High blood pressure levels are the main risk factor for the global burden of disease and were responsible for some 10.4 million deaths worldwide in 2013 (1). There is evidence that, from 115 mmHg systolic blood pressure (SBP) and 75 mmHg diastolic blood pressure (DBP), cardiovascular risk increases steadily and linearly (2). The relation between blood pressure and cardiovascular outcomes is thus continuous and direct, but the lower boundary, below which no gain results from reducing blood pressure levels, is still uncertain (3).
Although blood pressure is a continuously distributed cardiovascular risk factor, studies of its determinants have focused on the central portion or the extremes of the distribution, using conventional regression methods that estimate effects for the population mean or that model high blood pressure as a dependent variable (4–6). These analytical strategies are very useful, but they do not permit an understanding of how such determinants affect the whole blood pressure distribution. Accordingly, important aspects of the association studied can remain hidden. Quantile regression is an alternative technique by which the effects of exposure can be estimated in different quantiles of the outcome distribution (7), but it is rarely used in epidemiology (8). One study using this method to examine some of the factors most related to blood pressure, including age, body mass index and physical activity, revealed the existence of heterogeneous patterns of association across the blood pressure distribution (9). In that light, efforts are needed to improve the understanding of how other important determinants act on the blood pressure continuum.
Of the various factors that determine increased blood pressure levels, major emphasis has been placed on the role of psychosocial variables, including workplace stressors (10). Job strain has become an important field of research in recent decades and a number of theoretical models have been proposed for evaluating its health effects. The most widely known of these, the demand–control model (DCM) developed by Karasek, defines job strain as the product of a combination of high demands and low control over the work process (11). Job demands relate to the load and pace of activities and the difficulty of performing them, as well as the presence of contradictory requirements. Job control comprises two subscales: skill discretion and decision authority, the former relating to opportunities to be creative, use intellectual competences and lean new things; the latter, to the authority to take decisions on how to work (12).
As there is no consensus on the best approach to evaluating DCM, job strain has been operationalized in different ways (13, 14). The strategy most commonly adopted is a classification by quadrants (15), where the dimensions are dichotomized at their medians and job strain is defined as the combination between high job demands and low job control (11). Other procedures often used include independent evaluation of the dimensions and using ratios (16) or subtractions (14) between levels of job demands and job control. One aspect common to all these approaches has drawn criticism: the analyses merge the two subscales of the job control dimension, disregarding the fact that they represent theoretically distinct constructs (17). There is evidence that skill discretion and decision authority can exert opposing effects on different health outcomes (18–21). Also, psychometric analyses of the main instruments used to gauge DCM have shown that these subscales do in fact form two distinct dimensions (17, 22–24).
Despite the positive evidence of an association between job strain and blood pressure (25–27), a growing number of studies have also produced inconclusive results (28–31). The possible explanations proposed for these divergences relate mostly to methodological issues, including: study design (cross-sectional versus longitudinal studies), study population (general population versus specific occupation), method of measuring blood pressure (casual versus ambulatory), the instrument used to measure the DCM, how the exposure and outcome measurements are operationalized, and adjustment for potential confounders (26, 27, 32–34). Also, from the foregoing, two potential sources of inconsistencies as yet little explored in prior studies have been identified: analysis of the job control dimension subscales as a single construct and the use of analytical strategies that yield evidence on specific portions of the blood pressure distribution.
In addition to methodological considerations, the divergences among the studies can be ascribed partly to the fact that the effects of job strain are not homogeneous in the population. In that regard, various factors have been noted to act on moderate individual susceptibility to the harmful effects of job strain (35, 36). Prominent among these are gender-related factors, as less consistent results for the association between job strain and blood pressure have been observed among women (26, 33). Another important, and as yet little explored, factor is use of antihypertensives. Although practically all studies of the relation between job strain and blood pressure consider such use a confounder (37, 38) or exclude medicated hypertensives from their analyses (39, 40), it has been demonstrated that the relations between a number of factors and blood pressure vary between users and non-users of antihypertensives (9, 34, 41). Accordingly, it is suggested that antihypertensives are potentially effect modifiers of the association studied here (37, 38).
Given these observations, the aims of this study were to: (i) investigate whether the individual DCM components, especially skill discretion and decision authority, are associated differently with blood pressure; and (ii) using quantile regression, examine whether these associations vary over the blood pressure distribution. We also tested for the presence of interaction with gender and use of antihypertensive drugs.
