Working time patterns have been a public health issue for over a century. At first, the focus was on night/shift work, which was considered potentially harmful to health. In the 1920s, night work was banned for women in several European countries based on an early International Labour Organization (ILO) convention (1). In 2007, the International Agency of Research on Cancer classified shift work as “probably carcinogenic to humans” (Group 2A) (2), increasing the scientific debate of the possible risks of night shift work (3–6). Circadian disruption caused by working at night has been found to be associated with occupational accidents (7), cardiovascular diseases (8–10), and peptic ulcer (11). Shift work may also be related to type 2 diabetes (12), rheumatoid arthritis (13), multiple sclerosis (14), and psoriasis (15). Currently, the scientific debate of the possible health risks related to working time patterns covers the length of working hours and its social dimensions. Extensively long working hours appear to be a risk factor for coronary heart disease, type 2 diabetes, depression, sleep disturbances, and occupational and traffic safety (7, 16–18), and irregular and “unsocial” working hours have been associated with work–life imbalance, work stress, and mental disorders (19–21).
Epidemiological research on working hours and health has increased rapidly but the findings have been surprisingly inconsistent. Most previous studies have used questionnaire or interview-based data on working hours, which provide only crude information on the exposure to the multidimensional aspects of working hours and are additionally based on subjective reporting (3–6, 22–25). The specific measures used vary considerably between the studies, making exposure measurement an important source of bias also for systematic reviews (5). To date, very few studies have utilized daily registry data on working time patterns for exposure information. Lie et al’s (26) nested case–control study used national registers to categorize exposure to night work (information on night work was based on the Norwegian Board of Health’s registry of nurses and census data), but this study was based on assumptions that may not always hold, such as “work sites other than infirmaries only involved daytime work”, whereas “all work at infirmaries was assumed to include night work, except for managerial jobs, teaching, and work at physiotherapy or out-patients’ departments”. Some observational studies have used company records (27, 28) to categorize workers into day and shift workers. Taylor and Pocock et al (28), for example, defined shift workers as following a system of working hours other than regular day work (eg, 3-shift rotas at weekly or more frequent rotation, alternate day and shift work, double days, rotating 12-hour shift, regular night work). Further measures to characterize exposure to shift work include the use of job titles or a job exposure matrix (29). These methods have been criticized as being biased (30); in addition, information on job title or job exposure matrix cannot characterize multidimensional exposure.
In the National Longitudinal Survey of Youth (31), exposure to different work shifts based on information from both questionnaires and interviews did not match. Questionnaire measurement of the exposure to shift work may result in misclassifications that, if non-differential, reduce the estimated risk towards the null. Findings from the Million Women Study showed that the demographic characteristics of shift and day workers differed (32). This may lead to “differential” misclassification, which can bias results in either direction. Selection bias is possible, for example, if night workers were more difficult to reach by questionnaire or interview than day workers. Reverse causation bias will occur if women with breast cancer recall and report more accurately their lifetime exposure to night work than healthy women (5). A healthy worker effect and lower response rates in surveys of employees in “unsocial” working hours, in turn, can increase the risk for differential exposure misclassification in case-control studies (33).
To reduce various biases in epidemiological studies on working time and health, it is crucial to develop more accurate and reproducible exposure variables (30, 34–37). Such assessments would also increase opportunities to identify unhealthy components of working time patterns associated with long working hours and shift work. Accordingly, the aim of this study was to design a wide range of objective algorithms to measure and analyze working time patterns in epidemiologic studies that use register-based exposure assessment of working hours. The use of objective, register-based exposure assessment methods we develop in this study could be used to clarify the effects of shift work on chronic diseases in future studies. In addition, we validate a method to retrieve standard payroll data on working hours from the employer electronic records.
Methods
Participants
The individual-level data were collected from employers’ electronic working time records from six hospitals participating in the Finnish Public Sector study (38). Total data included 12 391 employees, ranging from 7836–8096 individuals per year and a total of 14 488 274 work shifts (table 1).
