The health benefits of physical activity and health hazards of sedentary behavior are well documented (1, 2). Sedentary individuals are more likely to develop cardiovascular diseases, type 2 diabetes, and metabolic syndrome (3). In contrast, physically active individuals have a lowered risk of coronary heart disease and type 2 diabetes (4).
However, this evidence is mainly based on studies using questionnaire-based information on time spent sedentary and in physical activity, which can be biased (5). For example, individuals have been shown to underestimate their self-reported daily sedentary time by 15–43% (6–8) and overestimate their self-reported physical activity level by 36–173%, compared to objective measurements (9). Both under- and over-reporting of time spent sedentary and in physical activity will lead to biased associations between these exposures and health outcomes.
The extent of self-report bias may depend on participant characteristics. Individual factors, like body mass index (BMI) (10), musculoskeletal pain (11), education (12), leisure-time physical activity (13), and occupation (12, 14) have been shown to influence the extent of under- or over-reporting of sedentary behavior and physical activity. This property of self-report bias, ie, that the error in self-reported information depends on the level of the factor, is known as differential bias. For example, obese participants appear to over-report physical activity to a larger extent than non-obese participants (10, 15). This differential bias will lead to associations between physical activity and health being even more attenuated in an obese population than in a non-obese one. Thus, in studies based on self-reports, associations between sedentary behavior or physical activity and health need to be adjusted for self-report bias, and, in order to arrive at a proper adjustment, knowledge about individual characteristics influencing that bias is required.
Valid assessment of the size and properties of differential bias in self-reports requires that accurate measurements of sedentary behavior and physical activity are available. For this purpose, accelerometer-based reference methods have been used, identifying activities on the basis of accelerometer counts per minute (16). Activity discrimination thresholds have not been derived empirically and they have been criticized for their poor ability to differentiate between sedentary behaviors and physical activities (17), such as between sitting postures and stationary standing (18). Differential bias in self-reports of sedentary behavior and physical activity should therefore be addressed using reference methods that identify postures and activities with a better validity.
Using accelerometer-based data as the reference, the aim of this study was to investigate the extent to which age, gender, BMI and low-back pain (LBP) introduce differential bias in self-reported information on time spent sedentary and in physical activity among blue-collar workers.
Methods
Study design and population
This study is based on data from the cross-sectional New method for Objective Measurements of physical Activity in Daily living (NOMAD) study (19, 20). Data were collected from October 2011 to April 2012 at seven Danish workplaces engaged in a variety of blue-collar occupations (construction, cleaning, garbage collection, manufacturing, assembling, mobile plant operations, and healthcare services). We recruited 358 workers with support from trade unions or safety representatives at the individual workplaces. Workers were excluded if they were pregnant, had fever during the measurements, or were allergic to adhesives. Inclusion criteria were work in the primary occupation for ≥20 hours per week and age 18–65 years.
All workers provided their written informed consent prior to participation. The Danish Data Protection Agency and local Ethics Committee (The Capital Region of Denmark; journal number: H-2–2011–047) approved the NOMAD study which was conducted according to the Helsinki declaration.
Variables
Accelerometer-based time.
Workers wore one tri-axial Actigraph accelerometer (Actigraph GT3X+, Actigraph LLC, Florida, USA) on the right thigh (21) for four consecutive days (4×24 hours), including at least two working days. During these days, workers were instructed to register their working hours, non-work time, when they went to bed and got out of bed, non-wear time, and time of reference measurement (ie, standing in an upright position for 15 seconds) in a paper diary (22).
All non-working days, non-wear periods and bedtime periods were excluded from further analysis according to previously reported criteria (20, 23). We then selected those workers who had at least one day with at least 23 hours of recorded data. A day was defined from midnight to midnight.
Time spent sedentary and in physical activity was determined using the customized MATLAB software Acti4, which has shown a good sensitivity and specificity in identifying body postures (sitting and lying) and different physical activities (ie, standing, walking, running, cycling, and stair climbing) in standardized conditions (21) and during occupational work (24). The measured exposures were subsequently averaged across available days for each worker.