Methods
Study population
The Brazilian Longitudinal Study of Adult Health (ELSA-Brasil) is a multicenter cohort study whose baseline included 15 105 current and retired civil servants of both genders, 35–74 years old, from higher education and research institutions in six of Brazil’s state capitals (the federal universities of Minas Gerais, Espírito Santo, Bahia and Rio Grande do Sul, the University of São Paulo and the Oswaldo Cruz Foundation). Volunteers were recruited through communication strategies (on-site and radio announcements, mailings, outdoor billboards and telephone calls) and active recruitment (from a randomly ordered list of employees). Efforts were made to meet recruitment goals by gender, age, and occupational category so as to ensure a wide demographic and socioeconomic gradient across the study population. Detailed descriptions of the study design and recruitment methods can be found in other publications (42–44). The research ethics committees of each of the participating institutions and the national research ethics committee (CONEP – No. 13065) approved the ELSA-Brasil research protocol. Informed consent was obtained from all study participants.
This study used ELSA-Brasil baseline data (2008–2010), which were collected by interview and clinical and laboratory examinations conducted to rigorous standards of quality assurance and control (45). The analyses in this article excluded the retired participants (N=3009) and those with missing data on the study variables (N=449), resulting in a study sample of 11 647 participants.
Variables
Blood pressure and use of antihypertensive drugs
Blood pressure was measured during the morning in a quiet room at a controlled temperature (20–24 °C). With the participant in a seated position, with an empty bladder and after resting for five minutes, three measurements were taken on the left arm at one-minute intervals, using a validated oscillometric blood pressure monitor (Omron HEM 705CPINT). Blood pressure was considered to be the mean of the last two measurements (46).
Participants were asked about any continuous drug use in the two weeks prior to the baseline examination and antihypertensive drugs were characterized according to their pharmacological classification. In this study, users of antihypertensives were considered to be those who, in addition to reporting using an antihypertensive drug, answered affirmatively to the question: “Was any of the drugs you have taken during the past two weeks for hypertension (high blood pressure)?”.
DCM components
The Brazilian version (47) of the Swedish Demand–Control–Support Questionnaire (DCSQ) (16) was used to measure levels of job demands (5 items), skill discretion (4 items) and decision authority (2 items). Each item comprised four response categories, ranging from “often” to “never or hardly ever”. The Brazilian version of the DCSQ demonstrated satisfactory reproducibility and internal consistency (47). In keeping with the findings of studies that have evaluated the dimensional structure of this instrument (23, 24), the item on repetitive work was removed from the skill discretion scale. In constructing the indicators for each DCM component, the scores produced by adding together the responses to the items on job demands (5–20 points), skill discretion (3–12 points) and decision authority (2–8 points) were dichotomized into high and low at the median for these dimensions (14, 11, and 6 points, respectively).
Potential confounding factors
Potential confounders of the association between job strain and blood pressure have been identified in the literature. As a guide in selecting covariables for inclusion in the analyses, a directed acyclic graph (DAG) was constructed using the DAGitty programme (48). This approach has been used in epidemiology to represent causal relations among variables and assists in identifying a minimum set of covariables that is sufficient to control for the confounder situation (49).
On the basis of this strategy, the following covariables were considered in this study: gender (male and female); age (continuous, in years); race/color, self-reported by the classification used in Brazil’s population census (black, brown, white, Asian, or indigenous); schooling, as a marker of present socioeconomic position (up to lower secondary complete, upper secondary complete, undergraduate incomplete, undergraduate complete and postgraduate); mother’s schooling, as a marker of early socioeconomic position (less than lower secondary complete, lower secondary complete, upper secondary complete and undergraduate complete); weekly hours worked (continuous, in hours) and recruitment site (Bahia, Espírito Santo, Minas Gerais, Rio de Janeiro, Rio Grande do Sul or São Paulo). Unhealthy lifestyle-related variables (poor diet, sedentarism and alcohol and tobacco use), obesity and type-2 diabetes were not considered confounders, because they have been indicated to be mediators of the association studied here (33, 50). In the same way, family history of cardiovascular diseases was not considered a confounder, because there is no evidence to support an association with job strain.
Statistical analyses
Tests of means (T-test and ANOVA for covariables with two or more categories, respectively) and proportions (chi-square) were used to compare blood pressure levels and DCM component frequencies by covariable.
