift working nurses (46 female) were recruited to participate in the current study. Volunteers were recruited from three public metropolitan hospitals and were employed on one of the following shifts: morning (07:00–15:30), afternoon (13:00–21:30) or night (21:00–07:30) shift or a combination of these shifts. Table 1 depicts the combination of shifts worked by participants in this study and table 2 depicts characteristics. Participants were included in the study if they were over the age of 18 years and employed on a shift work schedule at one of the three hospitals. Participants were excluded from the study if they were pregnant or if they reported a health condition effecting dietary intake such as Crohn’s disease. Final analyses included 40 nurses across 181 shifts (see details in the statistical analysis section below).
Table 1
Combination of shift types worked during the study period
Shift type | Number of participants working shift combination |
---|---|
Morning | 2 |
Afternoon | 1 |
Night | 4 |
Morning, afternoon | 16 |
Morning, night | 1 |
Afternoon, night | 3 |
Morning, night, afternoon | 13 |
Table 2
Demographic and work related characteristics of the study population. [BMI=body mass index; SD=standard deviation]
Procedure
Information sessions were held in wards at each hospital. Nurses were provided with general information about the study and an information sheet. Interested volunteers were asked to contact the research team to arrange a one-on-one meeting to allow for a more detailed explanation of the study and the materials involved. If the volunteer wished to proceed they were asked to sign a consent form and were given a study pack which included a log book and an activity monitor (Actiwatch 2, Respironics Inc., USA, 2009).
Participants completed the study for a total of 14 consecutive days. During the 14-day period, participants were asked to wear the activity monitor around their non-dominant wrist at all times, except in situations where the watch may get wet (eg, showering). Participants were also required to complete their log book which included a mood scale and a sleep, work, and food diary. Participants completed the mood scales twice per working day (at the start and end of each shift). Sleep and work diaries were completed daily and the food diary was completed on three days per shift type and on one day off. As work schedules varied, the number of days the food diary was completed depended on the variety of shifts types worked during the study period. Participants working three shift types (eg, morning, afternoon, and night shift) completed the food diary for a minimum of ten days. Participants working only one shift type (eg, morning shift only) completed the food diary for a minimum of four days. Participants also completed a demographic questionnaire on a modified version of the General Information Questionnaire (GIQ) from the Standard Shiftwork Index (SSI) (25). A member of the research team returned to the hospital at the end of the 14-day period to collect completed log books and activity monitors.
Measures
Demographic information was completed on a modified version of the GIQ from the SSI. The GIQ requires participants to complete demographic details (eg, marital status), questions relating to work (eg, shift work history) and shift type (eg, types of shifts worked). The GIQ also includes questions relating to health and lifestyle such as smoking status and height and weight (to allow BMI to be calculated).
Sleep was measured objectively using a wrist activity monitor (Actiwatch 2, Respironics Inc, USA, 2009). Data from the activity monitors were derived from Actiware sleep software (Actiware – sleep software, Respironics Inc, USA, 2009). A measure of sleep duration was determined using the period between sleep onset time and wake time including naps in each 24-hour period prior to the beginning of a shift (subtracting any period of wake during this time). Sleep efficiency was calculated as time asleep/total time in bed × 100 (as a percentage). Participants recorded all sleep periods including naps in sleep diaries for the duration of the study. Data were compared with sleep diaries to validate recordings and give an overall picture of wake and sleep (including naps) during the 14-day period of the study. Participants also rated sleep quality daily on a scale of 1‒6 in their sleep diary with higher scores indicating a poorer quality of sleep. Sleep diaries have been found to be a valid and reliable measure for studying sleep quality (26). Activity monitors and the associated algorithms have been validated in field and laboratory studies (27, 28).
