Previous studies have shown that in addition to health, physical and psychosocial working conditions are the most important determinants of sickness absence (1–5). Low socioeconomic position is related to a markedly increased risk of sickness absence, and – according to the level of education, occupational class and income level – there is a 2–4 times higher risk of sickness absence, lowered work ability, and disability pension in the lower compared to higher groups. Moreover sickness absence rates vary between genders with women reporting more sickness absences (2, 3, 5–10).
The economic relevance of sickness absence is notable: in Finland in 2010, the expenditure on sickness benefits was approximately €55 per person per day in long sickness absence periods among persons aged 30–64, amounting to a national annual expenditure of over 0.45% of total GDP of Finland, altogether €800 million (11). Moreover for employers, the indirect costs of sickness absences are approximately €300 per person for each day of absence (12). Long-term sickness absence predicts disability pensions and mortality (13, 14). Understanding the underlying mechanisms leading to high sickness absence rates particularly among persons with a low socioeconomic position is a prerequisite for being able to lengthen work careers significantly in the whole population (7). Only a few population-based studies have examined the causes of socioeconomic differences in sickness absence.
The need to study this topic more deeply is based on earlier research indicating that physical and psychosocial working conditions contribute significantly to inequalities in sickness absence (2, 7, 15, 16). Previous studies explaining the relationship between socioeconomic differences in sickness absence have mainly concentrated either on health-related factors or only on one or two work-related factors, generally psychosocial working conditions. We are aware of few studies that have analyzed the impact of a large variety of determinants on socioeconomic differences in sickness absence (1, 17). However, that study examined only the employees of one employer. Furthermore only a few studies have used nationally representative surveys on population subgroups (2, 18–20).
We examined the educational differences in long-term sickness absence in an 8-year follow-up. Our hypothesis was that educational differences in sickness absence are partly amenable and can largely be explained by working conditions even after adjusting for health, health behavior, and biological risk factors at baseline. Moreover we developed a method to predict adjusted average sickness absence days per working year (DWY). The DWY is an absolute measure calculated as the average of individual expected sickness absence days per working year, which is the product of the expected number of sickness absence episodes per year and their lengths, representing the sample or population average. Therefore it is directly applicable to estimate burden statistics such as economic costs, ie, both direct and indirect costs. The DWY is based on regression models and the benefits of regression modelling such as adjustment for several confounding factors are directly available. Explaining these differences can lead to important improvements, as most of the explanatory factors are easier to adjust than socioeconomic status.
Methods
Study population at baseline
The study was based on the Health 2000 Survey, a comprehensive cross-sectional health interview and examination survey conducted in Finland in 2000–2001. The population-based sample included 5835 Finns aged 30–64 years, of whom 5171 (89%) participated. The survey included an extensive interview (approximately 1.5 hours), several self-administered questionnaires, and a thorough health examination. A detailed description of the sample, contents and implementation of the Health 2000 Survey have been published earlier (21). We focused on participants who had worked at any time during the past 12 months (N=3946). In the analysis, the study population included 1885 men and 1875 women. Mean age was 44.7 years for men and 44.0 years for women.
The Ethics Committee for Epidemiology and Public Health in the Hospital District of Helsinki and Uusimaa, Finland, approved the Health 2000 study. Participation was voluntary and register linkages were approved by the subjects who signed the informed consent form.
Sickness absence follow-up
We linked information on sickness absences from the register of the Social Insurance Institution of Finland (SII) to the survey data. This register covers those sickness absence spells that entitle one to sickness benefits, ie, sickness spells >10-day waiting period. To calculate the risk appropriately, we obtained information on other possible absences from employment during the follow-up from the register of unemployment benefits at the SII, the pension registers at the SII and the Finnish Centre for Pensions, and the Causes-of-Death register at Statistics Finland. The follow-up started from the day when the person participated in the survey and ended if not censored partly or fully by unemployment, pension or death in 31 December 2008. The mean follow-up time was 7.16 (range: 0.003–8.375) years for men and 7.18 (range: 0.002–8.342) years for women.