The associations between the exposure variables (job demands, skill discretion and decision authority) and outcomes (SBP and DBP) were first tested using gamma regression models. These models belong to the class of generalized linear models and are appropriate for strictly positive, asymmetrical, continuous outcomes, as is the case with blood pressure (51). Although the ordinary least squares (OLS) method is widely used to model blood pressure as a continuous variable, prior studies have indicated a better fit from the gamma model using a dependent variable with similar distributional characteristics (52, 53). In the gamma models, the identity link function was chosen because it permits direct interpretation of the estimated parameters (51). Thus, our results can be directly compared with those of previous studies on the topic that have used the OLS method.
From the set of covariables mentioned above, four models were constructed for each DCM component, considering SBP and DBP separately: (i) crude model; (ii) model adjusted for gender, age and recruitment site; (iii) model 2 with additional adjustment for race/color and markers of socioeconomic position; and (iv) model 3 with additional adjustment for weekly hours worked. An interaction term was included in the final models (model 4) in order to test for the presence of interaction between each DCM component and the variables “gender” and “use of antihypertensives”.
In order to test for heterogeneity in the association between exposure and outcome over the blood pressure distribution, quantile regression models were also fitted (7). The quantile models were constructed for each DCM component, considering SBP and DBP separately. Coefficients were estimated at regular 5-quantile intervals, from the 5th to the 95th quantile and were adjusted for the covariables present in the final gamma model (model 4).
In all the analyses, the coefficients of the gamma and quantile models represent the difference in blood pressure, in mmHg, for the categories “high job demands”, “high skill discretion” and “high decision authority” in comparison with the reference categories (respectively, “low job demands”, “low skill discretion” and “low decision authority”). All analyses were performed using R 3.1.2 (54). The quantreg library (55) was applied to fit the quantile regression models, while the graphs were drawn using ggplot2 (56).
Results
Participant characteristics
In the overall study population, mean SBP was 119.5 mmHg (1st quartile [Q1]=108; median [Q2]=117.5; 3rd quartile [Q3]=128.5) and mean DBP was 76.3 mmHg (Q1=68.5; Q2=75.5; Q3=83) (see supplementary file 1 for sample quantiles of the blood pressure distribution, www.sjweh.fi/show_abstract.php?abstract_id=3755). Of total participants, 50.4% (N=5871) were classified as under high job demands, 62.1% (N=7237) as high skill discretion and 60.1% (N=6998) as enjoying high decision authority. Table 1 shows the descriptive characteristics of the study population, which comprised 5523 men and 6124 women, including mostly participants who were 45–54 years old (47.8%), white (51.5%), postgraduates (37.1%), whose mothers were educated to less than complete lower secondary level (55.7%) and who worked a 40-hour week (49.4%).
Table 1
Characterization of participants by blood pressure levels and demand–control model components. ELSA-Brasil, 2008–2010 (N=11647) [DBP=diastolic blood pressure; SBP=systolic blood pressure].
Variables | N | % | Mean (mmHg) | Frequency (%) | |||
---|---|---|---|---|---|---|---|
|
|
||||||
SBP | DBP | High job demands | High skill discretion | High decision authority | |||
Gender | |||||||
Male | 5523 | 47.4 | 124.3 b | 79.1 b | 46.1 b | 63.6 b | 62.6 b |
Female | 6124 | 52.6 | 115.3 | 73.8 | 54.3 | 60.8 | 57.8 |