Visual analog scales (VAS) were used to measure moods hypothesized to be associated with diet (happiness, anxiety, depressive mood, stress, and tiredness). A separate scale was used to assess each mood. Participants were asked to respond to questions such as “how depressed do you feel” by placing a vertical line on a 100 mm scale representing how they felt at that point of time. The scale was anchored with “not at all” on the far left-hand side of the scale and “extremely” on the far right-hand side of the scale. A score of 0‒100 was obtained for each domain. Scores prior to the start of the shift and scores at the end of the shift for each mood domain were averaged to obtain a daily mood score for each mood domain per study day. VAS have been found to be a reliable and valid measurement for studying mood in numerous studies (29).
Food and drink consumption was recorded using food diaries. Participants were asked to keep a record of all food and drink consumed on three days per shift type and on one day off. Participants were asked to record the method of cooking (eg, boiled pasta), the type of food consumed (eg, whole meal bread) and the quantity of food (eg, two teaspoons of white sugar or the weight in grams). Participants were asked to measure food using weights and common household measurements. In addition, participants were asked to provide the brand names of foods and recipes where available. Participants were asked to complete the diary as soon as possible after meals and snacks. Concurrent validity has been demonstrated in food diaries for food and nutrient intake. For example, correlation coefficients have ranged from 0.68‒0.97 for food intake and 0.75‒0.92 for nutrient intake (30). Foodworks nutrient analyses software (Version 7,00, Xyris Software Australia, Kenmore Hills, QLD, Australia) was used to determine daily energy intake in kilojoules (kJ) (1 calorie = 4.184 kJ) and macronutrients (in grams). The database selected was NUTTAB 2010 (Food Standards Australia, New Zealand). In order to maximize the strength of the repeated measures, design data from each day were extracted separately for analysis rather than averages per shift type.
Statistical analysis
Sample. We recruited 52 shift working nurses to participate in the study, and 12 were excluded from the final analyses. Of these, 2 did not return their log books, 4 did not complete their food diaries correctly, 1 reported a health condition and 1 reported being pregnant. Additionally, 2 participants were excluded as their actigraphy data could not be retrieved and 2 participants had missing mood data such that we were unable to obtain a daily average. Therefore, the final sample included 40 participants, across a total of 181 shifts.
Missing data. Data were checked for missing values and screened for normality. There were no clear patterns in missing data across participants or variables. Less than 5% of data were missing for all variables except for BMI (18% missing), sleep quality (8% missing), and sleep efficiency (6% missing). Given that we used mixed effects models (with a random effect of subjectID), which deal well with missing data (31), the amount of missing data was low, and there was no clear bias suggested, missing data were not replaced.
Coding of variables. Marital status was categorized into ‘married’ and ‘not married’ (divorced, widowed or single) and dependents were categorized into ‘yes’ (dependent <18 years currently living with the participant) or ‘no’. Sleep duration was calculated as the total amount of sleep obtained in each 24-hour period including naps (subtracting any period of wake during sleep episodes) prior to the beginning of a shift. The two mood scores (before and after each shift) for each day were averaged to determine a total daily mood score for each mood variable. Energy intake and dietary profiles were analyzed as the total energy and macronutrient content of food and drink consumed during the 24-hour period (ie, 24:00‒24:00 hours) to capture the influence of the shift on the diet for the whole day, rather than simply looking at on-shift diet. Macronutrients were converted to a percentage of daily energy intake in kJ.
Univariate exploration. Analyses were conducted using STATA version 15.0 (StataCorp, College Station, TX, USA). A significance level of P≤0.05 was used to determine significance. Univariate regression models with a random effect of participant ID, to take into account repeated measurements for each participant (31), were conducted to determine differences in predictor variables and dietary intake by shift type. Shift type was specified as a categorical variable [morning (M)/afternoon (A)/night (N), with afternoon shift as the reference level). Given that these analyses were exploratory, differences between shifts were examined using tests of linear hypotheses after model estimation for pairwise shift type combinations (M-A, M-N, A-N). These were used to set reference categories for the models in the path analyses (outlined below).