Education
Information on the level of education was linked from Statistics Finland. We classified education into three categories: (i) “low” consisted of subjects with less than a post-secondary non-tertiary education or a level unspecified or unknown (Level 0–4 of ISCED 1997), (ii) “medium” had a first stage of tertiary education (Level 5–5B); and (iii) “high” had a higher stage of tertiary education or second stage of tertiary education (Level 5A–6).
Health status and work ability
Information on diseases was based on physician clinical diagnoses made during the health examination, applying uniform diagnostic criteria or standardized measurements done by trained nurses, including an electrocardiogram (ECG), spirometry, and blood tests. For cardiovascular diseases and diabetes, the information collected in the health examination was supplemented with register data on hospital discharge diagnoses and entitlement to special reimbursement for medication. Self-rated health (SRH) was assessed with an interview question using a 5-point response scale, dichotomized into good or rather good versus moderate or worse SRH. Mental health status was assessed using the World Health Organization’s composite international diagnostic interview (CIDI) (22). The work ability score, ranging from 0–10, was used as a single-item indicator for self-rated work ability (23, 24). Scores of 0–7 were defined as indicating limited work ability (25).
Health behavior and obesity
The interviewees were asked about their history of smoking and categorized as current, ex-, and never-smokers. Information on physical exercise was based on a self-administered questionnaire. Exercising ≥4/week for ≥30 minutes at a time was defined as adequate. The questionnaire also enquired about sleeping problems during the last 30 days (five categories ranging from no problems at all to frequent problems) and alcohol consumption (measured as grams/week). Heavy drinking was defined as >140 grams/week for women and >280 grams/week for men (26). Body mass index (BMI) was based on measurements of height and weight; values of ≥30 kg/m2 were classified as obesity.
Working conditions
Questions on working conditions at baseline were asked both in the interview and via questionnaire. Physically demanding work was defined as work that included lifting, carrying, bad working postures, working with hands above the shoulder plane, working on knees or squatting, strenuous work with hands or repetitive hand movements, lasting at least half of the workday, all of which were dichotomized for the analysis. Interviewees were also asked about the following chemical and physical hazards at work: noise, dust, shaking or vibration, chemicals, gases, cigarette smoke, coldness, heat, air draught, and insufficient lighting. Response categories were (i) not present or no harm, (ii) troubles to some extent, (iii) troubles quite much, and (iv) troubles a lot. Respondents reporting that ≥1 of these problems troubles them quite a lot were considered to be working in a hazardous environment. Responses were dichotomized accordingly for the analysis.
Indicators of psychosocial working conditions included job control (eight items), job demands (five items), and the combination of low control and high demands, ie, job strain (27, 28). Social support at work (four items) (29) and job insecurity (30) (five items) were also assessed. These questions were based on validated questionnaires. Furthermore, the interviewees were asked about mental violence or bullying and appreciation for their work in the workplace. Work climate (four items) and possibilities to increase job skills with education were also included. These questions have been used in the Finnish Work and Health Study. All the above items were asked in the questionnaire and dichotomized for the analysis.
Statistical analysis
The missing values of the covariates were multiply imputed (31) using the Stata 11 software (StataCorp LP, College Station, TX, USA) and the Markov chain Monte Carlo (MCMC) method. The imputation model contained all covariates. An imputation model that also contained the outcome variables appeared to have virtually no effect on the results. The imputed binary covariate values were transformed back to the original binary scale using the midpoint of the values of the binary covariate as the threshold. Twenty imputed datasets were produced.
The complex sampling design has been described previously (21) and was accounted for. The unit non-response was accounted for using post-stratification weights (31). The survey versions of the Poisson model and the generalized linear model—the latter of which was used for the gamma regression models using the gamma family and log link options procedures of Stata—were used for the regression analyze on the number and the lengths of the sickness absence episodes, respectively. The covariates of the regression models were selected using the Bayesian information criterion (BIC) (33) and the complete-case data. The complex sampling design was ignored in this procedure, since the BIC is based on the likelihood inference.
The margins procedure of Stata was applied to estimate the predicted margins (PM) (34) of the number and the lengths of the sickness absence episodes in the education groups.