Age (years) a | |||||||
35–44 | 3265 | 28.0 | 114.6 b | 74.2 b | 53.1 b | 63.8 b | 58.1 b |
45–54 | 5568 | 47.8 | 119.7 | 76.9 | 51.0 | 60.3 | 59.2 |
55–64 | 2580 | 22.2 | 124.5 | 77.6 | 47.0 | 63.7 | 63.8 |
65–74ß | 234 | 2.0 | 131.3 | 77.7 | 35.9 | 66.7 | 67.9 |
Race/color | |||||||
Black | 1879 | 16.1 | 124.6 b | 79.1 b | 48.8 c | 52.7 b | 51.9 b |
Brown | 3374 | 29.0 | 121.3 | 77.4 | 49.9 | 56.5 | 53.5 |
White | 5993 | 51.5 | 117.0 | 74.9 | 51.2 | 68.3 | 66.1 |
Asian | 283 | 2.4 | 117.1 | 73.9 | 54.8 | 69.3 | 71.4 |
Indigenous | 118 | 1.0 | 124.6 | 77.2 | 41.5 | 42.4 | 45.8 |
Schooling | |||||||
Up to lower secondary complete | 1199 | 10.3 | 128.4 b | 80.1 b | 38.5 b | 34.6 b | 43.8 b |
Upper secondary complete | 3339 | 28.7 | 121.9 | 77.5 | 46.5 | 46.2 | 46.1 |
Undergraduate incomplete | 889 | 7.6 | 119.4 | 77.0 | 51.3 | 54.1 | 53.3 |
Undergraduate complete | 1896 | 16.3 | 117.6 | 75.4 | 49.1 | 59.3 | 57.5 |
Postgraduate | 4324 | 37.1 | 116.2 | 74.5 | 57.1 | 84.9 | 77.9 |
Mother’s schooling | |||||||
Less than lower secondary complete | 6483 | 55.7 | 121.0 b | 77.0 b | 47.5 b | 54.4 b | 53.2 b |
Lower secondary complete | 2280 | 19.6 | 119.0 | 75.9 | 52.5 | 65.3 | 62.9 |
Upper secondary complete | 2051 | 17.6 | 117.4 | 75.5 | 54.3 | 74.3 | 71.8 |
Undergraduate complete | 833 | 7.2 | 114.8 | 73.6 | 57.7 | 83.8 | 77.1 |
Working week (hours) a | |||||||
<40 | 2042 | 7.2 | 121.5 b | 76.7 b | 43.7 b | 50.0 b | 49.2 b |
40 | 5759 | 49.4 | 119.9 | 76.6 | 43.0 | 56.4 | 58.9 |
>40 | 3846 | 33.0 | 118.0 | 75.7 | 65.1 | 77.2 | 67.6 |
Recruitment site | |||||||
Bahia | 1453 | 12.5 | 124.7 b | 78.9 b | 47.2 b | 58.9 b | 57.9 b |
Espírito Santo | 810 | 7.0 | 117.9 | 74.3 | 53.8 | 57.9 | 59.3 |
Minas Gerais | 2389 | 20.5 | 119.4 | 77.1 | 54.9 | 64.7 | 58.4 |
Rio de Janeiro | 1589 | 13.6 | 118.6 | 76.9 | 48.6 | 72.7 | 65.3 |
Rio Grande do Sul | 1445 | 12.4 | 119.2 | 75.6 | 49.0 | 59.8 | 59.7 |
São Paulo | 3961 | 34.0 | 118.5 | 75.2 | 49.4 | 59.3 | 60.1 |
Higher SBP and DBP levels were observed in men, those who were older, who declared themselves to be black or indigenous, had less schooling, had mothers with less schooling and who worked a shorter week. As regards the DCM components, the highest frequency of high job demands was observed among women and the younger participants, while higher levels of skill discretion and decision authority were observed among men and the older participants. Participants of white and Asian race/color, those with more schooling, those whose mothers had more schooling and those with longer working weeks reported higher levels of the three components examined. The “recruitment site” variable returned significantly higher blood pressure levels among participants from Bahia state. A higher frequency of high job demands was observed in the states of Espírito Santo and Minas Gerais, while high skill discretion and high decision authority were more frequent in Rio de Janeiro (table 1).
Association between the DCM components and blood pressure in the gamma models
In the overall study population, job demands, skill discretion and decision authority were inversely associated with SBP and DBP in the unadjusted gamma regression models. After adjusting for confounders, however, no significant association was observed. The coefficients estimated were similar by gender (P for interaction test >0.05), but use of antihypertensives and decision authority were observed to interact significantly in relation to SBP (table 2).
Table 2
Coefficients (95% CI) for the association between the demand–control model components and blood pressure in the gamma regression models for the total study population. ELSA-Brasil, 2008-2010 (N=11 647) [CI=confidence interval; Coef=coefficient; DBP=diastolic blood pressure; SBP=systolic blood pressure].