Additional univariate associations were explored using correlations. Pearson correlations were calculated for all continuous variables. Magnitudes of correlations were interpreted as small 0.1 < r < 0.3; moderate 0.3 < r <0.5; and large r >0.5 (32). There was a large correlation between anxiousness, depression, and stress (r ≥ 0.8), and therefore only stress was included in the final model to avoid potential multicollinearity issues. In this way, results for stress are interpretable more broadly as negative mood. Therefore, the mood variables that were included in the path analyses below, were happiness, tiredness, and stress.
Path analyses. Multivariate relationships were investigated using generalized structural equation modelling (GSEM) with a random effect of participant ID (participants completed different shift combinations and therefore were represented in the model by different numbers of data points, final number of observations for each model is provided in the results section). Models were conducted, organized by dependent variable (DV), from right to left on figure 1.
Relationships between shift type, sleep, mood, and diet
Separate models specified DV of energy intake (kJ) and macronutrients (fat, protein, and carbohydrate expressed as a percentage of energy intake). Predictor variables included shift type, which was specified as a categorical predictor (M/A/N), with the reference category set from results of the univariate exploration (above). For example, univariate analyses revealed that total energy intake was significantly lower on afternoon shifts than on morning or night shifts (P<0.01, table 3), and therefore, afternoon was set as the reference category for total energy intake. Significant main effects of shift type were further explored by post-model tests for pairwise shift type combinations. Predictors also included sleep (total sleep time, sleep efficiency, and subjective sleep quality) and mood (happiness, stress, and tiredness) variables (anxiety and depression were not included to avoid multicollinearity issues with stress, as explained above). Covariates included age, sex, BMI, marital status, smoking status, and total hours worked per week. Years worked in shift work and years worked in present shift system were not included to avoid multicollinearity arising from their large correlation with age (r=0.90 and r=0.80 respectively).
Table 3
Summary of estimated marginal means (MM) and standard errors (SE) from regression models (with a random effect of participant ID) to test differences in mood, sleep, and dietary intake by shift type. [M-A=morning versus afternoon; M-N=morning versus night; A-N=afternoon versus night; TST=total sleep time; kJ=kilojoules (1 calorie = 4.184 kJ).]
Morning | Afternoon | Night | M-Ab | M-Nb | A-Nb | ||||||||||
---|---|---|---|---|---|---|---|---|---|---|---|---|---|---|---|
|
|
|
|
|
|
||||||||||
MM | SE | Na | MM | SE | Na | MM | SE | Na | INLINE | P-value | INLINE | P-value | INLINE | P-value | |
Mood ratings (0‒100) | |||||||||||||||
Happiness | 53.4 | 2.5 | 79 | 56.2 | 2.5 | 77 | 48.7 | 2.9 | 38 | 2.0 | 0.15 | 3.4 | 0.06 | 8.8 | <0.01 |
Anxiety | 23.9 | 3.1 | 79 | 20.8 | 3.1 | 78 | 25.3 | 3.5 | 38 | 2.0 | 0.16 | 0.3 | 0.61 | 2.5 | 0.11 |
Depression | 24.3 | 3.2 | 79 | 21.9 | 3.2 | 78 | 25.0 | 3.6 | 38 | 1.3 | 0.25 | 0.1 | 0.74 | 1.4 | 0.24 |
Stress | 29.4 | 3.3 | 79 | 23.9 | 3.3 | 78 | 29.6 | 3.7 | 38 | 6.1 | 0.01 | 0.0 | 0.94 | 3.8 | 0.05 |
Tiredness | 55.9 | 3.1 | 79 | 44.8 | 3.1 | 77 | 62.0 | 3.6 | 38 | 21.7 | <0.01 | 3.7 | 0.05 | 30.8 | <0.01 |
Sleep | |||||||||||||||
TST (hours) | 5.8 | 0.2 | 79 | 7.5 | 0.2 | 77 | 7.3 | 0.3 | 37 | 33.6 | <0.01 | 14.9 | <0.01 | 0.7 | 0.41 |