To evaluate the annual distributions of worktime characteristics, we limited the data to employees with a work contract of ≥10 months and ≥150 working days a year and excluded small groups not belonging to either nursing personnel or physicians (N=191 in 2013) based on their work contract data. The final analytical sample for comparisons between the study years included 7643 participants varying from 4808–4967 participants per year (tables 3–6). They covered 10 235 267 work shifts. The hospital districts gave a written permission to the Finnish Institute of Occupational Health to use the employers’ registry data on working hours for scientific research. All data were anonymized for research purposes. The ethics committee of the Hospital District of Helsinki and Uusimaa approved the Finnish Public Sector Study.
Table 3
Description of the working time variables among the final analytical sample including 4631 employees with a work contract of at least 10 months and with the minimum of 150 working days in 2013 [Md=median; Min=minimum; Max=maximum; SD=standard deviation]
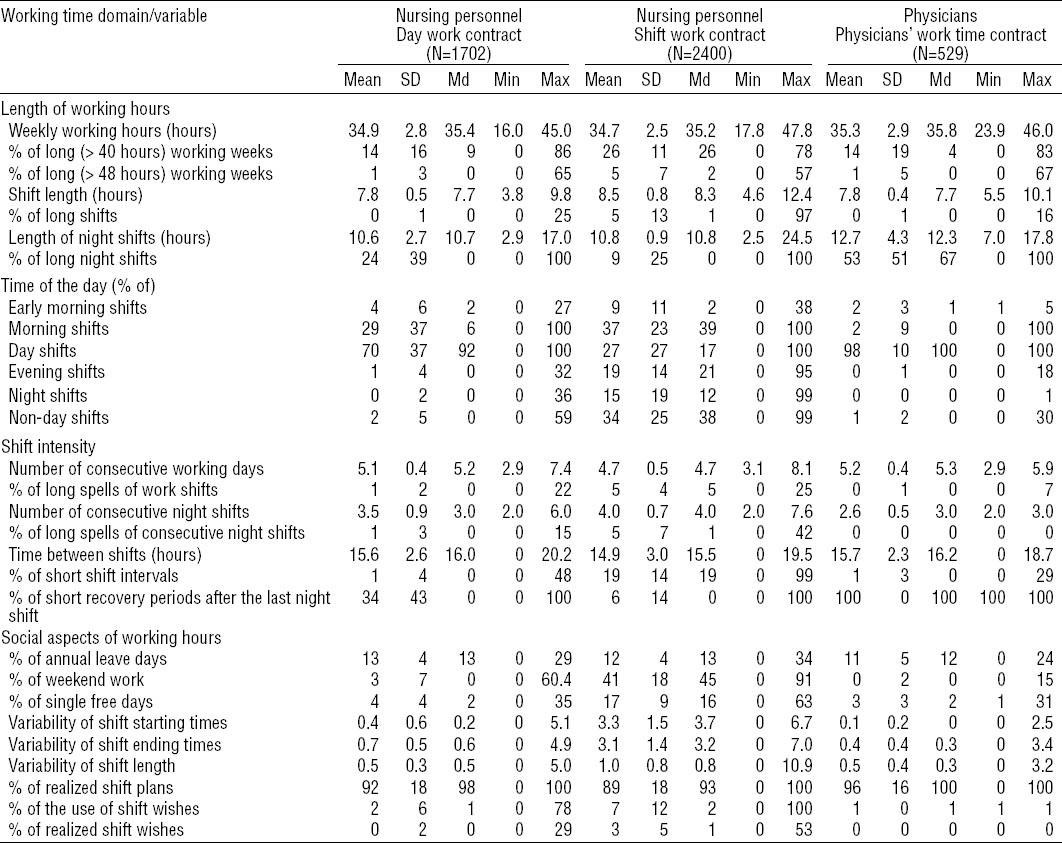
Table 4a
Between correlations of variables of annual working time patterns within each working time domain in 2013 for the final analytical sample limited to nursing personnel with a shift work contract of ≥10 months and with ≥150 working days in 2013, N=2400.
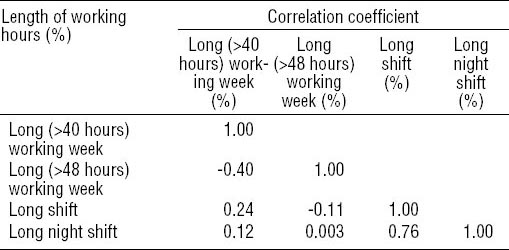
Table 4b
Between correlations of variables of annual working time patterns within each working time domain in 2013 for the final analytical sample limited to nursing personnel with a shift work contract of ≥10 months and with ≥150 working days in 2013, N=2400.