Subsequently, the average time spent standing still, moving and slow walking was combined to obtain time spent on light physical activity (LPA), while time spent fast walking, running, stair climbing and cycling was combined to obtain moderate-to-vigorous physical activity (MVPA) time. Finally, times spent sedentary, in LPA, and MVPA were expressed in percent of average wear time per day.
Self-reported time
At the end of the last measurement day, workers completed a retrospective questionnaire regarding the average time spent lying, sitting, standing, slow and fast walking, running, and cycling per day during the measurement period. Responses were provided in hours and minutes and later converted to minutes. Subsequently, the times spent lying and sitting were added to represent sedentary time. Likewise, times spent standing and slow walking were added to represent LPA, and time spent fast walking, running, or cycling represented MVPA. Finally, these calculated variables were expressed in percent of the total measurement time.
Candidate differential bias factors
Age was determined using the Danish unique civil registration (CPR) number, and gender was determined using a question “are you male or female?”. BMI was determined from objective measurements of the height (m) and weight (kg) of the worker. LBP intensity was determined using a modified single item from the standardized Nordic questionnaire for the analysis of musculoskeletal symptoms (25). The single item was “please rate your worst intensity of pain in the lower back during the past month” with a response scale from 0 (“no pain”) to 9 (“worst imaginable pain”).
Statistics
For each participant, differences between self-reported and accelerometer-based values of sedentary time, LPA time and MVPA time (self-report – accelerometer) were calculated as measures of (dis)agreement. Values >50% were considered erroneous and excluded from further analyses. Summary agreement between self-reported and accelerometer-based values was described using Bland and Altman plots, in terms of bias (mean difference between the methods) and limits of agreement (mean - 1.96×SD to mean + 1.96×SD). Sedentary time, LPA, and MVPA were linear least-square regressed on age, gender, BMI and LBP intensity as well as the interaction between each of these factors and the accelerometer-based exposure, using the following general model:
Where, Y = difference self-report – accelerometer-based exposure; b0 = intercept; b1–b9 = regression coefficients; Eacc = accelerometer-based exposure (time sedentary, in LPA, or in MVPA). All variables were treated as continuous variables except gender (reference category=females).
If none of the interaction terms in a model were significant, a separate model without the interaction terms was run to analyze the main effect of each candidate bias factor:
Multicollinearity between independent variables, normality of the standardized residuals, and equality of variances were assessed to check if the data met the assumptions underlying linear regression models.
Time spent sedentary, in LPA and in MVPA adds up to 100%. Thus, time spent on LPA can be calculated from the other two. Results for LPA are therefore redundant and are not presented.
Statistical Package for the Social Sciences (SPSS, version 24, IBM, Armonk, NY, USA) was used for all statistical operations.
Results
A flow diagram of the participants is shown in appendix A (www.sjweh.fi/show_abstract.php?abstract_id=3693). Of 358 eligible blue-collar workers, 152 workers were included in the analyses since they volunteered to participate, answered all questions about sedentary behavior and physical activity, and had at least one work day with valid accelerometer-based measurements.
Five workers were excluded from the analyses of sedentary time and three workers from the analysis of MVPA time according to the exclusion criterion of a >50% disagreement between self-reports and accelerometer results. On average, the workers were 44.4 years old, and 61% were males (table 1). Fifty-nine percent of the workers were overweight (25.0–29.9 kg/m2) or obese (>30 kg/m2), while 15% had high LBP pain. According to the accelerometer measurements, workers were sedentary for 64% of the time, while 9% of the time was spent on MVPA. Hallman et al (26) reported further details on sedentary behavior in the population, including data stratified by work and leisure.
Table 1
Characteristics of study participants (N=147). [MVPA=moderate-to-vigorous physical activity.]