Efficiency (%) | 86.1 | 1.0 | 75 | 87.6 | 1.0 | 73 | 85.0 | 1.3 | 36 | 1.6 | 0.21 | 0.5 | 0.47 | 3.0 | 0.08 |
Qualityc | 2.7 | 0.2 | 74 | 2.4 | 0.2 | 72 | 3.4 | 0.2 | 35 | 1.6 | 0.21 | 5.1 | 0.02 | 10.6 | <0.01 |
Diet | |||||||||||||||
Energy (kJ) | 8600 | 0.5 | 80 | 6800 | 0.5 | 78 | 8800 | 0.6 | 39 | 17.3 | <0.01 | 0.3 | 0.62 | 13.6 | <0.01 |
Fat (%)d | 30.1 | 1.1 | 80 | 30.5 | 1.1 | 78 | 31.3 | 1.5 | 39 | 0.1 | 0.78 | 0.1 | 0.78 | 0.3 | 0.61 |
Saturated fat (%)d | 12.7 | 0.6 | 80 | 11.5 | 0.6 | 78 | 11.8 | 0.8 | 39 | 2.9 | 0.09 | 1.1 | 0.30 | 0.1 | 0.73 |
Carbohydrates (%)d | 40.5 | 1.7 | 80 | 43.3 | 1.7 | 78 | 47.0 | 2.1 | 39 | 2.6 | 0.11 | 8.4 | <0.01 | 2.8 | 0.09 |
Protein (%)d | 21.6 | 1.3 | 80 | 21.4 | 1.1 | 78 | 18.4 | 1.4 | 39 | <0.01 | 0.91 | 4.6 | 0.03 | 4.3 | 0.04 |
Relationships between shift type, sleep, and mood
Separate models specified DV of mood (happiness, stress, and tiredness), with a categorical predictor of shift type (M/A/N, reference category set from univariate results). Predictors also included sleep variables (total sleep time, sleep efficiency and subjective sleep quality), with covariates of age, sex, BMI, marital status, smoking status, and total hours worked per week.
Relationship between shift type and sleep (DV)
Separate models specified DV of sleep variables (total sleep time, sleep efficiency, and subjective sleep quality), with a categorical predictor of shift type (M/A/N, reference category set from univariate results), and covariates of age, sex, BMI, marital status, smoking status, and total hours worked per week.
Initially, full models were run as specified above. Then predictors were selected using purposive selection of covariates (33), which is an alternative to stepwise modelling. After running initial models, all non-significant variables were then removed, and the preliminary model was re-run including only significant variables (this is referred to as the preliminary main effects model). Following this, each non-significant variable was returned to the preliminary main effects model one at a time and was retained if it either was significant or significantly impacted the relationship between another predictor and the dependent variable. This method allows examination of the contributions of each variable to the overall model in the context of the other predictors. The models presented in the results section are the final models, re-run with purposively selected predictors only.
Component models were used to construct the pathways between shift type, sleep and mood as these variables come together to predict diet (controlling for covariates), and these path diagrams are presented in the results section. In these path diagrams, for ease of illustration, a single path weight is presented for shift type, with reference category determined from univariate analyses, as outlined above, relative to the two other shift types. Coefficients for the differences between specific shift types are also provided in text. Mediation effects (indirect effects) (34, 35), are presented with bootstrapped standard errors and z-tests.
It is relatively well established that shift type influences sleep and that both shift type and sleep influence mood. Since the focus of this manuscript was to focus on how these factors together may influence diet, results of regression models are only presented for pathways that lead to a dietary dependent variable. For example, while expected relationships were seen between shift type, total sleep time, and sleep quality, none of these sleep variables were shown to be significantly associated with mood or diet in this sample, and so are not included in the results section.