Models | SBP (mmHg) | DBP (mmHg) | ||||||||||
---|---|---|---|---|---|---|---|---|---|---|---|---|
|
|
|||||||||||
High job demands e | High skill discretion f | High decision authority g | High job demands e | High skill discretion f | High decision authority g | |||||||
|
|
|
|
|
|
|||||||
Coef. | 95% CI | Coef. | 95% CI | Coef. | 95% CI | Coef. | 95% CI | Coef. | 95% CI | Coef. | 95% CI | |
Model 1 a | -2.06 | -2.66– -1.47 | -2.00 | -2.62– -1.39 | -1.02 | -1.63– -0.42 | -1.15 | -1.55– -0.76 | -0.93 | -1.34– -0.53 | -0.71 | -1.11– -0.31 |
Model 2 b | -0.88 | -1.43– -0.34 | -2.07 | -2.64– -1.51 | -1.69 | -2.25– -1.14 | -0.60 | -0.98– -0.22 | -1.17 | -1.55– -0.78 | -1.06 | -1.44– -0.68 |
Model 3 c | -0.26 | -0.79–0.27 | 0.37 | -0.22–0.96 | 0.25 | -0.31–0.82 | -0.24 | -0.61–0.13 | 0.22 | -0.19–0.63 | 0.03 | -0.36–0.43 |
Model 4 d | -0.11 | -0.65–0.43 | 0.47 | -0.12–1.07 | 0.27 | -0.29–0.84 | -0.20 | -0.57–0.18 | 0.26 | -0.15–0.67 | 0.04 | -0.35–0.43 |
P for interaction with gender | 0.14 | 0.74 | 0.72 | 0.12 | 0.48 | 0.56 | ||||||
P forinteraction with use of antihypertensives | 0.36 | 0.31 | 0.02 | 0.66 | 0.84 | 0.69 |
In analyses stratified by use of antihypertensives (table 3), a borderline positive association was observed between decision authority and SBP only among participants who were non-users of antihypertensives, where SBP increased by 0.59 mmHg (95% CI 0.00–1.18) among those with high decision authority. Nor was interaction with gender significant in the models stratified by use of antihypertensives.
Table 3
Coefficients (95% CI) for the association between decision authority and blood pressure in the gamma regression models, by use of antihypertensives. ELSA-Brasil, 2008-2010 [CI=confidence interval; Coef=coefficient; DBP=diastolic blood pressure; SBP=systolic blood pressure].
Models | SBP (mmHg) | DBP (mmHg) | ||
---|---|---|---|---|
|
|
|||
High decision authority e | High decision authority e | |||
|
|
|||
Coef. | 95% CI | Coef. | 95% CI | |
Non-users of antihypertensives (N=9057) | ||||
Model 1 a | -0.30 | -0.94–0.34 | -0.44 | -0.88–0.00 |
Model 2 b | -1.08 | -1.66– -0.50 | -0.86 | -1.28– -0.45 |
Model 3 c | 0.57 | -0.02–1.17 | 0.10 | -0.32–0.53 |
Model 4 d | 0.59 | 0.00–1.18 | 0.11 | -0.32–0.53 |
P for interaction with gender | 0.78 | 0.90 | ||
Users of antihypertensives (N=2590) | ||||
Model 1 a | -1.99 | -3.37– -0.61 | -0.76 | -1.61–0.09 |
Model 2 b | -2.34 | -3.68– -1.00 | -0.65 | -1.48–0.18 |
Model 3 c | -0.24 | -1.60–1.12 | 0.26 | -0.60–1.12 |
Model 4 d | -0.19 | -1.55–1.17 | 0.28 | -0.58–1.14 |
P for interaction with gender | 0.91 | 0.35 |
Association between DCM components and blood pressure in the quantile models
Total study population results from the quantile models are shown in figure 1. No association was observed with job demands or decision authority anywhere in the distribution of either of the outcomes examined. In relation to the skill discretion component, a positive association was found only with SBP at the lower end of the distribution, with a coefficient of 1.01 mmHg (95% CI 0.31–1.71) in quantile 10.
Figure 1
Coefficients (95% CI) for the association between demand–control model components and blood pressure in the quantile regression models for the total study population. ELSA-Brasil, 2008-2010. The solid lines and adjacent shaded areas represent, respectively, the estimates and their 95% confidence interval (CI). The broken lines indicate the null value. The coefficients are adjusted by gender, age, recruitment site, race/color, schooling, mother’s schooling and weekly hours worked. [DBP=diastolic blood pressure; SBP=systolic blood pressure.]