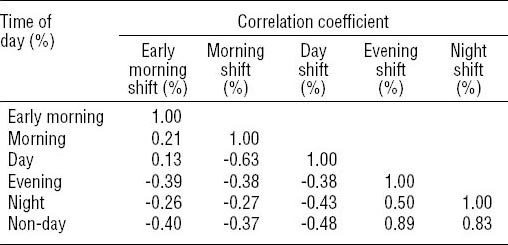
Table 4c
Between correlations of variables of annual working time patterns within each working time domain in 2013 for the final analytical sample limited to nursing personnel with a shift work contract of ≥10 months and with ≥50 working days in 2013, N=2400.
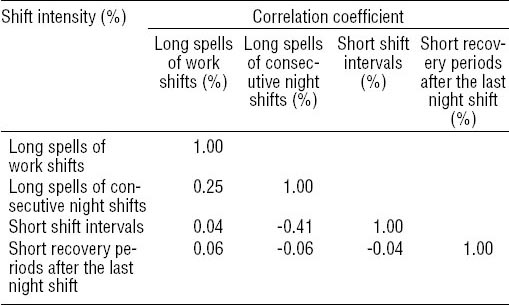
Table 4d
Between correlations of variables of annual working time patterns within each working time domain in 2013 for the final analytical sample limited to nursing personnel with a shift work contract of ≥10 months and with the ≥150 working days in 2013, N=2400.
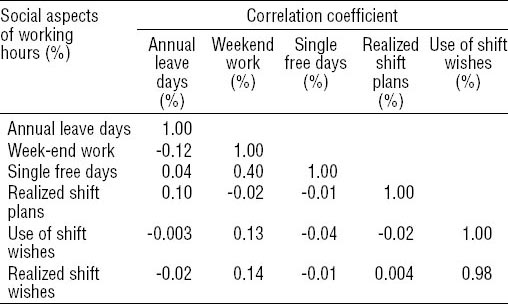
Working time data
All payroll-based daily working hour data were retrieved using the shift scheduling program Titania® from the beginning of 2008 to the end of 2013. The working time data were based on the shift plans (rotas) that were made with Titania® (CGI Finland) for every 3 or 6-week-period. Titania® is a Windows-compatible software which is used for shift planning and payroll in the majority of public sector organizations in Finland. It has tools for the planning of working hours according to national legislation and collective agreements with labor market organizations. The software calculates employee’s monthly reimbursements based on the materialized working hours and is linked to the electronic time card systems to correct the planned working hours by the individually punched true hours on minute-by-minute bases. The rotas are checked by the superiors of the organizations before acceptance for payroll and filed for ten years according to Finnish legislation
The sampling software (by CGI Finland) was used to retrieve all the data from the saved rotas. The resulted data included the starting and ending times of the daily working hours and the reasons for an absence (day off, sick leave, maternity leave, physician’s on-call duties, annual leave etc). Each individual work shift was also linked to numerous background information including the unique personal identification code ID (with information on age and sex), occupational title, working time contract/shift system, work unit, and shift rota unit.
To classify work shifts based on the shift starting and ending times, the sampling software scored the working hours into the following shift types: early morning shift (starts before 06:00 hours and is not categorized as a night shift); morning shift (starts 06:00–07:00 hours); day shift (starts after 07:00 hours and ends no later than 18:00 hours); evening shift (starts after 12:00 hours and is not categorized as a night shift); night shift (≥3 hours between 23:00–06:00 hours, according to the Finnish working time law).
Analysis of data quality
The process of data cleaning involved raw data analysis, definition, and verification of data transformation workflow, mapping rules, and the handling of possible errors (39). The quality of the retrieved total working hour data was examined by using the main lines of data taxonomy developed for time-oriented data (40). Based on the analysis we excluded all duplicates and missing data. The correctness of the data was additionally verified by comparing randomly selected consequent 3-week shift plans of one psychiatric inpatient department (with 15 employees), one acute inpatient department (with 22 employees), and one outpatient ward of home care (with 36 employees) to the data retrieved day-to-day and on individual basis. This assessment was done by comparing the scoring of work shifts obtained from the sampling software with the shifts based on the original raw data.