In this article, we have calculated the expected average number of sickness absence DWY in the population. The DWY was defined as the (weighted) average of these individual expected lengths, which are absolute measures. For each individual the expected length of sickness absences per working year was defined as the product of the expected number of sickness absence episodes and the expected length of an episode using the predicted values based on the regression models and the individual covariate values as in the predicted margins above. Manipulation of the covariate values allows one to assess the influence of different covariates. The absolute and relative differences are calculated using the DWY estimates based on different manipulations of covariates, eg, highest versus lowest education class. See the appendix (http://www.sjweh.fi/data_repository.php) for the details of the PM and the DWY.
The hypothetical economic calculations regarding the burden of avoidable costs of educational differences to the national economy were made using one-year values for both genders in sickness-absence benefits based on the statistics of the SII which were €59 and €51 per day for men and women, respectively (35). The magnitude of effects was based on the size of the employed 30–64-year-old target population from Statistics Finland (36), the educational structure of the study population from the Health 2000 Survey, and the costs to employers which were valued at roughly €300 per day (12). In the analysis, estimates and modelling addressed in this paper and absolute figures from DWY were used to calculate the avoidable costs. In the economical calculations, we accounted for gender, age, and educational structure.
Results
Table 1 presents the descriptive statistics of the variables.
A clear educational gradient was seen in sickness absence (table 2). Among men in the lowest education category, the average number of sickness absence days was 2.5 times as high as in the highest category; among women the corresponding difference was 2.3-fold. The absolute difference between the education groups among men was 4.86 and 5.97 DWY among men and women, respectively.
Table 2
Age-adjusted sickness absence estimates based on episodes of >9 working days per working year, their standard errors (SE) and differences by gender and education.a
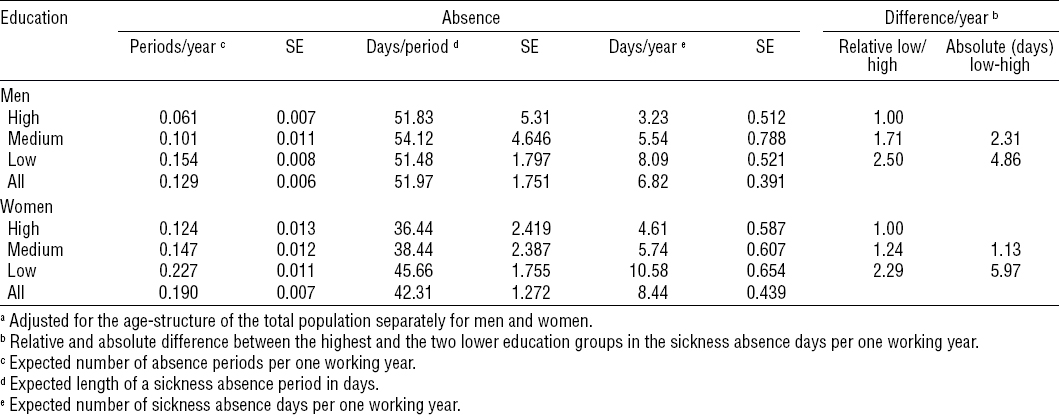
Next we examined the contribution of several health statuses, health-and work-related factors to the association between education and sickness absence.
The average number of sickness absence DWY in the 8-year follow-up remained higher among women than men, and the educational differences remained steep even after the adjustments for working conditions, health status and health behavior (table 3). The variation of the estimates between the different explanatory models in the medium education group was modest (DWY range for men: 5.46–5.17 and women: 5.48–5.07), thus these results are not presented in table 3. Moreover gender differences in overall DWY among men and women was notable (men 6.8 DWY, women 8.4 DWY), and remained similar through the analysis.
Table 3
The average number of sickness absence days based on episodes of >9 working days per working year among employed men. Modelling variables as if the risk would be the same as in the highest education group.a Comparison of the highest and the lowest educational groups. [M1=model one; ref=reference; SE=standard error.]