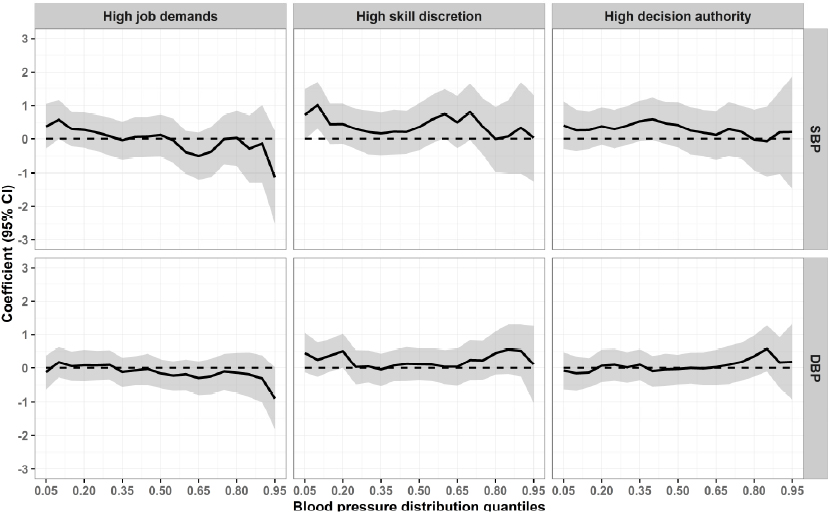
Figure 2 shows the results from the quantile models for the association between decision authority and blood pressure, stratified by use of antihypertensives. Among non-users of antihypertensives, a positive association was observed in the central portion of the SBP distribution. The coefficients were significant from quantiles 35–60, the highest value being found at quantile 55, where high decision authority was associated with an increase of 0.94 mmHg (95% CI 0.22–1.66) in SBP. Among users of antihypertensives, in turn, a direct association was observed only at the lower end of the DBP distribution, where the coefficients were 1.85 mmHg (95% CI 0.48–3.22) and 1.32 mmHg (95% CI 0.28–2.36) in quantiles 5 and 10, respectively.
Figure 2
Coefficients (95% CI) for the association between decision authority and blood pressure in the quantile regression models, by use of antihypertensives. ELSA-Brasil, 2008-2010. The solid lines and adjacent shaded areas represent, respectively, the estimates and their 95% confidence intervals (CI). The broken lines indicate the null value. The coefficients are adjusted by gender, age, recruitment site, race/color, schooling, mother’s schooling and weekly hours worked. Reference category: low skill discretion. [DBP=diastolic blood pressure; SBP=systolic blood pressure.]
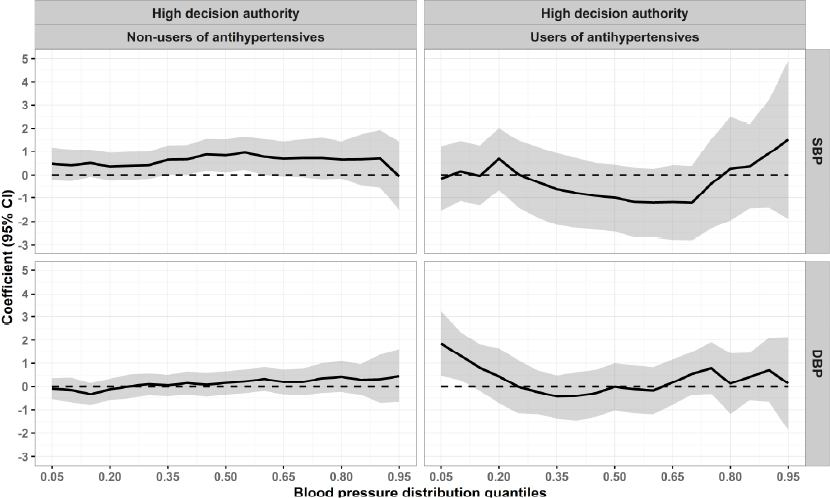
For details on quantile regression coefficients and 95% CI, see supplementary files 2 and 3, www.sjweh.fi/show_abstract.php?abstract_id=3755.
Discussion
Our main results indicated that high decision authority associates positively with SBP and that this association occurs only in the central portion of the blood pressure distribution and among non-users of antihypertensives. No consistent associations were observed for decision authority and DBP or for skill discretion and SBP/DBP. Moreover, no significant association was observed for job demands. This is the first study to investigate the association between job strain and blood pressure using quantile regression analysis. To date, we have been unable to identify any publication that has compared the two components of the job control dimension in studying this relationship.
Independently of the analytical strategy applied, our analyses detected no association between job demands and blood pressure. Although the DCM originally proposed that excessive job demands act adversely on cardiovascular health (57), other authors have also failed to identify significant associations between this dimension and blood pressure levels (28, 58) and with others cardiovascular risk factors (21, 59). It has been suggested that these inconsistencies can be explained, at least in part, by non-differential information bias, given that the concept of job demands measured by the DCM does not properly capture emotional demands. Thus, the actual level of job demands that workers are exposed to is being underestimated, which consequently leads to underestimation of the true association between this dimension and blood pressure (33).