Dimensions and variables characterizing working time patterns
Based on the literature (see discussion for reasoning and justification), we constructed 29 annual variables of working time patterns (table 2). These variables belonged to four major working-hour domains: (i) the length of the working hours, including seven variables describing annual, weekly or daily working hours calculated for each year; (ii) time of the day (shift work), including six variables that were used to measure the proportion of different shifts; (iii) shift intensity, including seven variables related to both the consecutive work shifts and recovery time between the shifts; and (iv) social aspects of the working hours, including nine variables related to the distribution of free days, irregularity and predictability of the working hours, and worktime control.
Statistical analysis
Although the working-hour records are used for payroll purposes and should be precise, we examined whether the retrieved data and the created working time variables were correct and meaningful by calculating the distributions (mean, median, min and max) of these variables for each year in the final analytical sample. This helped to detect possible outliers and analyze the external validity of the data. Two statisticians calculated the working time variables from the original retrieved ASCII data using different statistical packages (Stata and SAS) to double-check the used formulas for calculating the variables. Based on the annual distributions, we investigated the prevalence of the working time variables in 2013 by calculating the proportion of those having (i) at least once a year, (ii) ≥10%, (iii) ≥25%, or (iv) ≥50% of the annual occurrence of the working time variables. The stability of the data between subsequent years was studied by calculating pairwise correlation coefficients of the repeated data on the working hour characteristics among those with ≥10 months of work contract and ≥150 working days during both subsequent years for all year-to-year combinations. We averaged the correlation coefficients for the combinations within 2-, 3-, 4- and 5-year periods. For example, the mean was calculated over the coefficients including year to year pairs for 4-year periods: 2008–2011, 2009–2012, and 2010–2013. Furthermore, we investigated the interrelationships between the working time variables by calculating the correlation coefficients for annual working time pattern variables (that were described in proportions) within the four working time domains.
Results
Quality control
In the original raw data from the 12 392 participants, including 14 488 274 work shifts in 2192 separate days from the time period 1 January 2008 to 31 December 2013, there were no missing values in shift starting or ending times. Only 189 participants had incomplete data and one had a missing ID code. There was a small amount of redundant duplicates (26 308 shifts, <0.01% of the shifts) due to the same employee being recorded twice in the same rota. The redundant duplicates were removed. A larger amount of shifts (1.8%) were incorrect duplicates having some differences in some of the variables for the same day of the same participant. The most frequent reasons for the wrong duplicates were (i) different rota number but otherwise identical shift data due to the employee being at the same time in two separate rotas; the second duplicate was removed; (ii) two separate shift starting and/or ending times very close to each other (eg, 08:00–16:00 and 08:00–16:15 or 08:00–16:15 and 08:15–16:15 hours). This error could occur due to after-shift corrections to the starting or ending times for salary corrections; the earlier starting and/or later ending times were kept and the shorter work shift was removed; (iii) having both a free day and a work shift overlapping with each other that could be because of last-minute cancelling of a free-day. In that case, the work shift was kept and the free day was removed. There were no outdated data (shifts outside the analyzed years).
The comparison of the six randomly selected 3-week shift plans from three different inwards showed a complete match between the original on-wall Excel rotas of the hospital departments and the retrieved Titania® registry data for shift starting and ending times, shift types, and absences from work.
Frequency and distributions of working time variables
The descriptive statistics of the created working time characteristics in 2013 are shown in table 3 according to the main occupational group and worktime contract. We found no unexpected distributions, based on our earlier knowledge and analysis of the working hours of the same or similar organizations (41, 42) and discussions with the persons responsible for the shift planning of the organizations. Among nursing personnel, the mean of the average weekly working hours during a year (when all calendar weeks with any work were included and both paid leave – eg, sickness absence – and unpaid leave were excluded) was 34.9 hours in day work and 34.7 hours in shift work among the nursing personnel. Among the physicians, the average weekly working hours was 35.3 hours, the maximum mean weekly working hours ranging from 45–48 hours. Few subjects, mostly physicians, had single calendar weeks with working hours of up to 92 hours (including some 24 hour shifts). There were considerable differences in the shift characteristics between the nursing personnel’s day work and shift work contracts and physicians’ working time contracts.