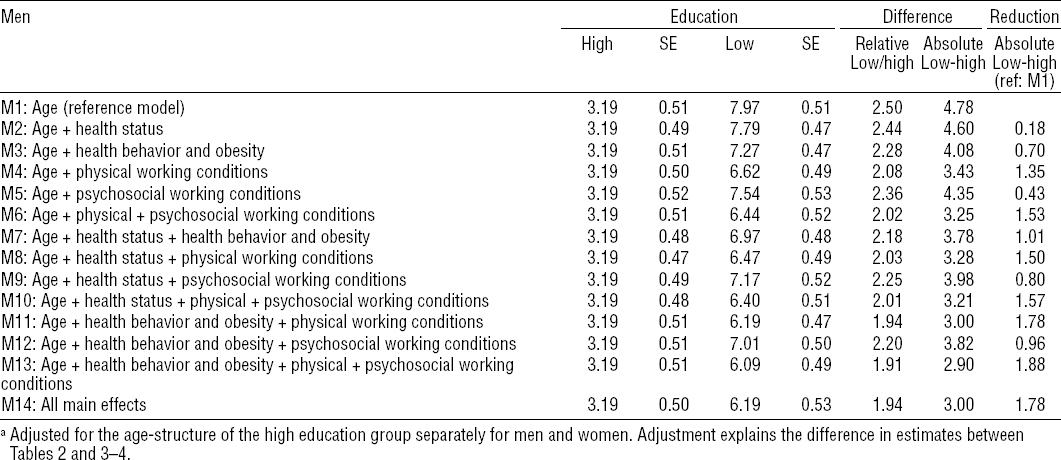
Adjusting for self-rated and diagnosed health status at baseline (model 2) attenuated the difference in sickness absence only by 0.18 DWY among men but by 0.56 DWY among women. Within the health status and work ability domain, the most important explanatory factors were self-rated work ability among men and self-rated health among women. Adjusting for health behavior and obesity (model 3) attenuated the differences by 0.70 DWY among men and 0.94 DWY among women, mostly attributable to smoking.
Adjusting for physical working conditions reduced the educational differences in sickness absence by 1.35 DWY among men and 1.24 DWY among women (model 4), whereas adjusting for psychosocial working conditions (model 5) only slightly attenuated the difference (0.43 and 0.08 among men and women, respectively), mainly attributable to job control. Adjusting for the job demands only, we found negligible effect among men (0.024 DWY) and women (increase of 0.1 DWY). Adjusting for all working conditions (model 6) reduced the difference by 1.53 and 1.08 DWY among men and women, respectively.
Simultaneous adjustment for health status, health behavior, and obesity (model 7) decreased the educational differences in sickness absence by 1.01 and 1.82 DWY among men and women, respectively. Adjusting for health status and physical working conditions attenuated the difference among men by 1.50 DWY and 1.89 DWY among women (model 8). Adjusting for health status and psychosocial working conditions (model 9) reduced the difference by only 0.80 DWY among men and 0.81 DWY among women. Adjusting for health status and all working conditions (model 10) attenuated the difference by 1.57 DWY for men and women.
Adjusting for health behavior and physical working conditions (model 11) reduced the difference by 1.78 and 2.00 DWY among men and women, respectively whereas adjusting for health behavior, obesity, and psychosocial working conditions (model 12) only attenuated 0.96 DWY among men and 1.27 DWY among women. Among men, the largest reduction (1.88 DWY) in the educational differences in sickness absence was achieved by adjusting simultaneously for health behavior, obesity, and all working conditions (model 13). Among women, the largest reduction (2.57 DWY) in the difference was achieved by adjusting simultaneously for health status, health behavior, and all working conditions.
Among both men and women, physical working conditions had an important effect when explaining the educational gradient in sickness absence. Yet, no single item came up among the various aspects of physical conditions included in the analysis. Psychosocial working conditions had a smaller effect, mainly contributed to by low job control and the monotony of work. Furthermore, among men, the explanatory power of health behavior was mostly due to smoking while the effect of health status was mainly caused by self-rated health among women. The largest single decline in educational differences in sickness absence was achieved by adjusting simultaneously for physical workload, health behavior (especially smoking), and ill-health (data not shown).