High decision authority at work, on the other hand, was found to associate with higher blood pressure, contrary to the effects proposed by the DCM (57). In agreement with the results of this study, Joensuu et al (20), in a cohort of 13 510 employees of a Finnish company, identified greater overall mortality and specific mortality by cardiovascular outcomes and causes related to alcohol consumption among those with higher levels of decision authority. Previous results in the same cohort indicated that greater decision authority was associated with increased risk of hospital admission for mental disorders, including those relating to alcohol use and depression (19). Similarly, other authors have found that decision authority is positively associated with overall and central obesity as measured, respectively, by body mass index and waist circumference (21).
In recent years, changes in how work is organized – especially as regards increasingly long workhours, the prevalence of occupations requiring intense computer use and higher qualifications and the declining number of manual workers – point to a need to reconsider certain aspects of the DCM (60). In this new context, the health benefits of increasing decision authority may not be as evident as in the historical context where the DCM was developed (11), which was characteristically one of industrialization and repetitive tasks (61).
In the contemporary world of work, although the opportunity to take decisions about one’s own work has directly impacted productivity (62), excessive decision authority resulting from the growing need for job flexibility can be seen as a source of stress. The growing burden of responsibilities and decisions may reach overload, especially when workers are not given the necessary resources (20, 61, 63). Also, high degrees of decision authority at work may lead to negative feelings resulting from the number of decisions to be taken and the uncertainties involved in making those choices (21, 64). However, more studies are needed to confirm these hypotheses, among other things to consider the possible influence of structural and contextual determinants on psychosocial aspects of work (65).
Also, and again contrary to the effects proposed by the theory of Karasek & Theorell (57) and found by other authors as regards different outcomes (20, 21, 59), we observed a positive association between skill discretion and SBP in the quantile model estimated for the total study population. However, we insist that it should be interpreted with great caution because it was significant in only one outer quantile of the distribution (quantile 10). On this point, it has been shown that, in quantile regression models, estimation for outer quantiles (lower or upper) is prone to major instability because there is generally considerable data dispersion at these extremes (66). The same care should be taken in interpreting the positive association observed between decision authority and DBP among antihypertensive users as the coefficients were significant for quantiles 5 and 10 only.
On the other hand, more consistent results were found in the quantile regression models for the association between decision authority and SBP for non-users of antihypertensives, where significant coefficients were observed from quantiles 35–60. Investigation of the relationship between different factors and blood pressure using quantile regression found a similar pattern for the association between stressful life-course events and SBP: higher coefficients were observed in the central portion of the distribution among participants who were non-users of antihypertensives (9). As with our findings, a study of Japanese workers found no overall association between job control and blood pressure, but significant results were observed among subjects with moderately high blood pressure (130≥SBP<160 mmHg and 85≥SBP<100) (67). That study, however, found an inverse association, but assessed job control as a single dimension. Another study also found no association between job strain quadrants and blood pressure for the overall study population, but it did identify significant results in a subgroup of previously normotensive subjects with high SBP in the workplace (68).
In this latter respect, Landsbergis et al (32) suggested that job strain first raises blood pressure levels in the workplace, without alterations being detected in casual blood pressure. Increased casual blood pressure is thought to occur only at later stages, when chronic exposure to these stressors has produced structural modifications to the cardiovascular system (36, 69, 70), particularly by remodeling minor arteries and arterioles which are the primary site of total peripheral resistance (71).
Accordingly, in our results, the small increase in SBP associated with high decision authority that was observed among those with blood pressure levels within normal limits (in the most central portion of the distribution) may reflect the presence of more pronounced alterations in blood pressure in the workplace. Future studies using ambulatory blood pressure monitoring will be able to provide additional evidence to help understand these findings.
Despite evidence of gender differences in the relationship between job strain and blood pressure (26, 33), this study did not identify any interaction between gender and the DCM components examined. It is suggested that this finding may be attributed partly to the specific characteristics of the ELSA-Brasil study population, which comprised civil servants, which may be confirmed by future studies using longitudinal cohort data. Meanwhile, the results of this study show that antihypertensives act as a considerable moderator of the relationship examined here, given that the more consistent associations were observed among non-users of these drugs. Antihypertensives act by reducing blood pressure artificially, while the blood pressure levels of individuals who use antihypertensives result from the combined action of a variety of factors, including proper prescribing by health professionals, the individuals’ adhering to drug therapy, modifications in lifestyle and the presence of comorbidities including obesity and diabetes (72–74). Accordingly, the interaction among all these factors may make the study of the relationship between blood pressure and its determinants more complex among users of antihypertensives.