Given that there were such a small number of males (N=5), models were run controlling for, rather than stratifying for gender. However, models were repeated removing males, and results were not substantively different.
Results
Demographics
Participants (N=40) were aged 22‒63 years and the majority were female (table 2). On average, participants were overweight according to BMI. The majority of participants did not smoke. Just over half of the participants were married and just under half of the participants reported that they were currently living with dependents aged <18 years. Participants reported that they had been employed in shift work for approximately 15 years on average. Just over half of the nurses (58%) reported they typically worked a slow rotating schedule (working the same shift for five days). However, during the study period most nurses rotated shifts rapidly (every one to two days). Of all participants, 17% reported regularly working overtime. Participants reported working approximately 39 hours per week during the study period.
Univariate analyses (table 3) showed participants reported feeling significantly less happy on night shifts compared to afternoons (P=0.01). On average participants reported lower ratings of anxiety, depression, and stress on afternoon shifts. Stress ratings were significantly lower on afternoons than mornings (P=0.01) or nights (P=0.05). Participants reported lowest tiredness on afternoons (P<0.01) and highest on night shifts. On average, sleep duration in the 24-hour period prior to a shift was 6.9 hours, with shortest sleep durations prior to morning shifts (P<0.01) and longest durations prior to afternoon shifts. Lowest energy intakes were consumed on days that afternoons shifts were worked (P<0.01). Carbohydrate intake was significantly higher on night shifts compared to mornings (P<0.01). Protein was lower on nights than on mornings (P=0.03) or afternoons (P=0.04).
Path analyses ‒ relationships between shift type, sleep, mood, and diet
Energy (kJ). Controlling for sex, shift type, and stress rating were significant predictors of energy intake (figure 2, inset). Relative to mornings and nights, intake on afternoon shifts was 1659 kJ lower, (representing approximately 20% of the reported average intake for this sample, mean 7899 kJ). When compared separately to afternoon shifts, parameter estimates revealed that the differences were biggest between afternoon and night shifts (night: ß=1897.7, z=3.5, P<0.001; morning: ß=1556.1, z=3.6, P<0.001). These are medium effects (d=0.5 and 0.4 respectively). The difference between morning and night shifts was small and not significant (χ12=0.39, P=0.531). Every unit rating increase in the 100-point stress scale was associated with a 35 kJ increase in energy intake, which equates to an increase of 753 kJ for a one standard-deviation (SD 21.5) change in stress rating (or 9.5% of the reported average intake for this sample). Relative to mornings and nights, stress ratings on afternoon shifts were 5.6 points lower. Bootstrapped estimates indicated that the total indirect pathway from shift type to energy via stress rating was significant (ß=-198.2, standard error=102.7, z=-1.9, P=0.05), representing approximately 9% of the total effect (from both indirect and direct pathways).
Figure 2
Path analysis models showing predictors of energy and macronutrient intake. Controlling for sex, shift type was a significant predictor of energy intake and of stress. In turn, stress was also a significant predictor of energy intake (upper left inset). Stress was a significant predictor of both fat and saturated fat intake (upper right). Shift type and sleep efficiency were significant predictors of carbohydrate intake (lower left). Controlling for sex, shift type and sleep efficiency were significant predictors of protein intake (lower right). Paths display parameter estimates and 95% confidence intervals. Rectangles denote measured variables with parentheses indicating the reference value for categorical variables, double circles denote random effects (participant ID), ε denotes error. Numbers in the lower right corners of the boxes for dependent variables denote the model intercept. Models for energy and fat, include 181 observations. The model for carbohydrate and protein include 170 observations due to missing values for sleep efficiency. Models show significant predictors only (P<0.05) following purposive selection of covariates (33). Initial models for diet variables started with 13 predictors, and purposive selection involved 11 model runs for energy and carbohydrate, 13 for fat and saturated fat, and 10 for protein to identify final models. The initial model for stress included 10 predictors, and involved 11 runs to identify the final model.