Variables | N | Mean | SD | % |
---|---|---|---|---|
Age (years) | 147 | 44.4 | 9.6 | |
Males | 90 | 61 | ||
Occupations | ||||
Mobile plant operators | 9 | 6 | ||
Workers in the health service sector | 10 | 7 | ||
Assembly workers | 17 | 11 | ||
Cleaners | 21 | 14 | ||
Construction workers | 26 | 17 | ||
Manufacturing workers | 48 | 32 | ||
Garbage collectors | 21 | 14 | ||
Body mass index (kg/m2) | 147 | 26.4 | 4.8 | |
>30.0 | 26 | 18 | ||
25.0–29.9 | 61 | 42 | ||
<25.0 | 60 | 41 | ||
Low back pain (LBP) (0–9) a | 143 | 2.9 | 2.4 | |
High pain | 22 | 15 | ||
Mild/no pain | 121 | 85 | ||
Self-reported sedentary time (%) | 147 | 62.4 | 14.5 | |
Self-reported MVPA time (%) | 147 | 14.3 | 13.1 | |
Objectively measured sedentary time (%) | 147 | 63.8 | 9.7 | |
Objectively measured MVPA time (%) | 147 | 8.9 | 3.2 |
Bland & Altman plots (Figure 1) showed that the mean difference between self-reported and accelerometer-based measurements was -1.5% for sedentary time (95% CI -3.6–0.7%, P=0.19), and 5.5% for MVPA time (95% CI 3.7–7.5%, P<0.01). The corresponding limits of agreement were -27.7–24.8% for sedentary time and -19.0–29.9% for MVPA time.
Figure 1
Bland & Altman plots illustrating agreement between self-reported and accelerometer-measured time sedentary (left figure, N=147) and in moderate-to-vigorous physical activity (MVPA) (right figure, N=149).
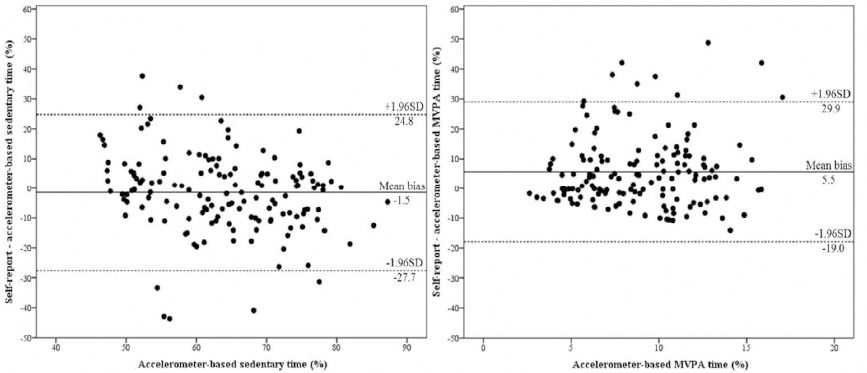
In the multiple regression analyses, none of the interaction terms between sedentary time and potential bias factors were significant, and the statistical model was re-run without interaction terms (table 2). The results showed that age was positively associated with self-report bias in sedentary time (B=0.31, 95% CI 0.09–0.54, P=0.008). Figure 2 illustrates that, on average, younger workers underestimated and older workers overestimated their sedentary time.
Table 2
Multiple regression analysis of bias in self-reported sedentary (N=147) and moderate-to-vigorous physical activity (MVPA) (N=149) time due to age, gender, body mass index (BMI) and low-back pain (LBP) intensity among blue-collar workers. 95% confidence intervals (CI) not including 0 are marked in bold. [acc=accelerometer-based; SED=sedentary.].
Overestimation of MVPA time increased with increasing exposure and was dependent on the level of LBP of the workers. As shown in Figure 2, workers with high LBP (ie, LBP intensity of 6–9) overestimated MVPA time to an increasing extent with increasing exposure. However, workers with mild/no LBP (ie, LBP intensity of ≤5) appeared to have the same size of self-reported bias in MVPA regardless of how much MVPA they actually had.
Figure 2
A: Differential bias by age in self-reported sedentary time, B: differential bias by low back pain (LBP) in self-reported moderate-to-vigorous physical activity (MVPA).