As regards the analyses stratified by use of antihypertensives, we would like to emphasize that blood pressure levels were higher among users than non-users of these drugs, as can be seen in supplementary file 1. Non-users of antihypertensives are mostly normotensive subjects (about 90% in our study population; data not shown), and, accordingly, are expected to display lower SBP and DBP. Also, despite the high proportion of hypertensive participants with blood pressure control in the ELSA-Brasil study, around 30% of users of antihypertensives did not control their blood pressure (75).
Using DAG as a strategy to assist in selecting potential confounders of the relation studied was a strength of this study. Although constructed on the basis of a deduced causal structure between the variables examined, this approach does help to estimate less biased measures of effect (76). On this point, insufficient control for potential confounders has been indicated as one of the main sources of inconsistencies among studies of job strain and blood pressure (33). This study thus used a broad set of covariables and also considered mother’s schooling as a marker of early socioeconomic position, because it has been shown that the relationship between aspects of work and cardiovascular risk factors can be partly confounded by social disadvantages that originate in earlier stages of life (77). In our analyses, we observed that adjusting for potential confounders affected the gamma model coefficients considerably, generally resulting in loss of statistical significance. Notably, the direction of the association between the two job control components and blood pressure was observed to reverse after adjustment for the indicators of socioeconomic position. In our study population, the levels of DCM components, especially skill discretion and decision authority, were directly associated with socioeconomic position, while socioeconomic position associated inversely with blood pressure. Accordingly, socioeconomic position seems to act as a qualitative confounder in the association between the two job control components and blood pressure (78).
A strength of this study includes the use of data collected with extreme methodological rigor and relatively large sample size. Lastly, another strength of this study is its innovative analytical strategy, which combined independent assessment of the DCM components with statistical techniques suited to the nature of the data, yielded complementary evidence of the relationship studied here.
The limitations of the study include the cross-sectional nature of the analyses, which limits interpretations of the direction of the associations observed, while the possibility of reverse causality cannot be discarded. However, as the condition is asymptomatic, it is hardly plausible that increased blood pressure levels can modify the status of exposure to job strain. In studies such as ELSA-Brasil, volunteer recruitment is a potential source of selection bias, because participants and non-participants may differ systematically in relation to various characteristics (78). However, because of two characteristics of the ELSA-Brasil study, we believe it unlikely that our results were influenced by such bias. Firstly, recruitment quotas guaranteed demographic and socioeconomic variety in the study population. Secondly, the recruitment strategies used invited participation in a follow-up study, with no mention of any specific risk factor or disease. It is therefore unlikely for participants and non-participants to differ systematically as regards job strain and blood pressure, that is, that there be any distortion of exposure-outcome association.
The use of casual blood pressure measurement is another limitation, because as already mentioned it does not measure alterations in blood pressure at important points in the day, such as at work or during sleep, and measurements are liable to the “white coat effect”. Lastly, the study population displayed high levels of skill discretion and decision authority (medians of 11 and 6 points, respectively) and as a result, less heterogeneity in scores in these subscales. On this point, it has been pointed out that, in samples that are occupationally homogeneous (as with this study sample made up of civil servants), levels of the DCM dimensions are also more similar, which can lead to an underestimation of measures of association (33). It is thus possible that, as our sample was less heterogeneous in levels of skill discretion and decision authority, this may have led to underestimation of the association between these subscales and blood pressure.
In conclusion, our main findings showed that high decision authority and SBP are positively associated and that this association varies over the blood pressure distribution and by use of antihypertensives: significant coefficients were observed only in the central portion of the SBP distribution and only among non-users of antihypertensives. On the other hand, no consistent associations were found for decision authority in relation to DBP or for skill discretion in relation to either SBP or DBP. Moreover, no significant association was observed for job demands. The various analytical strategies applied in this study yield new evidence of the relationship between job strain and blood pressure. It is to be stressed that further studies are needed to evaluate the possibility of differential effects of the DCM components and to consider not only specific parts, but the whole continuum of blood pressure.