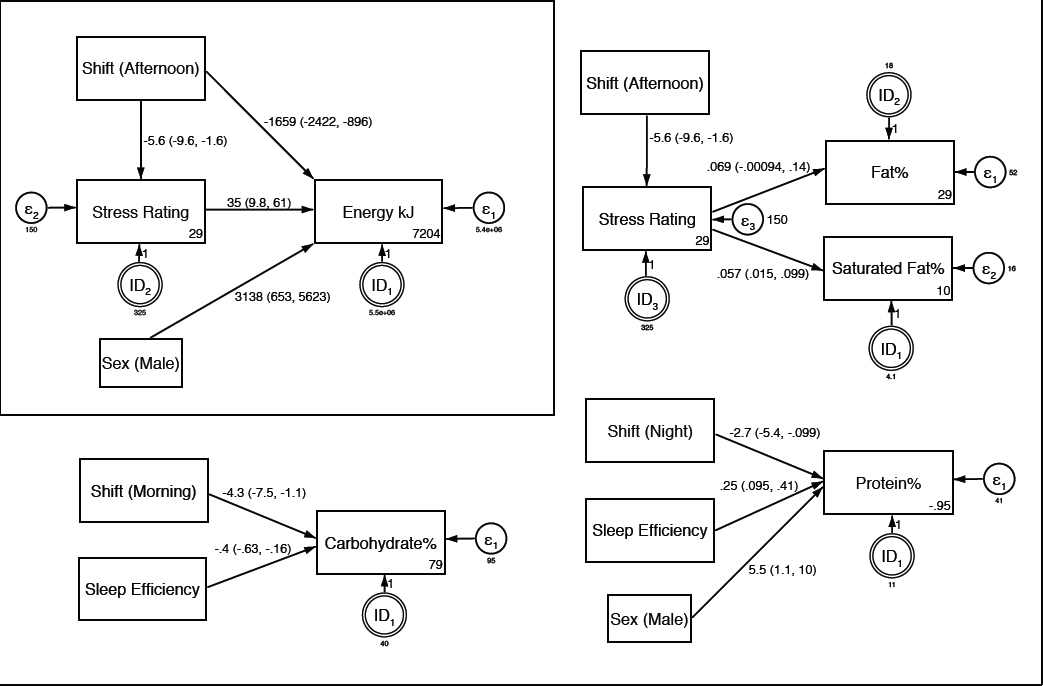
Fat and saturated fat. Stress rating was a significant predictor of fat intake as a percentage of total energy (figure 2, upper right). Every unit rating increase in the 100-point stress scale was associated with a 0.07% increase in fat intake, which equates to an increase of 1.5% for a one standard-deviation (SD 21.5) change in stress rating (or 4.8% of the reported average intake for this sample, mean=31%). Every unit rating increase in the stress scale was also associated with a 0.06% increase in saturated fat intake, which equates to an increase of 1.2% for a one standard deviation change in stress rating (or 10% of the reported average intake for this sample, mean=12%). Bootstrapped estimates indicated that the total indirect pathways from shift type to fat (ß=-0.39, standard error=0.29, z=-1.3, P=0.185) and saturated fat (ß=-0.31, standard error=0.20, z=-1.6, P=0.110) via stress rating were small effects (approximate d=0.1) and were not significant.
Carbohydrate. Shift type and sleep efficiency were significant predictors of carbohydrate intake as a percentage of total energy (figure 2, lower left). Relative to afternoons and nights, intake on morning shifts was 4.3% lower (representing approximately 10% of the reported average intake for this sample, mean 43%). When compared separately to morning shifts, parameter estimates revealed that the differences were biggest between morning and night shifts (night: ß=6.1, z=2.7, P=0.006; afternoon: ß=3.4, z=1.9, P=0.052). These are small to medium effects (d=0.5 and 0.3 respectively). The difference between afternoon and night shifts was small and not significant (χ12=1.4, P=0.230). Every unit increase in sleep efficiency (maximum of 100%) was associated with an 0.4% decrease in carbohydrates, which equates to a decrease of 3% for a one standard-deviation (SD 7.4%) change in sleep efficiency (or 7% of the reported average intake for this sample, mean 43%).