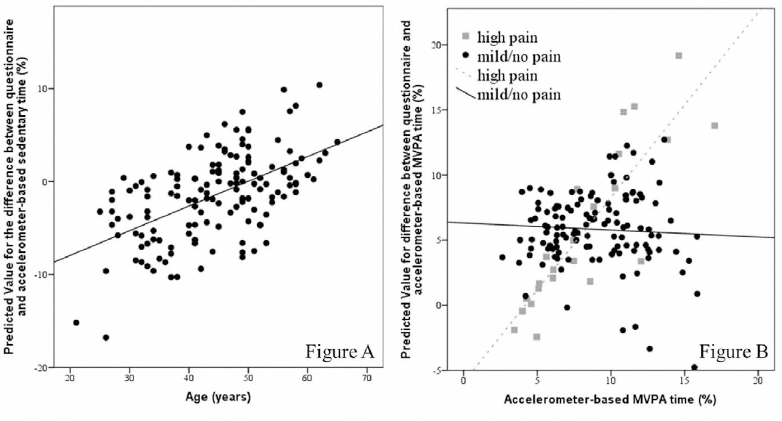
Multicollinearity was considered not to be critical according to the tolerance index (>0.20) and the variance inflation factor (<5) (27). Visual inspection of histograms of standardized residuals and Levene’s test of equality of variances also indicated that assumptions associated with linear regression were not significantly violated.
Discussion
In this study, we investigated the extent to which age, gender, BMI, and LBP introduced differential bias in self-reported time spent sedentary and in MVPA. We found that self-reported sedentary and MVPA time were under- and overestimated, respectively. Differential bias with age was identified for sedentary time; bias in MVPA increased with exposure for workers with high LBP, but was not related to exposure for workers with mild/no LBP.
Bland and Altman plots indicated that, on average, workers underestimated sedentary time by 1.5 time percentage points or ≈22 minutes per day and overestimated MVPA by 5.5 time percentage points or ≈79 minutes per day, referencing the corresponding accelerometer-based measurements. The average difference in sedentary time between the two methods was smaller in our study than reported in previous studies (6–8), and it was not significant. A reason for this discrepancy could be different study populations, and differences in procedures for calculating sedentary time. For instance, previous studies did not express sedentary time in percent of time spent on all activities together within a day. Also, previous studies did not exclude workers with obvious erroneous values, in our case defined as a >50% difference between results from self-reports and accelerometer (6–8). On the other hand, we observed a significant difference of about 80 minutes between the self-reported and accelerometer-based MVPA time, corresponding to 62% of the average accelerometer-based MVPA time. According to global physical activity recommendations for adults, individuals should perform MVPA for at least 75 minutes per week (28). An overestimation as large as 80 minutes per day can thus falsely classify individuals as being physically active. Such an overestimation will lead to an underestimation (attenuation) of the benefits of physical activity to health.
The limits of agreement read from the Bland & Altman plots, when translated into hours, were approximately -7–6 hours/day (ie, 13 hours) for sedentary time, and 5–7 hours/day (ie, 12 hours) for MVPA time. Limits of agreement of this size correspond to those reported in previous studies on general and working populations (7, 29, 30). Overall, our results indicate that self-reports can fairly correctly identify sedentary time at the group level, but not MVPA time. However, they are too uncertain to be used for identifying clinically relevant levels of sedentary time and MVPA at the individual level. Thus, we recommend that self-reports of physical activity and sedentary behavior should be used with due caution in clinical practice, when designing health programs, and in research.
We found that the extent of bias in self-reported MVPA time depended on LBP. Among workers with high LBP, overestimation increased with increasing MVPA whereas workers with mild/no LBP over-reported MVPA to the same extent regardless of their actual MVPA time. For instance, a worker with high LBP who spends 5% of his time on MVPA will, on average, overestimate MVPA by only 2%. Another worker with high LBP spending 15% of his time on MVPA will, on average, overestimate MVPA by 15%. Since, according to our knowledge, our study is the first to investigate the extent to which LBP influences the relationship between actual MVPA time and the extent of self-report bias, our results cannot be compared with previous reports. In order to allow a comparison, we performed analyses without the interaction term between exposure and pain (results shown in appendix B, www.sjweh.fi/show_abstract.php?abstract_id=3693). We observed that LBP did not introduce bias in self-reported MVPA if the interaction between accelerometer-based MVPA and LBP was removed from the model. This is in line with previous research (11, 31) on bias introduced by musculoskeletal pain. Thus, our results illustrate the limitation of statistical models used in previous studies of differential bias. To our knowledge, only two previous studies have, indeed, investigated the issue of interaction between factor(s) influencing self-report bias and the “true” exposure level; these two studies focused on sociodemographic factors (12, 29). We encourage more studies investigating whether the extent of differential bias in self-reported exposures depends on the actual exposure.