The interrelationships between different working time variables within each working time domain are shown in table 4. With few exceptions, the correlations were mostly low, indicating that the variables measured different aspects of working hours. High correlations were seen between % of long shifts and % of long night shifts (r=0.76) and the correlation between % of night shifts and % of non-day shifts (r=0.83).
Occurrence and prevalence
In order to decide the optimal algorithms for the exposure to potentially health-relevant aspects of working hour patterns, we calculated annual prevalence rates for different cut-off values related to the annual occurrence rates (at least once a year, and >10%, >25%, and >50% of the annual occurrence). As shown in table 5, depending on the occurrence, ie, the cut-off value chosen, the annual prevalence of the exposure variables among shift workers varied remarkably. For example, 93% of the subjects had at least one night shift in 2013, but the night shifts covered >25% of all the annual shifts only among 23% of the shift workers.
Stability and time trends of working time variables
Comparison between the years showed that working time variables within individuals were relatively stable from 2008–2013 (table 6), as shown by the mostly high year-to-year correlation coefficients ranging, from r=0.7–0.9 in 23 variables. The correlations decreased only moderately up to five years between the analyzed two years. The year-to-year correlation coefficients for rarely occurring variables (eg, % of early morning shifts, % of short recovery after the last night shifts) were lower, from r=0.4–0.6 showing lower stability.
Discussion
Epidemiological research on working hours and health has increased rapidly but the findings have been inconsistent. Transfer to electronic management systems, including the growing use of the electronic payroll and shift planning systems, has created new possibilities for access and analysis of exact exposure data on working hours in large epidemiological studies. The future possibilities to link objective exposure assessment of working time patterns to follow-up of morbidity and mortality will be a major step forward in the research of working hours and health. In this paper, we developed general algorithms for the assessment of 29 variables describing working time patterns to be used in future epidemiological studies on working hours, shift work and health.
These variables capture four major domains of working time patterns (length of the working hours, time of the day, shift intensity, and social aspects of working hours) which are based on the current understanding and evidence on the potential pathways of how working hours could influence physical and psychosocial health. The possible pathways from shift work to decreased health can be related to psychosocial, behavioral, or physiological mechanisms (2, 9). Since working hours are associated with different types of health outcomes and psychosocial problems, and we do not know yet which of the developed variables prove to be the most important ones, we suggest here a wide range of potential algorithms for the future studies. However, the selection of the exposure variables should be based on specific hypotheses and outcomes of the study and if this were not the case, the analyses should be corrected for multiple testing. All 29 working time pattern variables have been calculated for an annual time window due to the observed annual variation in the distribution of free-time and operational working hours linked to the many of used variables. The annual time window is also sufficiently long to allow a reliable estimation and follow-up of the used working time exposure variables.
The first domain, length of the working hours included algorithms for the annual, weekly or daily working hours calculated for each year separately. The selection of this domain and the variables designed can be justified by the fact that the length of the working hours needs to be related to the variation of cumulative work load if the work intensity itself is not modified. There is also good evidence of the association of long work shifts, long working weeks and long annual working hours with ill health, safety and work-home balance (7, 16, 17, 21, 43–45). The second domain, time of the day, includes the annual proportion of the different shifts; and the third domain, shift intensity includes several variables related to the number of consecutive work shifts and recovery time between the shifts. Studies have shown that night shift work is related to circadian dysrhythmia due to the changed exposure to environmental light and other circadian time cues (2, 37). Earlier studies have also indicated the effects of non-standard work shifts (especially night shifts and early morning shifts), and shift intensity (including factors like the speed and direction of shift rotation and recovery time between the shifts) on sleep and health (22, 45–48), Indeed, good conceptual (48) and epidemiological (49–51) support exists on the role of sufficient recovery in terms of sleep, health, and well-being. Besides the early morning and nights shifts, fixed evening work may also be associated with dissatisfaction with working hours and increased risk for sick leaves.
The last domain social aspects of working hours includes variables related to the distribution of free days, irregularity and predictability of the working hours and working time control. These aspects of working hours are closely related to the organization of work and the possibilities to influence free-time. Evidence also shows that fewer free weekends and more frequent single free days are associated with lower work satisfaction and subjective well-being (19, 52–54). As indicated by recent reviews, there are both theoretical and empirical reasons to assume that working time control would be an important factor for work-life balance, health and well-being (55, 56). In epidemiological studies, working time control has predicted subjective health, sickness absence, disability pensions and later retirement (57–59).