Protein. Controlling for sex, shift type and sleep efficiency were significant predictors of protein intake as a percentage of total energy (figure 2, lower right). Relative to afternoons and mornings, intake on night shifts was 2.7% lower (representing approximately 13% of the reported average intake for this sample, mean 21%). When compared separately to night shifts, parameter estimates revealed that the differences were larger for morning shifts (morning: ß=3.0, z=2.0, P=0.042; afternoon: ß=2.5, z=1.7, P=0.092). These are small to medium effects (d=0.4 and 0.3 respectively). The difference between morning and afternoon shifts was trivial and not significant (χ12=0.2, P=0.675). Every unit increase in sleep efficiency was associated with an 0.25% increase in protein, which equates to an increase of 1.9% for a one standard-deviation (SD=7.4%) change in sleep efficiency (or 9% of the reported average intake for this sample, mean 21%).
Discussion
Findings indicated that shift type, sleep efficiency, and mood were associated with energy intake and macronutrient profile. Specifically, compared to other shift types, nurses consumed less energy on afternoon shift, carbohydrate intake was lower on morning shift, and protein intake was lower on night shift. Higher stress ratings were associated with a higher energy intake and a higher percentage of fat and saturated fat in the diet. Lower sleep efficiency was associated with a lower carbohydrate and higher protein intake.
Results suggest that the association between shift type and energy intake could be mediated by mood, with analyses supporting pathways directly from shift type to energy intake, and indirectly via stress. Stress, anxiety, and depression were highly correlated and therefore, the illustrated relationships between diet and stress can be broadly interpreted as relationships between diet and indicators of negative mood (ie, encompassing stress, anxiety, and depression symptoms). The relationship between negative mood and increased food intake is consistent with previous research (16, 17). The finding that night shifts and morning shifts were associated with more negative mood than afternoon shifts is also consistent with previous research, with suggestions that this may be related, not only to circadian fluctuations in mood but also to differences in workload (20, 34, 35). In addition, findings showed that poorer mood was associated with a higher intake of fat and saturated fat which concurs with prior studies (18). It is possible that the nurses in the current study were using food as a coping strategy for dealing with negative mood states (36, 37). Investigating ways to improve the mood of shift workers may in turn assist with reduced consumption of food and healthier food choices.
Although dietary measurements reflect the 24-hour day, mood ratings were only reported at the start and end of each shift. Therefore, it is unlikely that the measurements capture the variation over the entire shift. Future research with more frequent sampling of mood measures across a shift would help to identify within-shift fluctuations in mood. Moreover, repeated mood measurement across the night shift would facilitate investigation of mood pre- and post- food consumption during the nightshift. This would assist with the interesting question of whether mood changes drive food consumption or vice versa. Indeed, the relationship between sleep, mood, and diet is likely to be bidirectional. A strength of the current study was that sleep was examined prior to mood and diet on shift, which provides support for this pathway. However, the design of this study did not allow us to unpack directionality between mood and diet. Carefully designed longitudinal studies will be required in the future to examine possible bidirectional relationships between mood and diet.
The finding that nurses had a lower percentage of carbohydrate in their diet on morning shift compared to night and afternoon shift may be explained by circadian influences on food selection. There is evidence to suggest that preference for foods higher in carbohydrates such as sweet and/or starchy foods peaks during the evening (38, 39). Nurses appeared to be trading protein for carbohydrates on night shift as findings showed a lower proportion of protein on night shift. This highlights the need for examining diet quality in further studies of shift work and diet. For example, many snack foods such as cakes and lollies contain simple carbohydrates and are higher in energy, promoting weight gain. However, foods such as fruits and vegetables are higher in starch and natural sugars but also contain beneficial nutrients such as vitamins, minerals and fiber (40). Understanding differences in food preferences by time-of-day, combined with recommendations for high quality options to match these preferences, may assist in the promotion of shift worker health.