Using estimates from this study, if the two lowest educational groups reached the level of health status and health- and work-related factors prevailing among the highest educational group, approximately €450 million, some 0.3% of the Finnish GDP would be saved each year.
Discussion
This study had five key results: (i) Clear educational differences in sickness absence were found among men and women. The burden of sickness absence was higher among women (6 days/year) than men (5 days/year) but the relative educational difference was slightly steeper among men than women. (ii) Physical working conditions contributed most to the educational differences in sickness absence. (iii) The largest decline in educational differences in sickness absence among men was achieved by adjusting simultaneously for health behavior, obesity, and all working conditions, and, among women, by adjusting simultaneously for health, health behavior, and all working conditions. (iv) The importance of explanatory factors varied between genders. (v) A substantial part of the educational difference remained unexplained (37% among men and 45% among women were explained). Our hypothesis was partly confirmed as physical working conditions appeared to make a large contribution to the differences in sickness absence between levels of education. However, psychosocial working conditions only had a smaller effect, mainly resulting from low job control and the monotony of work.
The results on clear socioeconomic differences in sickness absence as well as the higher absence level among women are in line with several earlier studies (5). Moreover, physical working conditions made a significant contribution to the educational differences as in previous studies (1, 16, 18). In this study, adjusting for physical working conditions attenuated the difference between education groups by approximately one quarter, whereas the corresponding contribution of psychosocial working conditions was <10% among men and negligible for women. Among men, adjusting for working conditions reduced the difference between education groups in sickness absence more than the adjustment for health and health behavior. In some earlier studies the role of psychosocial working conditions has been larger, but in others it has been similar to our study (7, 16, 18). The result suggests an association with physical working conditions and is in line with earlier albeit smaller and non-representative studies where physical but not psychosocial working conditions were associated with sickness absence (19, 20).
Health behavior and obesity contributed markedly to the difference between educational groups as shown also elsewhere (7). Simultaneous adjustment for health behavior and physical working conditions played the most important role in explaining educational differences in sickness absence. Earlier studies have reported only a small effect of health behavior and obesity (2, 7) and smoking and alcohol consumption (4). In this study, the effect increased substantially when adjusting for all of confounders simultaneously. However earlier studies were based on a representative sample of the Danish population (2) and employees of a French electricity company (16) and may therefore have different socially patterned exposures. Moreover using mainly psychosocial working conditions, job demands for instance, interpretations could lead us to increase educational differences.
The importance of many explanatory factors varied between genders. For example, health and health behavior together had a strong effect among women (DWY reduction 1.82) and a smaller one among men (DWY reduction 1.01). Among women, the contribution of health and health behavior to the educational differences in sickness absence was even greater than that of working conditions. Among women, health status also showed a higher association than for men, whereas the prevalence of most of the health-related variables used were higher among men. Health problems might cluster to lower educated women. This result is in line with earlier studies only regarding women (4). However including the psychosocial factors leads to a smaller reduction than using physical factors alone and might reveal the mixed explanatory power of psychosocial factors. As shown in table 1, high job demands are more common among women (35%) than men (21%) and this variety of mixed results are in the line with earlier studies using self-rated health as outcome (37). A more detailed analysis could provide answers to the somewhat interesting gender differences.
Although we were able to examine a large variety of health- and work-related factors, only one fifth to one fourth of the educational differences in the sickness absence days was explained. Our burden estimates showed that 2.90 and 3.12 days among men and women, respectively, could not be explained.
Methodological considerations
Persons with poor health and limited work ability who exit the workforce can result in a “healthy worker” effect. Furthermore, limited work ability may lead the person to seek a job that he or she can manage even with limited work ability (38). Therefore the results of this study might show conservative estimates for the population. Short sickness absences are prior to self-valuation, however they do predict (especially if frequent) longer episodes. Moreover socioeconomic differences in short-term sickness absences have also been reported (39). However, this study has several strengths. The sample had a high response rate (89%) and had a register-based follow-up for eight years that was representative of the working-aged Finnish population and the results can be generalized to the whole active workforce aged 30–64 years. The study included valid measurements of health-related factors, work ability, and working conditions. Many of the explanatory factors were based on health examinations or registers. Further studies should also allow for the main diagnosis categories causing sickness absence.