In addition to the use of average annual values of a working time variable (eg, average time between shifts during the whole year), we also suggest the use of dichotomized variables. The dichotomized variables have two components: the specified cut-off level and its frequency during the year. The cut-off levels (eg, long working hours being >48 hours a week) are mostly arbitrary and need to be re-defined when outcome data are available. There is some evidence on a few of the cut-offs suggesting, for example that >4 consecutive night shifts (weekly rotating shifts) are related to an increased health risk (21, 34–36). The suggested cut-off points of 40 and 48 hours for “long” and “very long” weekly working hours, 12 hours for “long” work shifts and the definition of 11 hours for “short” time between shifts are based on the existing European legislation and cut-offs used by many recent studies (51, 60–62). Based on the sensitivity analysis, we recommend the use of >25% occurrence when calculating prevalence for the individual variables. In relation to night shifts and quick returns, the use of 25% occurrence level means in practice >1 night shift or quick return in a week. This is more often than in the Nurses’s Health Study (24) where night shift work was defined as ≥3 night shifts in a month.
The use of objective exposure data would be a substantial improvement to the exposure assessment methods used in previous research on working hours and health. Most of the earlier studies have used questionnaires to assess exposure; this method is more prone for bias than objective register data. Many of the variables we suggest are similar to those used in earlier questionnaire studies. These variable are, for example, the weekly working hours, shift length, length of night shifts, number of consecutive working days and time between shifts. On the other hand, some of the variables are new since it is practically impossible to measure them in questionnaire studies (eg, the % of single free days or the % of realized shift wishes). Objective exposure data are naturally more precise than questionnaire data allowing determination of eg, the exact annual occurrence of night shifts (% of night shifts) for each year for all the subjects. Although it is possible that the “toxicity” of night work does not accumulate similarly as the toxicity of, for example, lead or other heavy metals, it is still the proportion of the night shifts, taking also into account the recovery aspect that is hypothesized to be important for the chronic health effects of shift work. To confirm this, further studies linking the different precisely measured exposure variable to health outcomes are needed.
The methodology used to retrieve and analyze the raw working hour data from employers’ registers proved to be valid. The retrieval of the data was easy, and the retrieved data included no wrong, missing, or outdated working hour data. The incorrect duplicate data were mostly due to the same employees attached to two different rotas having two separate rota codes but no or minimal differences in the actual working hours during a specific day. The duplicate data was easy to detect and delete or correct automatically. The created working time variables showed no unexpected distributions or significant outliers compared to previous smaller Finnish studies (41, 42). In rare cases, nurses who had a shift work contract did not have any night or evening shifts while some nurses with a day work contract were working during the nights. The results were logical based on the occasional delays in contract updating, as informed by the hospitals. The used working time variables were reasonably stable over the years, except for two variables with a very rare occurrence.
The use of an objective registry-based exposure assessment method does not fully substitute the use of questionnaire-based information on working hours since factors like the perceived control of working hours and the use of non-paid overtime work cannot be estimated from company records. Information on specific individual factors related to adaptation to night work, like chronotype, is also useful as such factors can modify the health effects of shift work (63). However, the registry data have several advantages, including continuous exposure information with no selection bias covering virtually all employees and no attrition (30, 36, 37). It also offers a possibility to analyze irregular, complex and changing working time patterns over long periods of time that are common in organizations, such as hospitals [eg, (64)]. Since the current knowledge on the effects of working time patterns on health is mostly based on crude methods on exposure assessment, it is likely that the use of the proposed more detailed register-based exposure information will create robust new knowledge on the association of working time patterns with health. Detailed exposure information is also essential in planning intervention studies to assess whether change in working time patterns could reduce morbidity or the other negative effects of shift work or unfavorable working hours.
To conclude, the data retrieval method used and the suggested algorithms allows a detailed characterization of working time patterns potentially relevant for health. For multidimensional exposure assessment, we suggest the measurement of four potentially health-relevant areas of working time patterns: the length of the working hours, time of the day, shift intensity, and the social aspects of the working hours. We propose that the developed method is considered as “a method for choice” to assess exposure to working time patterns in large-scale observational studies on working hours and health.