In this sample, the only sleep-related variable associated with dietary changes was sleep efficiency. Laboratory studies show that sleep restriction is linked to increased hunger, energy intake, and alterations in macronutrient distribution (9, 11). Participants in the aforementioned laboratory studies have had their sleep restricted to 4–5 hours per night. Participants in the current study reported relatively more sleep, with 6–7 hours of total sleep time, even prior to a night shift (likely reflecting preparatory napping). However, with estimated sleep efficiency at approximately 85%, and with relatively poor sleep quality ratings, it may be that for this group of workers, sleep quality was more important than duration in the association with diet. This may not be the case for workers experiencing more severe sleep time restriction. Results of the current study underscore the importance of examining sleep quality indicators in addition to sleep duration.
The design of the current study allowed for the examination of variables within and between participants, and measures were repeated across the 14-day study period, therefore reducing variability due to individual differences. This is important as previous studies that have mostly been cross sectional or have compared shift workers to day workers have produced mixed results (3–7), which may reflect individual differences in dietary intake. While the repeated-measures aspect of the study is a strength, the sample size was still relatively small (42 participants with 181 shifts). The effect sizes found for relationships in this study ranged from trivial to moderate. With a larger sample size, the small effects of the indirect (mediation) pathway from shift type to fat intake may have been found to be significant, for example. This highlights the need to replicate these findings with larger sample sizes. Furthermore, sleep was estimated objectively via actigraphy, which is a more accurate measure of sleep patterns (compared to polysomnographic measures) than self-reports alone. Additionally, diet was measured by food diaries which are considered to be gold standard when measuring diet in a field environment (41). However, it is important to note that diet-related studies often attract participants with an interest in health and nutrition (41) and this bias may have occurred in the current sample as participants were offered a nutritional report upon completion of the study. Furthermore, this study included mostly female participants who were in the overweight range, according to their BMI. This may have influenced findings as females and individuals with a higher BMI are more likely to under-report food intake (42). Importantly however, while this may have altered the total food reported by an individual it is unlikely to have been different across shift types. Although participants were asked to complete their diaries immediately following food consumption, it is possible that these instructions were not followed consistently which may have resulted in difficulties recalling food consumed.
It is also important to note that there may have been a number of factors associated with shift type not examined in the current study that also influence food choice. For example, food purchasing options vary depending on shift. Whilst the cafeterias at each of the three hospitals are open on the weekend, they are not open at night. Therefore, nurses on afternoon and night shift may have only been able to purchase food from vending machines if they did not bring food with them. Additionally, although the Nurses Working Agreement (South Australia) states that nurses are entitled to two 30-minute breaks per shift, it is not known if nurses took these breaks and whether this influenced opportunities to consume meals, or to snack, particularly on busy shifts. Furthermore, caffeine and alcohol consumption were not considered in this analysis and both may impact both sleep, appetite, and mood (43–45). These are important variables to consider examining in future studies.
In conclusion, this study showed that dietary composition and intake are influenced by shift type. However, it is also important to consider associated factors such as mood and sleep as they can also influence food intake and preferences. Results suggest that compared to nights and mornings, afternoon shift was associated with reduced energy consumption, and that the relationship between shift type and energy intake may be mediated by mood (stress, depression, anxiety). Negative mood was also associated with higher fat intake. Dietary interventions for shift workers should consider the role of mood as well as shift type. Future studies should examine how mood fluctuates across shifts, and whether interventions to improve mood in shift workers may have positive flow on effects for diet. Additionally, diet quality should be assessed in future studies as this will provide a more complete picture of the diet of shift workers and may assist with health promotion strategies.