We observed that self-report bias in sedentary time was positively associated with age. Age has previously been shown to influence self-report bias in sedentary time in some studies (32, 33) while others did not find an effect (9, 12, 14, 34). However, in one of these studies, the age range was limited to 60–83 years (12). In other studies where age was not found to influence self-report bias, the analysis was based on correlations techniques (12, 34), they did not adjust for actual sedentary time (34), did not use the difference between the two methods as an outcome (14), or they were not conducted on working populations (12, 34).
As illustrated in Figure 2, middle-aged workers about 50 years old were most accurate in reporting sedentary time, while the average young and old worker under- and overestimated sedentary time, respectively. Previous studies have found young individuals to be more accurate than older in reporting sedentary time (7, 35), which contradicts our findings for reasons that are not clear to us. We also found that self-report bias in sedentary time due to age did not change with increasing “true” sedentary time, confirming previous research on elderly (12).
We found that BMI and gender did not influence self-report bias in time spent sedentary and in MVPA. Our results agree with previous studies reporting that gender does not introduce differential bias in self-reported sedentary behavior and physical activity (12, 14, 34). However, previous studies have obtained mixed results on the effect of BMI on self-report bias (5, 7, 9, 10, 14, 35). The reason that we did not find any differential bias due to BMI could be that the prevalence of obese workers was lower in our blue-collar population than in the more mixed populations used in studies reporting BMI to introduce differential self-report bias (10, 15).
A major strength of our study is the use of a valid and reliable method, accelerometry processed by the Acti4 software, for obtaining reference data. Acti4 has shown a good sensitivity and specificity in identifying different postures and physical activity during standardized conditions (21) and during occupational work (24). Another strength of our study is the multiple regression analysis used to identify factors introducing self-report bias, accounting for a possible interaction of the factor with “true” exposure, and adjusting for effects of other variables. Most previous studies investigating differential bias factors have used bivariate analyses, without taking into account the possible confounding effect of other factors. For example, BMI was shown to introduce differential bias in self-reported sedentary time (12), but that study did not adjust for other potentially important bias factors such as gender and socioeconomic factors. A consequence of the multiple regression approach in the present study is, however, that the number of candidate bias factors that could be included in the analysis was restricted, due to the limited size of the study population. Previous studies have shown some psychological factors, such as attitude towards physical activity (36), and some demographical factors, such as education and occupational position (12) to introduce differential bias in self-reported sedentary and MVPA time, but our sample size did not allow analyses of these factors. We also emphasize that, while the present study population represented a variety of blue-collar occupations with a clear dispersion in sedentary behavior (23), it may not be representative of all blue-collar occupations. However, data in the literature on sedentary behavior among blue-collar workers in occupations others than those included in our study are scarce (37–40), and a formal analysis of representativeness is not justified.
As apparent from the present and other studies, straight-forward use of self-reported information of sedentary behavior and physical activity can result in misleading results in both epidemiologic and intervention studies. An attractive option is to correct for self-report bias in a calibration procedure and use the corrected exposure values for further analyses. Calibration, or exposure modeling, is an established method for correcting self-reported information in the field of nutritional and body composition (41, 42) and in occupational hygiene (43–45), and it is becoming increasingly popular in the field of physical activity (46–49), even in an occupational context (50–52). The present study contributes to the development of effective models for estimating “true” sedentary time and time in MVPA by identifying a number of factors that may, in addition to the self-reported exposure in its own right, influence the extent of self-report bias, and that may, therefore, be candidates for inclusion in calibration models.
In conclusion, we found that the average blue-collar worker overestimated time in MVPA, but reported sedentary time reasonably correct. However, self-reports may be grossly biased at the individual level. LBP and age introduced differential bias in self-reported sedentary and MVPA time, while gender and BMI did not. Thus, future studies addressing populations with a high occurrence of LBP and a large dispersion in age should be cautious when using self-reported data on MVPA and sedentary time.