Table 4
The average number of sickness absence days based on episodes >9 working days per working year among employed women. Modelling variables as if the risk would be the same as in the highest education group. a Comparison of the highest and the lowest educational groups. [M1=model one; ref=reference; SE=standard error.]
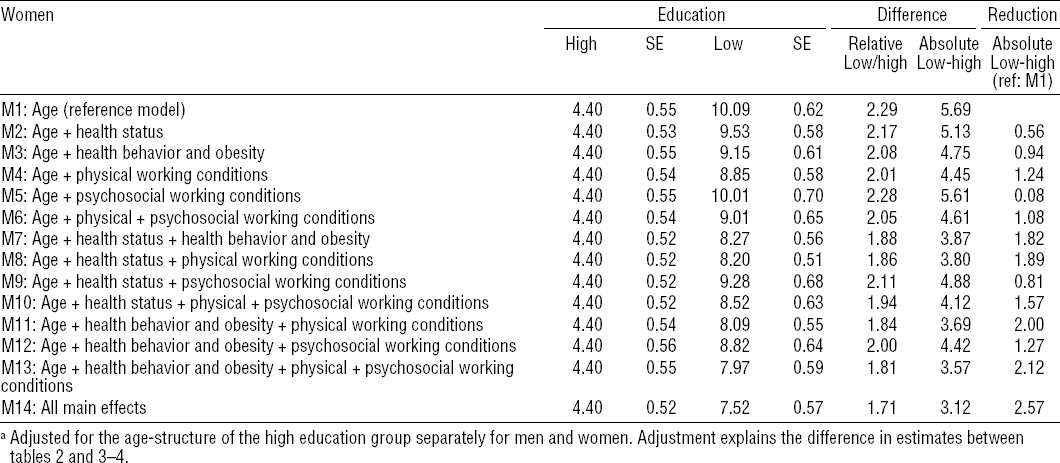
Standard regression analysis methods for non-negative outcomes provide proportional differences on individual level thus they are not directly applicable to estimate the burden on a population level. The DWY, which is an absolute measure, allows for a deeper understanding of the relative importance of different work- and health-related factors on socioeconomic differences in sickness absence. Adjusting for several factors related to both sickness absence and education simultaneously is difficult if not impossible using traditional methods such as direct standardization, as this method would require large amounts of data. Regression models, which the DWY was based on, can adjust for a relatively large number of factors.
The predictive margins of the number of sickness absence episodes and their lengths also provided insight into how to assess the origin and size of the differences between the educational groups and genders. The burden due to sickness absence depends not only on the number of episodes but also on their lengths. Some factors can be associated with only one of these components and others with both of them. In the latter case, the associations can amplify the burden or, if the associations with the components are positive and negative, even cancel each other out. In all of these cases, the researcher should analyze both components and then assess their joint effect on the burden using, for example, our novel method in order to obtain more detailed insight. Traditional regression analyze, which usually provide only estimates of proportional differences—such as risk ratios in case of the Poisson regression and proportional difference in the expected length of an episode in the case of the gamma regression—may be less useful if more concrete measures, such as absolute differences in sickness absence days, are sought.
The contribution of several health- and work-related factors to educational differences in sickness absence has not been simultaneously investigated earlier in a representative population-based study. In addition, a new method to calculate the average number of sickness absence DWY was introduced in this study. DWY enabled us to calculate the economic burden of long term sickness leave with rough estimates. However these calculations cover only roughly the direct and indirect hypothetical costs of sickness absence. Severity of remaining illness, disabilities, and their variance between individuals may vary.
Concluding remarks
Sickness absence is an important measure of vitality of the society and burden to national economy. This study also has implication to practice, improving working conditions, especially physical working conditions, and reducing smoking may reduce sickness absence particularly in the lower educational groups. Adjusting these factors is more plausible than increasing the educational level or other proxies of socioeconomic status in the whole population. The economic relevance of our findings is notable (€450 million per year, 0.3% GPD) and the method described for estimating the days of sickness absence can be applied to calculate economic burden in other countries as well.